Hardware-Friendly Block Variable-Length Sampling Pruning for Graph Neural Networks.
Midwest Symposium on Circuits and Systems(2023)
摘要
Graph Neural Networks (GNNs) have achieved success in graph-based applications. Despite the proposed technologies are useful to speed up GNNs, the existing pruning methods still require extra costs and are hardly able to improve the hardware efficiency. Here, we propose a Block Variable-length Sampling Pruning (BVSP) method to balance the hardware workload, reduce the redundancy of graph structure, alleviate data sparsity, and improve the efficiency of hardware acceleration. Two sampling strategies for different scenarios are proposed. We evaluate our method on datasets and models, and it is verified that our method can achieve a pruning ratio of 10%to 50% and reduce the hardware scheduling workloads by 60% to 99% without any accuracy loss and extra costs.
更多查看译文
关键词
Graph Neural Networks,Graph Pruning,Variable-length,Workload
AI 理解论文
溯源树
样例
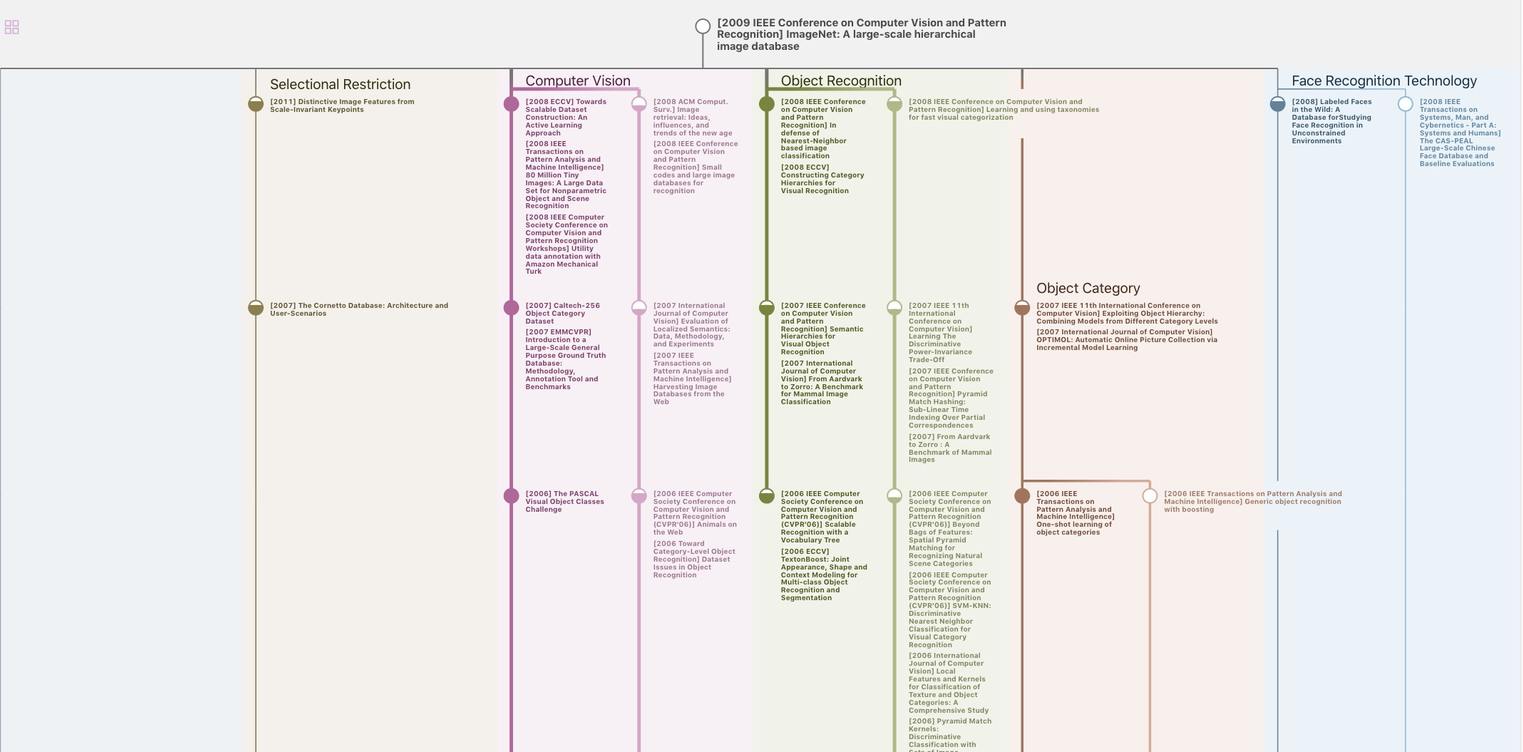
生成溯源树,研究论文发展脉络
Chat Paper
正在生成论文摘要