Fast Approximation to Discrete-Event Simulation of Markovian Queueing Networks.
Winter Simulation Conference(2023)
摘要
Simulation of queueing networks is generally carried out by discrete-event simulation (DES), in which the simulation time is driven by the occurrence of the next event. However, for large-scale queueing networks, especially when the network is very busy, keeping track of all events is computationally inefficient. Moreover, as the traditional DES is inherently sequential, it is difficult to harness the capability of parallel computing. In this paper, we propose a parallel fast simulation approximation framework for large-scale Markovian queueing networks, where the simulation horizon is discretized into small time intervals and the system state is updated according to the events happening in each time interval. The computational complexity analysis demonstrates that our method is more efficient for large-scale networks compared with traditional DES. We also show its relative error converges to zero. The experimental results show that our framework can be much faster than the state-of-the-art DES tools.
更多查看译文
关键词
Fast Estimation,Queueing System,Discrete Event Simulation,Time Interval,Computational Complexity,Parallelization,Large-scale Networks,Parallel Simulator,Computational Complexity Analysis,Estimation Error,Lower Bound,Error Analysis,Estimation Algorithm,Exponential Distribution,Multi-core,Conservation Strategies,Time Of Occurrence,Multilayer Network,Poisson Process,Service Time,Queue Length,Beginning Of Interval,Number Of Processors,List Of Events,Real-world Networks,Simulation Speed,Upper Bound Estimate,List Length,Regular Network,Service Rate
AI 理解论文
溯源树
样例
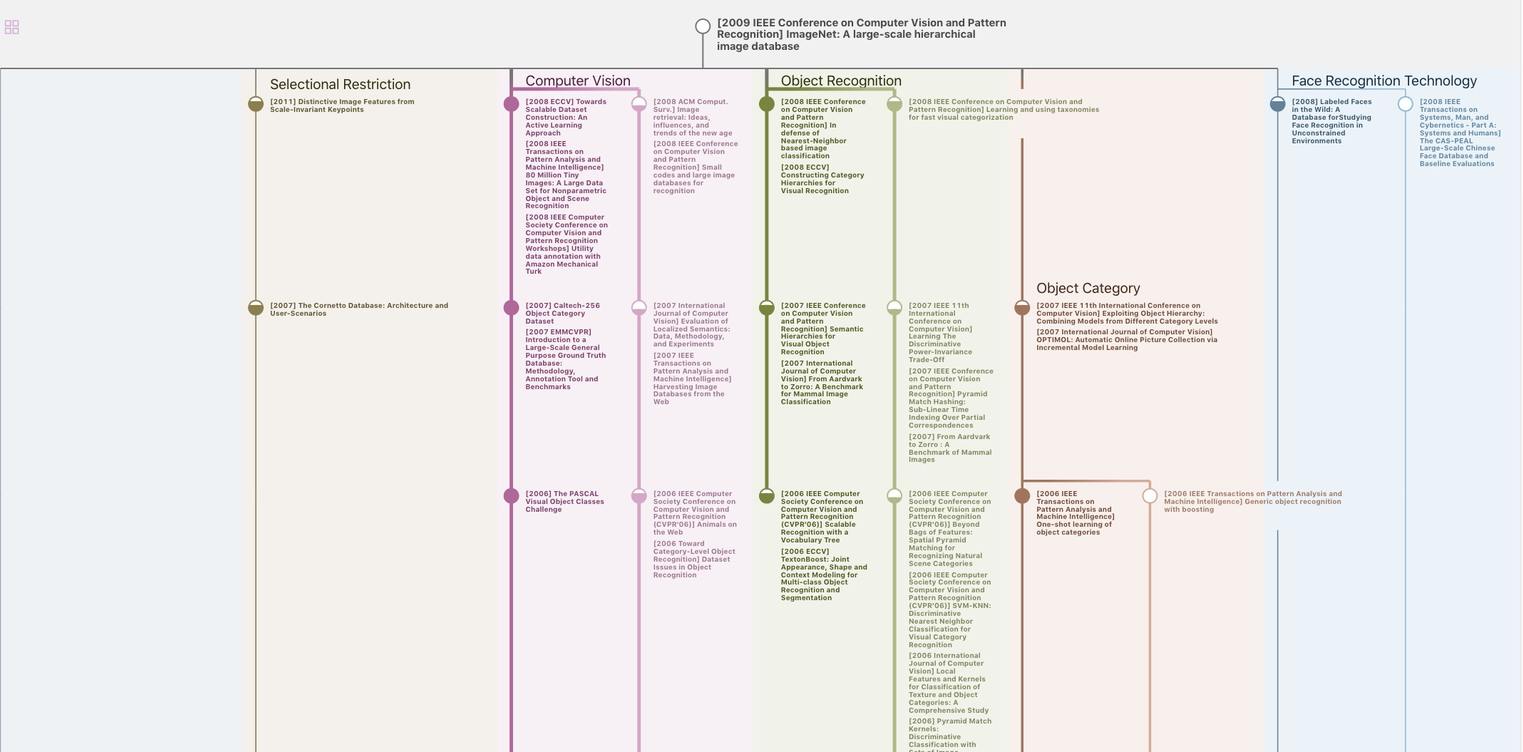
生成溯源树,研究论文发展脉络
Chat Paper
正在生成论文摘要