On the O(√(d)/T^1/4) Convergence Rate of RMSProp and Its Momentum Extension Measured by ℓ_1 Norm
arxiv(2024)
摘要
Although adaptive gradient methods have been extensively used in deep
learning, their convergence rates proved in the literature are all slower than
that of SGD, particularly with respect to their dependence on the dimension.
This paper considers the classical RMSProp and its momentum extension and
establishes the convergence rate of 1/T∑_k=1^T E[∇
f(x^k)_1]≤ O(√(d)C/T^1/4) measured by ℓ_1 norm
without the bounded gradient assumption, where d is the dimension of the
optimization variable, T is the iteration number, and C is a constant
identical to that appeared in the optimal convergence rate of SGD. Our
convergence rate matches the lower bound with respect to all the coefficients
except the dimension d. Since x_2≪x_1≤√(d)x_2 for
problems with extremely large d, our convergence rate can be considered to be
analogous to the 1/T∑_k=1^T E[∇ f(x^k)_2]≤
O(C/T^1/4) rate of SGD in the ideal case of ∇
f(x)_1=(√(d)∇ f(x)_2).
更多查看译文
AI 理解论文
溯源树
样例
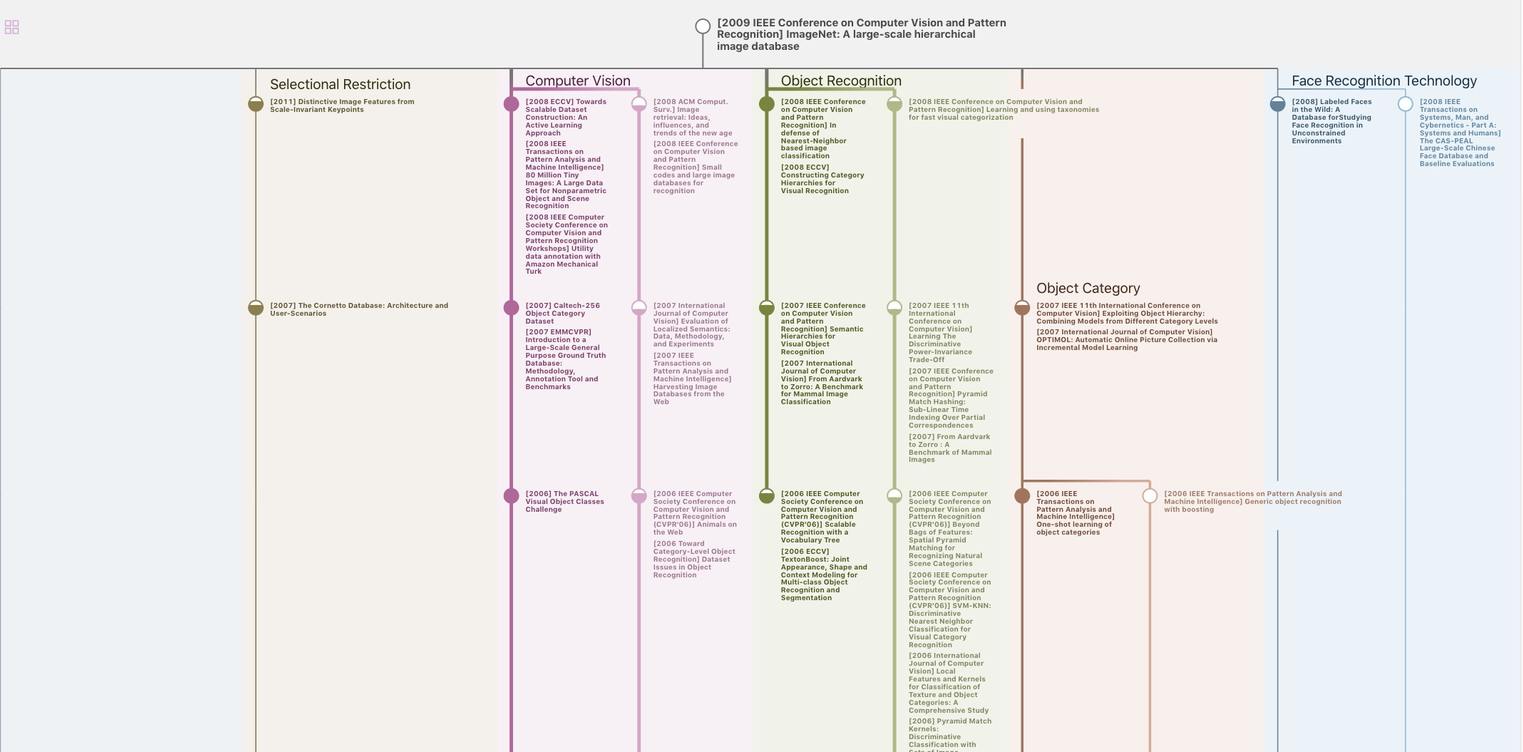
生成溯源树,研究论文发展脉络
Chat Paper
正在生成论文摘要