DiffMDD: A Diffusion-Based Deep Learning Framework for MDD Diagnosis Using EEG
IEEE TRANSACTIONS ON NEURAL SYSTEMS AND REHABILITATION ENGINEERING(2024)
摘要
Major Depression Disorder (MDD) is a common yet destructive mental disorder that affects millions of people worldwide. Making early and accurate diagnosis of it is very meaningful. Recently, EEG, a non-invasive technique of recording spontaneous electrical activity of brains, has been widely used for MDD diagnosis. However, there are still some challenges in data quality and data size of EEG: (1) A large amount of noise is inevitable during EEG collection, making it difficult to extract discriminative features from raw EEG; (2) It is difficult to recruit a large number of subjects to collect sufficient and diverse data for model training. Both of the challenges cause the overfitting problem, especially for deep learning methods. In this paper, we propose DiffMDD, a diffusion-based deep learning framework for MDD diagnosis using EEG. Specifically, we extract more noise-irrelevant features to improve the model's robustness by designing the Forward Diffusion Noisy Training Module. Then we increase the size and diversity of data to help the model learn more generalized features by designing the Reverse Diffusion Data Augmentation Module. Finally, we re-train the classifier on the augmented dataset for MDD diagnosis. We conducted comprehensive experiments to test the overall performance and each module's effectiveness. The framework was validated on two public MDD diagnosis datasets, achieving the state-of-the-art performance.
更多查看译文
关键词
Major depression disorder,electroencephalogram,mental disorder diagnosis,deep learning
AI 理解论文
溯源树
样例
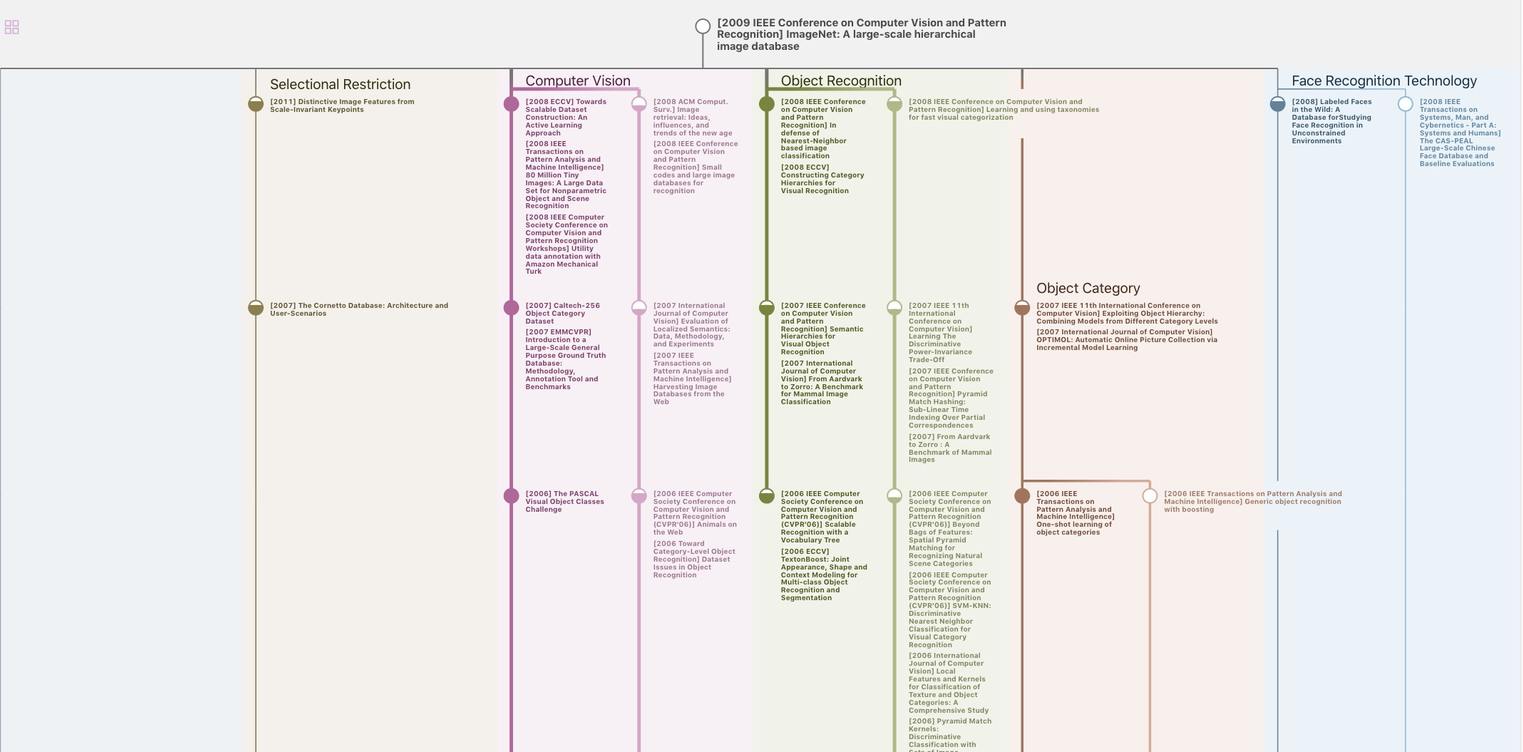
生成溯源树,研究论文发展脉络
Chat Paper
正在生成论文摘要