Breaking Through the Traffic Congestion: Asynchronous Time Series Data Integration and Xgboost for Accurate Traffic Density Prediction.
Winter Simulation Conference(2023)
摘要
The proliferation of data collection from smart cities has resulted in an exponential growth in the volume of measurements available for analysis. However, collecting all parameters concurrently at the same location is not feasible due to the complex nature of the real world. We present an innovative methodology that enriches asynchronous time series data from a variety of sources to facilitate data enrichment and city-wide behaviour simulation. A case study on OpenDataBCN attests to the efficacy of this approach via an XGBoost model, predicated on geographical coordinates and timestamp disparities. The consolidation of data from different sources improves the richness and granularity of information at disposal for analysis, thereby revealing previously hidden patterns and relationships, exhibiting new insights and underscoring the potential of this methodology for sustainable and efficient data enrichment processes as well as new possibilities for simulation based on smart city datasets.
更多查看译文
关键词
Time Series,Data Integration,Time Series Data,Traffic Congestion,Traffic Prediction,Asynchronous Data,Accurate Traffic Prediction,Geographic Coordinates,Volume Growth,XGBoost Model,Granular Information,Consolidated Data,Machine Learning,Accuracy Of Model,Receiver Operating Characteristic,Machine Learning Models,Minimum Distance,Minimal Differences,New Variables,Open Data,SHapley Additive exPlanations,Closest Measurements,Set Of Data Points,Sections Of The City,Traffic Data,Advanced Machine Learning Techniques,Urban Mobility,Pollution Data,Integration Of Data Sources,Traffic Patterns
AI 理解论文
溯源树
样例
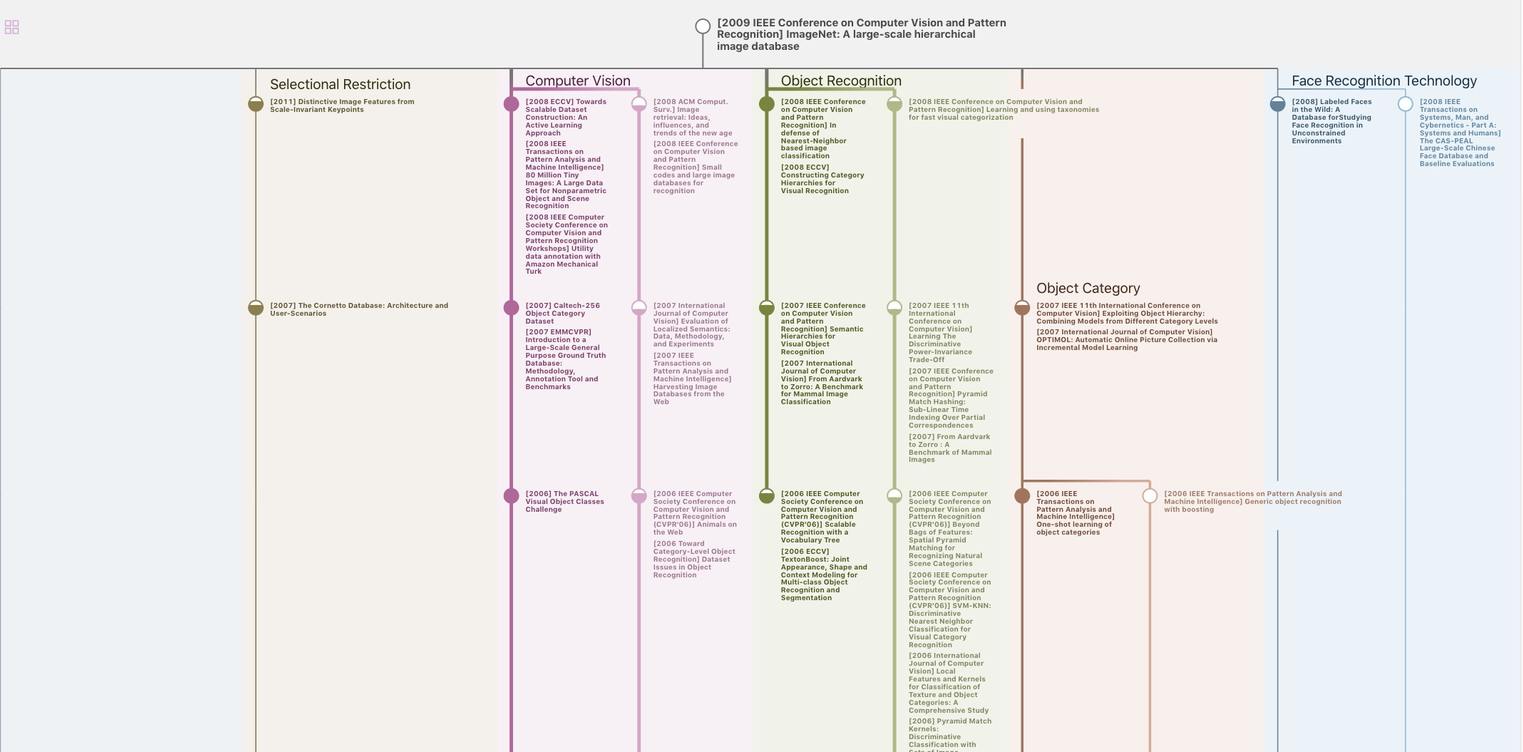
生成溯源树,研究论文发展脉络
Chat Paper
正在生成论文摘要