AutoHLS: Learning to Accelerate Design Space Exploration for HLS Designs
Midwest Symposium on Circuits and Systems(2024)
摘要
High-level synthesis (HLS) is a design flow that leverages modern language
features and flexibility, such as complex data structures, inheritance,
templates, etc., to prototype hardware designs rapidly. However, exploring
various design space parameters can take much time and effort for hardware
engineers to meet specific design specifications. This paper proposes a novel
framework called AutoHLS, which integrates a deep neural network (DNN) with
Bayesian optimization (BO) to accelerate HLS hardware design optimization. Our
tool focuses on HLS pragma exploration and operation transformation. It
utilizes integrated DNNs to predict synthesizability within a given FPGA
resource budget. We also investigate the potential of emerging quantum neural
networks (QNNs) instead of classical DNNs for the AutoHLS pipeline. Our
experimental results demonstrate up to a 70-fold speedup in exploration time.
更多查看译文
关键词
HLS acceleration,design space exploration,optimization,design automation,FPGA
AI 理解论文
溯源树
样例
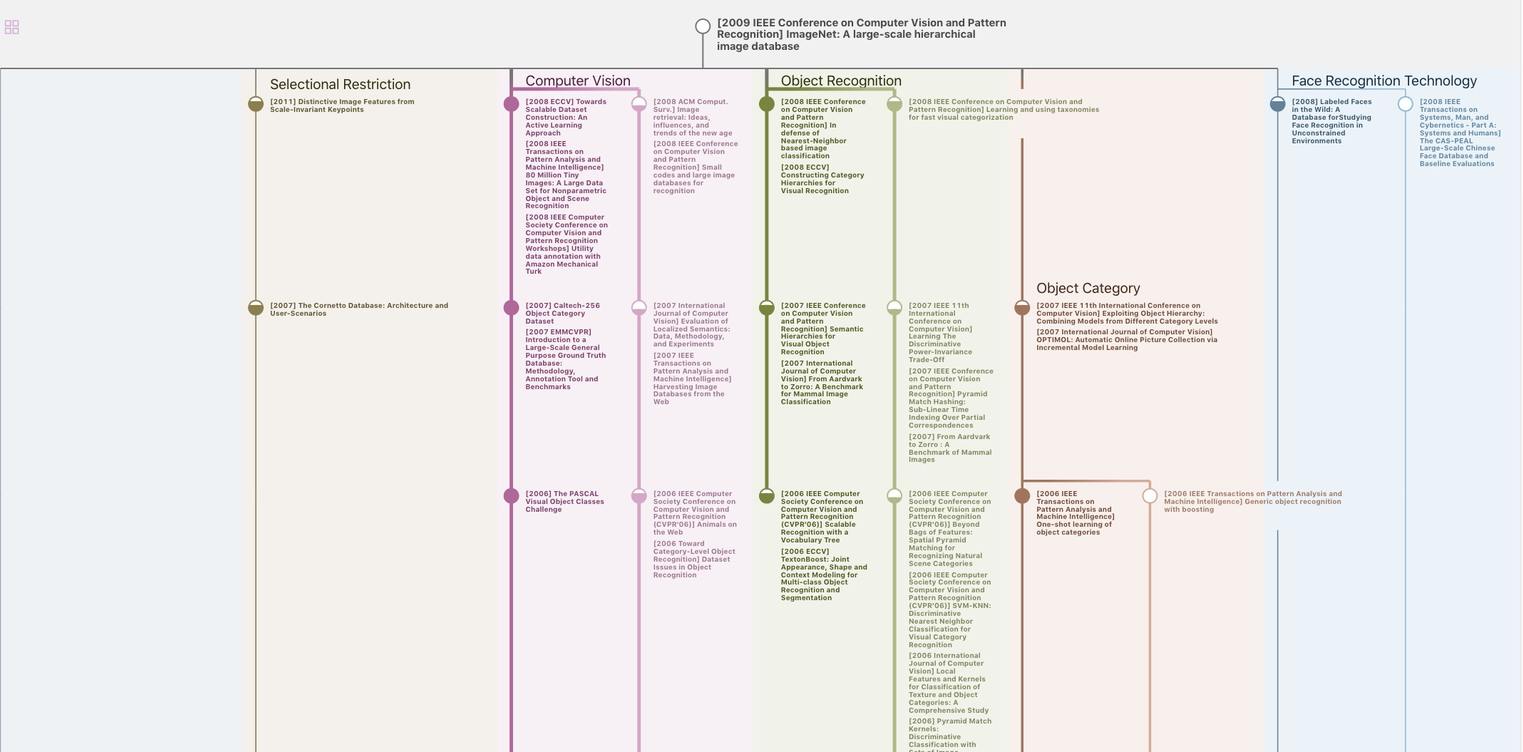
生成溯源树,研究论文发展脉络
Chat Paper
正在生成论文摘要