Does DetectGPT Fully Utilize Perturbation? Bridge Selective Perturbation to Fine-tuned Contrastive Learning Detector would be Better
arxiv(2024)
摘要
The burgeoning generative capabilities of large language models (LLMs) have
raised growing concerns about abuse, demanding automatic machine-generated text
detectors. DetectGPT, a zero-shot metric-based detector, first introduces
perturbation and shows great performance improvement. However, in DetectGPT,
random perturbation strategy could introduce noise, and logit regression
depends on threshold, harming the generalizability and applicability of
individual or small-batch inputs. Hence, we propose a novel fine-tuned
detector, Pecola, bridging metric-based and fine-tuned detectors by contrastive
learning on selective perturbation. Selective strategy retains important tokens
during perturbation and weights for multi-pair contrastive learning. The
experiments show that Pecola outperforms the state-of-the-art by 1.20
accuracy on average on four public datasets. And we further analyze the
effectiveness, robustness, and generalization of the method.
更多查看译文
AI 理解论文
溯源树
样例
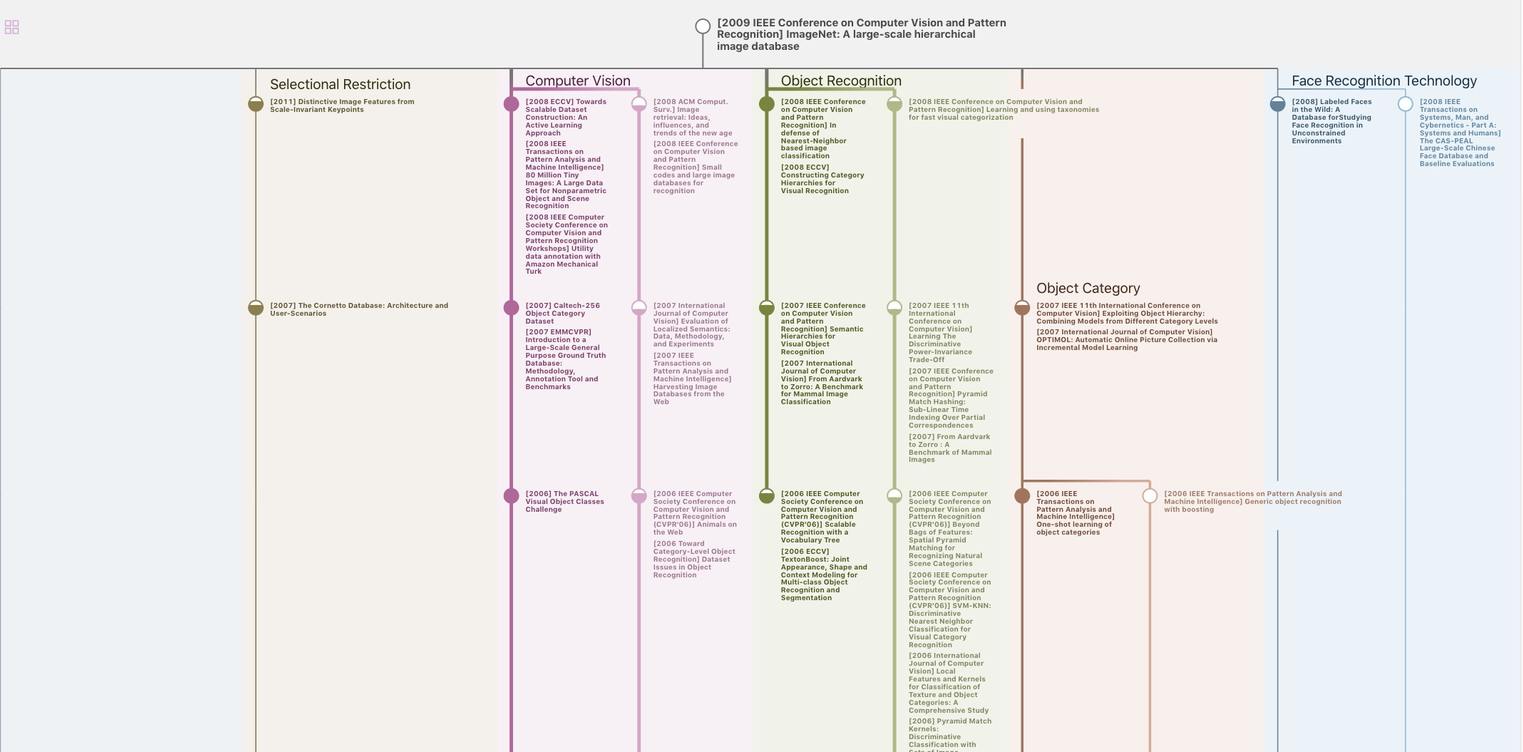
生成溯源树,研究论文发展脉络
Chat Paper
正在生成论文摘要