BRepGAT: Graph neural network to segment machining feature faces in a B-rep model
JOURNAL OF COMPUTATIONAL DESIGN AND ENGINEERING(2023)
摘要
In recent years, there have been many studies using artificial intelligence to recognize machining features in three-dimensional models in the computer-aided design (CAD)/computer-aided manufacturing field. Most of these studies converted the original CAD data into images, point clouds, or voxels for recognition. This led to information loss during the conversion process, resulting in decreased recognition accuracy. In this paper, we propose a novel deep learning model called the boundary representation graph attention network (BRepGAT) to segment faces in an original boundary representation (B-rep) model containing machining features. We define descriptors that represent information about the faces and edges of the B-rep model from the perspective of feature recognition. These descriptors are extracted from the B-rep model and transformed into homogeneous graph data, which are then passed to graph networks. BRepGAT recognizes machining features from the graph data input. Our experimental results using the MFCAD18++ dataset showed that BRepGAT achieved state-of-the-art recognition accuracy (99.1%). Furthermore, BRepGAT showed relatively robust performance on other datasets besides MFCAD18++. Graphical Abstract
更多查看译文
关键词
graph neural networks,deep learning,boundary representation,machining feature,face segmentation
AI 理解论文
溯源树
样例
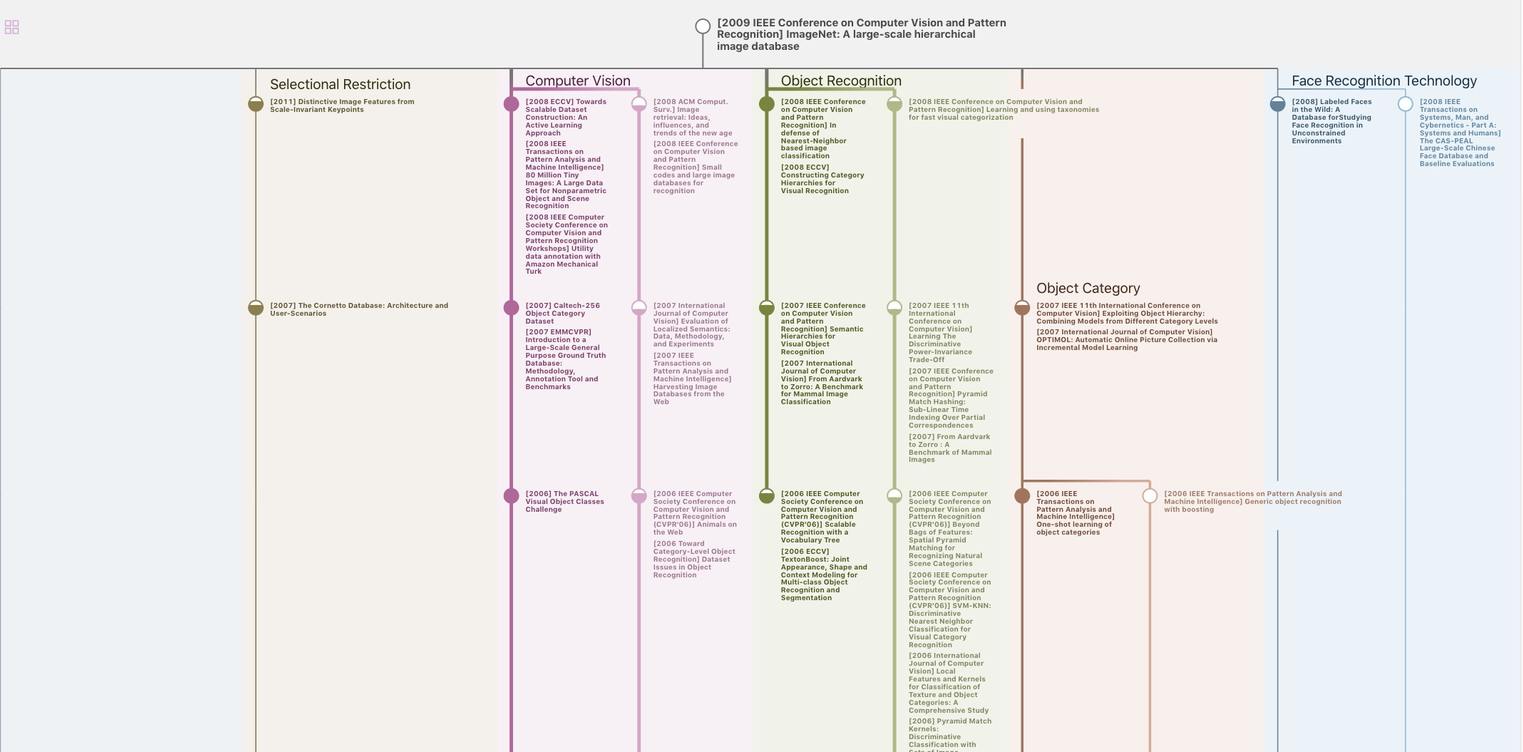
生成溯源树,研究论文发展脉络
Chat Paper
正在生成论文摘要