Modeling Multivariate Relations in Multiblock Semiconductor Manufacturing Data Using Process PLS To Enhance Process Understanding.
Winter Simulation Conference(2023)
摘要
The complexity of manufacturing process data has made it more challenging to extract useful insights. Data-analytic solutions have therefore become essential for analyzing and optimizing manufacturing processes. Path modeling, also known as structural equation modeling, is a statistical approach that can provide new insights into complex multivariate relationships between process variables from different stages of the manufacturing process. The incorporation of expert process knowledge and subsequent interpretation of model results can facilitate communication between stakeholders, promoting lean manufacturing and achieving the sustainability goals of Industry 5.0. This paper describes the use of a path modeling algorithm called Process Partial Least Squares (Process PLS) to gain new insights into the relationships between equipment data from several machines within the semiconductor manufacturing process. The methods used in this study can assist manufacturers in understanding the relations between different machines and identify the most influential variables that may be used to develop soft-sensors.
更多查看译文
关键词
Semiconductor Industry,Structural Equation Modeling,Manufacturing Process,Partial Least Squares,Path Model,Facilitate Communication,Partial Least,Incorporation Of Knowledge,Time Series,Part Of Process,Proportion Of Variance,R2 Values,Remainder Of This Paper,Latent Variables,Model Specification,Research Goals,Partial Data,Partial Least Squares Regression,Time Series Variables,Data Block,Inner Model,Outer Model,Target Block,Values Of P2,Data Analytics Applications,Manufacturing Steps,Partial Least Squares Model,Multiple Blocks,Optimal Prediction,Learning Algorithms
AI 理解论文
溯源树
样例
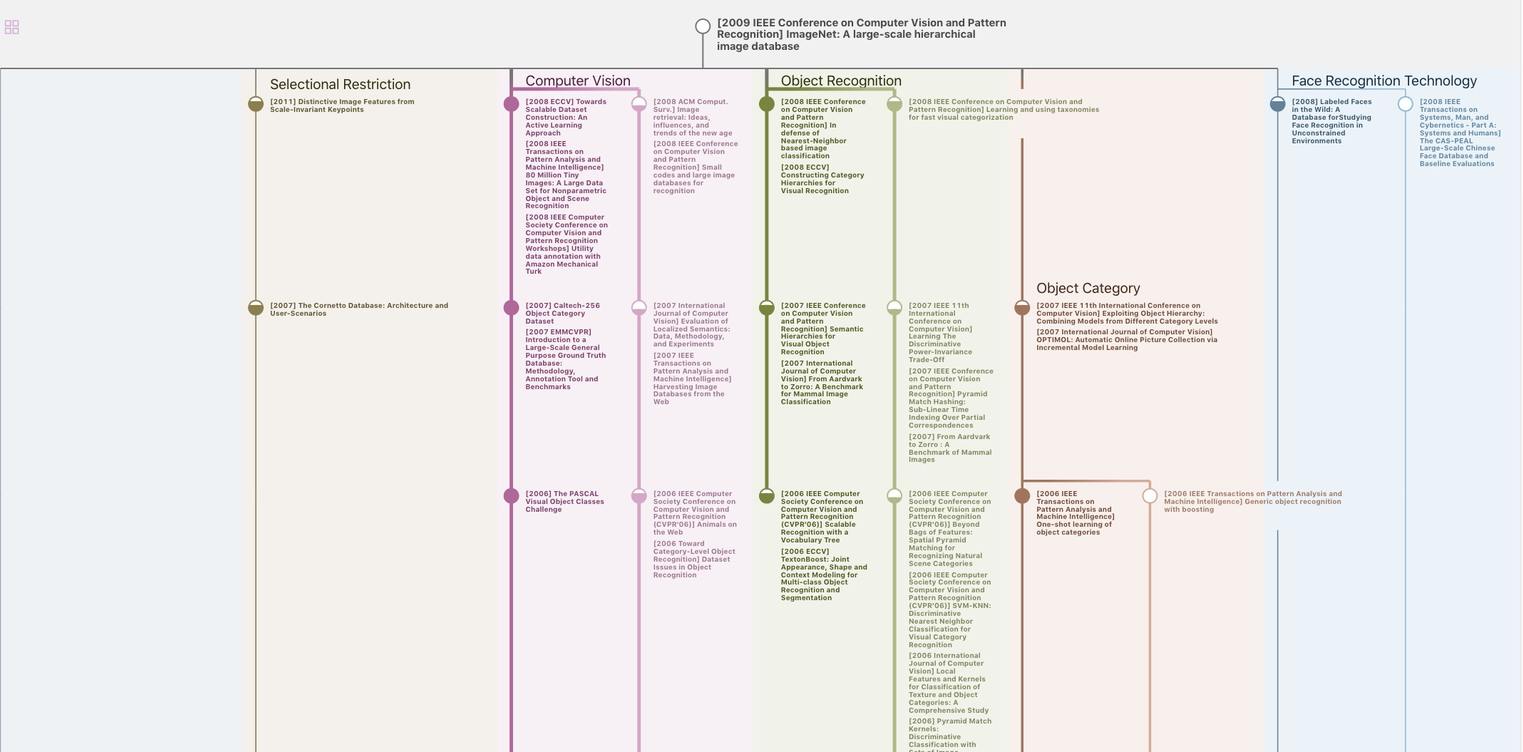
生成溯源树,研究论文发展脉络
Chat Paper
正在生成论文摘要