Ecarnet: enhanced clue-ambiguity reasoning network for multimodal fake news detection
Multimedia Systems(2024)
摘要
The growing popularity of social media platforms has simplified the creation of news articles, and fake news being spread on these platforms have a disruptive impact on our lives. With the prevalence of multimodal data in social networks, automatic detection of multimodal fake news plays an important role in the prevention of its negative effects. To the best of our knowledge, existing multimodal fake news detection works still face two challenging roadblocks: the lack of ambiguous clue reasoning across different modalities and insufficient fusion of multimodal information. To alleviate these concerns, this paper presents an Enhanced Clue-Ambiguity Reasoning Network (ECARnet) for multimodal fake news detection. The proposed model first extracts the fine-grained semantic features from salient image regions and semantic textual words, and further utilizes a cross-modal weighted residual network to learn their similar clue features. Subsequently, an efficient bidirectional clue-ambiguity reasoning module is constructed to explicitly excavate the ambiguous clue features. Specifically, forward reasoning branch employs the two-branch network with clue correlation analysis to maximally distinguish the cross-modal ambiguous clues, while backward reasoning branch utilizes uncertainty learning to eliminate the uncertain clue features. Through the joint exploitation of the above, the proposed ECARnet model can adaptively learn the cross-modal ambiguous clues to benefit various multimodal fake news detection tasks. Extensive experiments verify the superiority of the proposed framework and show its competitive performances with the state-of-the-arts.
更多查看译文
关键词
Multimodal fake news detection,Enhanced clue-ambiguity reasoning,Forward reasoning,Backward reasoning
AI 理解论文
溯源树
样例
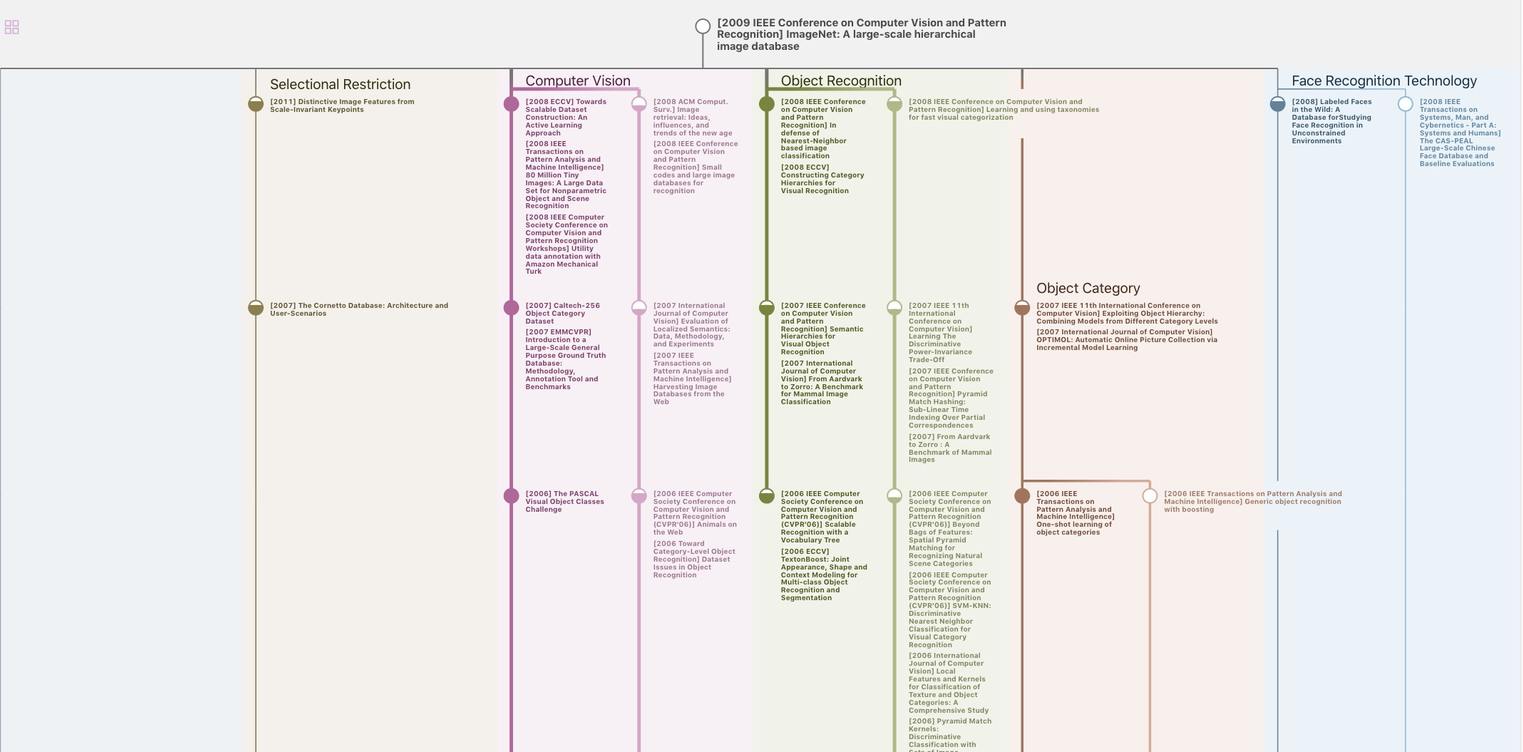
生成溯源树,研究论文发展脉络
Chat Paper
正在生成论文摘要