Comparing Spectral Bias and Robustness For Two-Layer Neural Networks: SGD vs Adaptive Random Fourier Features
CoRR(2024)
摘要
We present experimental results highlighting two key differences resulting
from the choice of training algorithm for two-layer neural networks. The
spectral bias of neural networks is well known, while the spectral bias
dependence on the choice of training algorithm is less studied. Our experiments
demonstrate that an adaptive random Fourier features algorithm (ARFF) can yield
a spectral bias closer to zero compared to the stochastic gradient descent
optimizer (SGD). Additionally, we train two identically structured classifiers,
employing SGD and ARFF, to the same accuracy levels and empirically assess
their robustness against adversarial noise attacks.
更多查看译文
AI 理解论文
溯源树
样例
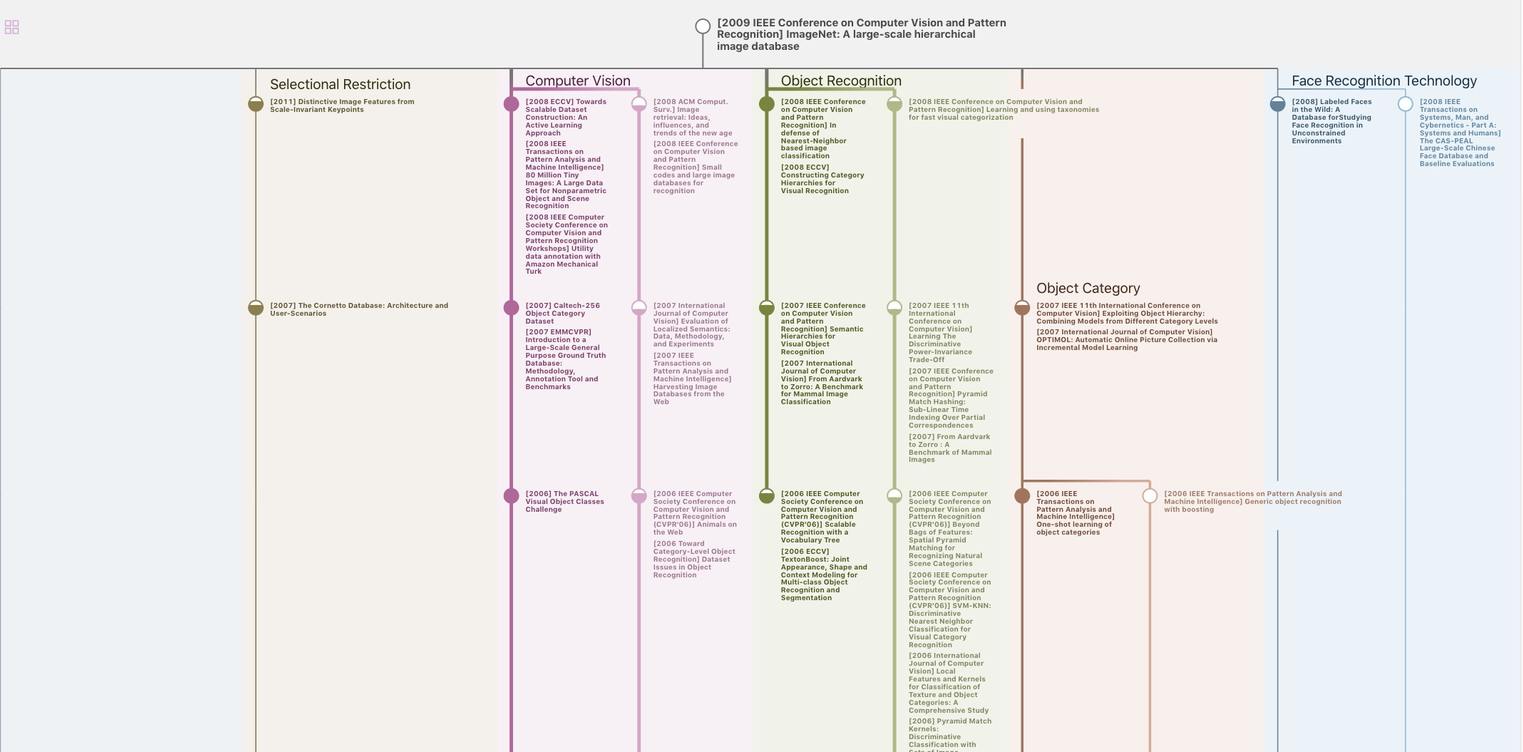
生成溯源树,研究论文发展脉络
Chat Paper
正在生成论文摘要