A Lightweight Fine-Grained Perception Defect Inspection Network Under Multitask Learning on Highly Reflective Surface
IEEE TRANSACTIONS ON INSTRUMENTATION AND MEASUREMENT(2024)
摘要
As an important mechanical transmission device, the camshafts with highly reflective surface (HRS) are widely used in various automobiles. The automobile manufacturers have increasingly strict requirements for the processing quality of camshafts. However, affected by multiple processing steps, it is impossible to achieve 100% defect-free products, which even tiny defects on their surfaces can significantly impact the performance, accuracy, and longevity of the camshaft. Therefore, precise inspection of camshaft defects in HRS becomes a necessary means of quality control, that reliable inspection methods still cannot meet the requirements. To address these issues, based on a multitask learning (MTL) framework, a lightweight fine-grained perceptual defect inspection network is proposed that is able to optimize both the saliency detection and classification tasks. The network uses a reparameterization technique and a lightweight bottleneck layer that can encode and fuse multiscale features efficiently, while a fine-grained feature-aware module combined with spatial attention and channel attention mechanisms effectively improved the classification accuracy. Based on the experimental validation, the proposed method can achieve a high Mathews correlation coefficient (MCC) of 0.951 and real-time speed of 190 fps on a single GPU, meanwhile demonstrate excellent performance and interpretability in HRS defect inspection and classification.
更多查看译文
关键词
Annotations,Graphics processing units,Inspection,Real-time systems,Task analysis,Camshafts,Surface treatment,Attention mechanisms,fine-grained perception,highly reflective surface (HRS),multitask learning (MTL)
AI 理解论文
溯源树
样例
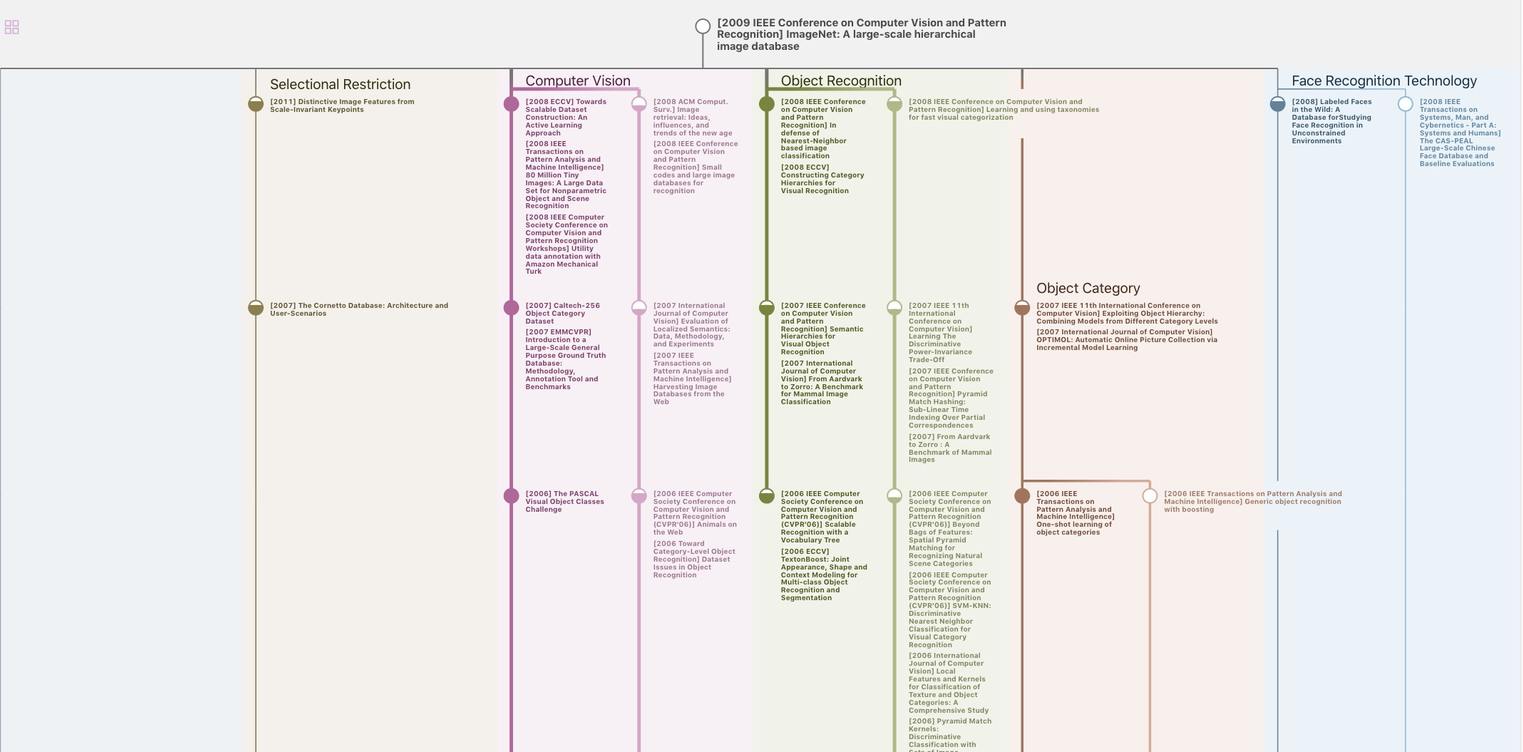
生成溯源树,研究论文发展脉络
Chat Paper
正在生成论文摘要