Prediction-CGAN: Human Action Prediction with Conditional Generative Adversarial Networks
Proceedings of the 27th ACM International Conference on Multimedia(2019)
摘要
The underlying challenge of human action prediction, i.e. maintaining prediction accuracy at very beginning of an action execution, is still not well handled. In this paper, we propose a Prediction Conditional Generative Adversarial Network (Prediction-CGAN) for predicting action, which shares information between completely observed and partially observed videos. Instead of generating future frames, we aim at completing visual representations of unfinished video, which can be directly utilized to predict action label no matter at any progress levels. The Prediction-CGAN incorporates the completion constraint to learn a transformation from incomplete actions to complete actions; the adversarial constraint to ensure the generation has similar discriminative power to complete representation; the label consistency constraint to encourage label consistency between each segment and its corresponding complete video; and the confidence monotonically increasing constraint to yield increasingly accurate predictions as observing more frames. Meanwhile, we introduce a novel adversarial criterion especially for prediction task, which requires the generation is more discriminative than its corresponding incomplete representation, while the generation is less discriminative than its real complete representation. In experiments, we present adequate evaluations to show that the proposed Prediction-CGAN outperforms state-of-the-art methods in action prediction.
更多查看译文
关键词
action prediction, completion transformation, conditional generative adversarial networks, label consistency preservation, temporal evolution preservation
AI 理解论文
溯源树
样例
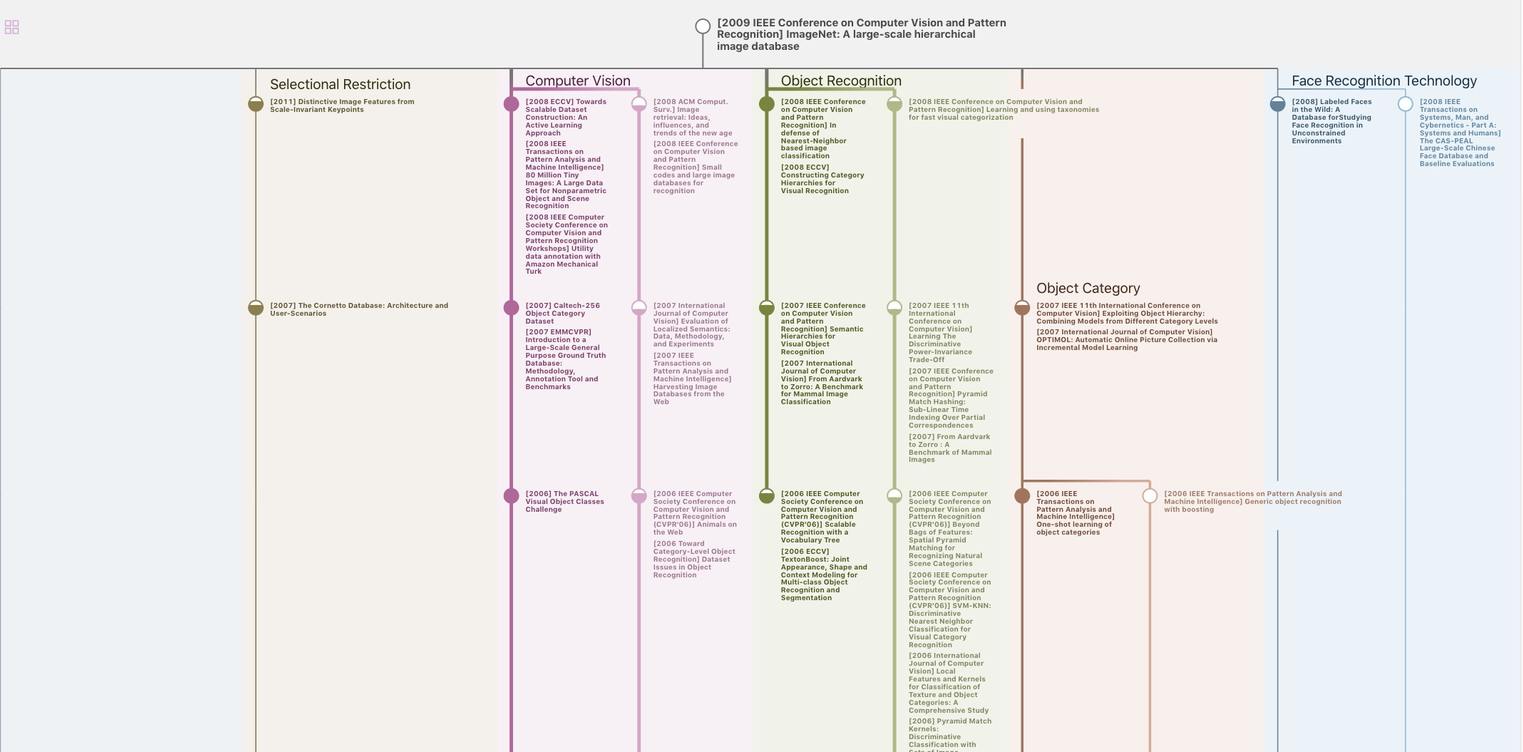
生成溯源树,研究论文发展脉络
Chat Paper
正在生成论文摘要