Frameworks for Privacy-Preserving Federated Learning
IEICE TRANSACTIONS ON INFORMATION AND SYSTEMS(2024)
摘要
In this paper, we explore privacy-preserving techniques in federated learning, including those can be used with both neural networks and decision trees. We begin by identifying how information can be leaked in federated learning, after which we present methods to address this issue by introducing two privacy-preserving frameworks that encompass many existing privacy-preserving federated learning (PPFL) systems. Through experiments with publicly available financial, medical, and Internet of Things datasets, we demonstrate the effectiveness of privacy-preserving federated learning and its potential to develop highly accurate, secure, and privacy-preserving machine learning systems in real-world scenarios. The findings highlight the importance of considering privacy in the design and implementation of federated learning systems and suggest that privacypreserving techniques are essential in enabling the development of effective and practical machine learning systems.
更多查看译文
关键词
Federated learning,privacy preservation,neural network,decision tree
AI 理解论文
溯源树
样例
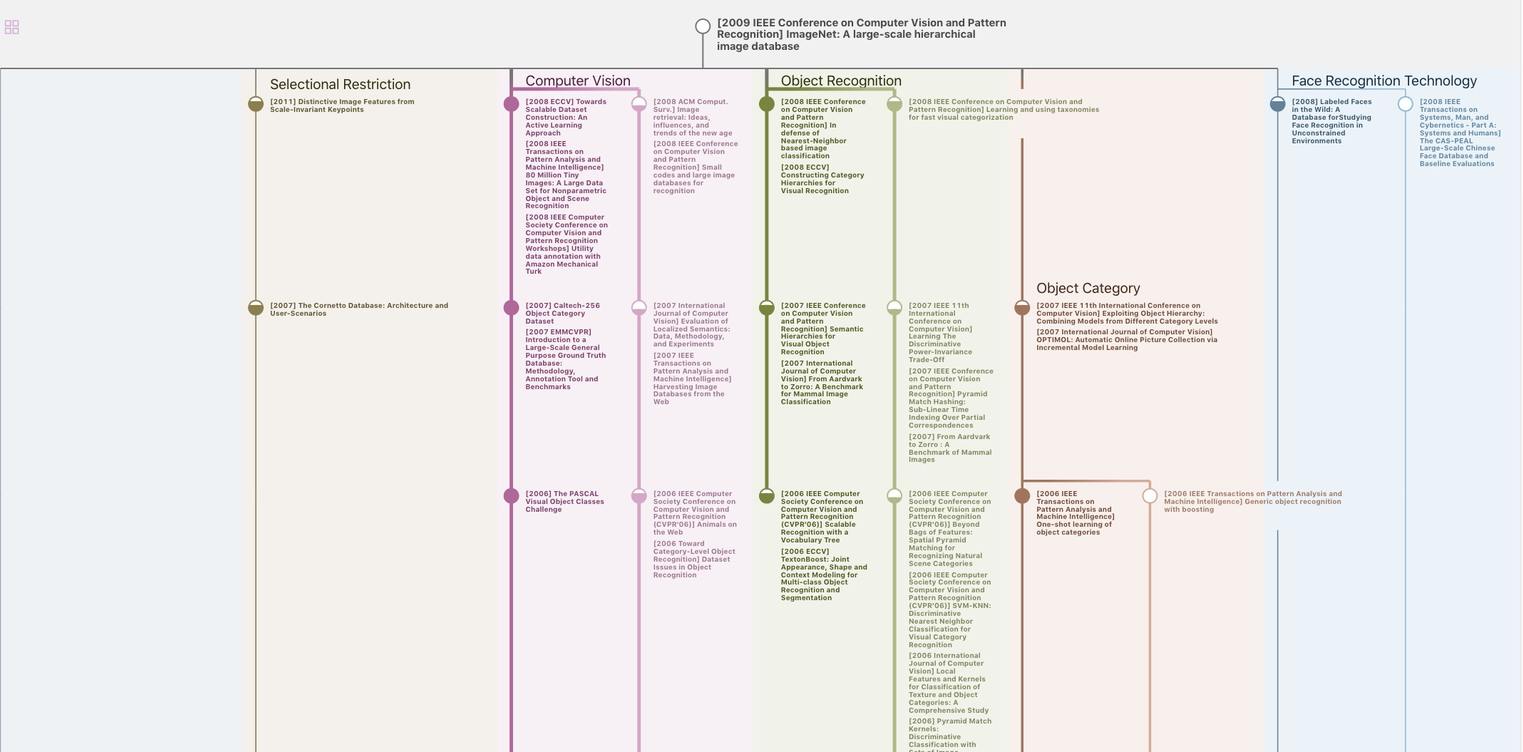
生成溯源树,研究论文发展脉络
Chat Paper
正在生成论文摘要