Metrics for Assessing Generalization of Deep Reinforcement Learning in Parameterized Environments
JOURNAL OF ARTIFICIAL INTELLIGENCE AND SOFT COMPUTING RESEARCH(2023)
摘要
In this work, a study focusing on proposing generalization metrics for Deep Reinforcement Learning (DRL) algorithms was performed. The experiments were conducted in DeepMind Control (DMC) benchmark suite with parameterized environments. The performance of three DRL algorithms in selected ten tasks from the DMC suite has been analysed with existing generalization gap formalism and the proposed ratio and decibel metrics. The results were presented with the proposed methods: average transfer metric and plot for environment normal distribution. These efforts allowed to highlight major changes in the model's performance and add more insights about making decisions regarding models' requirements.
更多查看译文
关键词
deep reinforcement learning,optimization,generalization,Sim2Sim transfer,adaptation
AI 理解论文
溯源树
样例
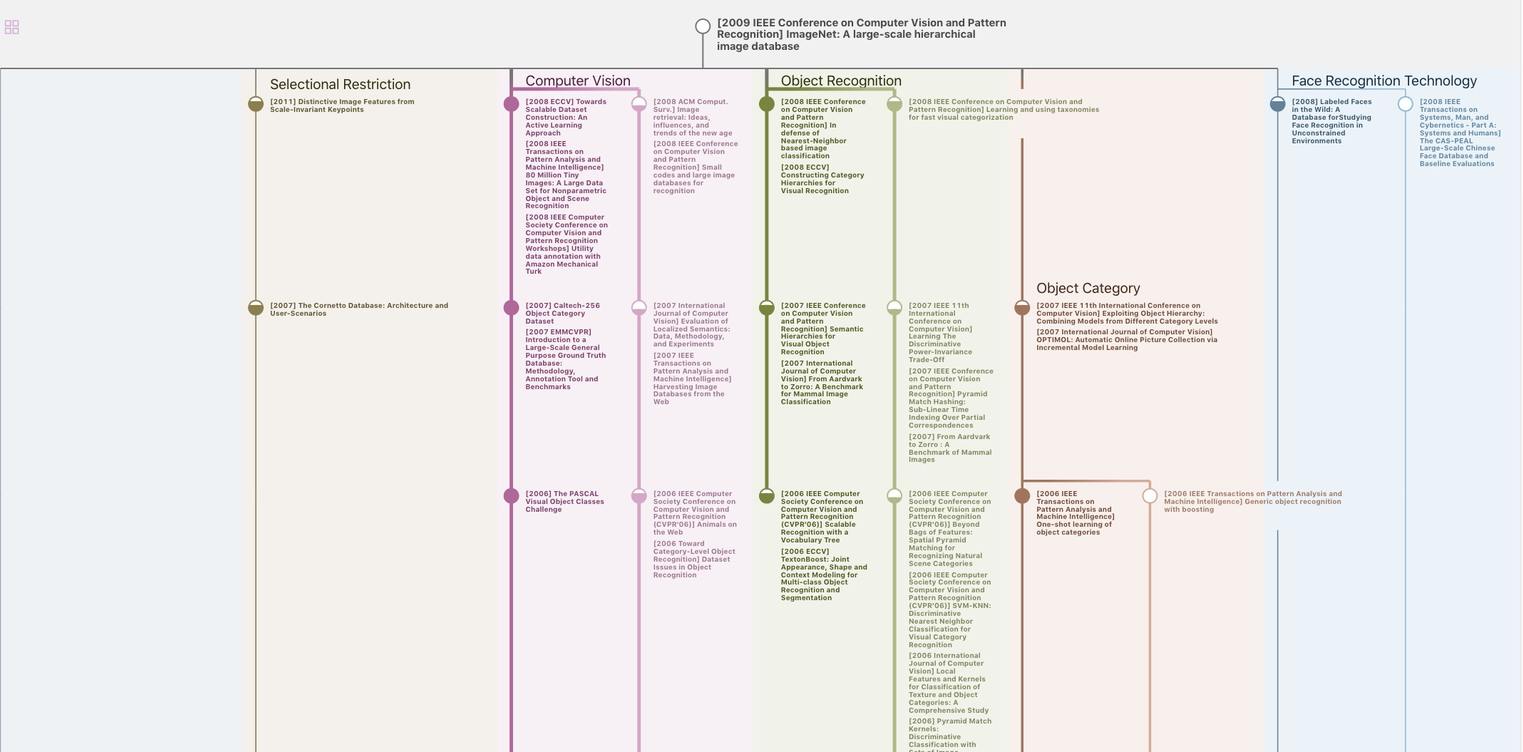
生成溯源树,研究论文发展脉络
Chat Paper
正在生成论文摘要