A novel approach for detecting deep fake videos using graph neural network
Journal of Big Data(2024)
摘要
Deep fake technology has emerged as a double-edged sword in the digital world. While it holds potential for legitimate uses, it can also be exploited to manipulate video content, causing severe social and security concerns. The research gap lies in the fact that traditional deep fake detection methods, such as visual quality analysis or inconsistency detection, need help to keep up with the rapidly advancing technology used to create deep fakes. That means there's a need for more sophisticated detection techniques. This paper introduces an enhanced approach for detecting deep fake videos using graph neural network (GNN). The proposed method splits the detection process into two phases: a mini-batch graph convolution network stream four-block CNN stream comprising Convolution, Batch Normalization, and Activation function. The final step is a flattening operation, which is essential for connecting the convolutional layers to the dense layer. The fusion of these two phases is performed using three different fusion networks: FuNet-A (additive fusion), FuNet-M (element-wise multiplicative fusion), and FuNet-C (concatenation fusion). The paper further evaluates the proposed model on different datasets, where it achieved an impressive training and validation accuracy of 99.3% after 30 epochs.
更多查看译文
关键词
Graph neural network,Convolutional neural network,Deepfake video detection,Multi-task cascaded convolutional neural network,Mini-GNN
AI 理解论文
溯源树
样例
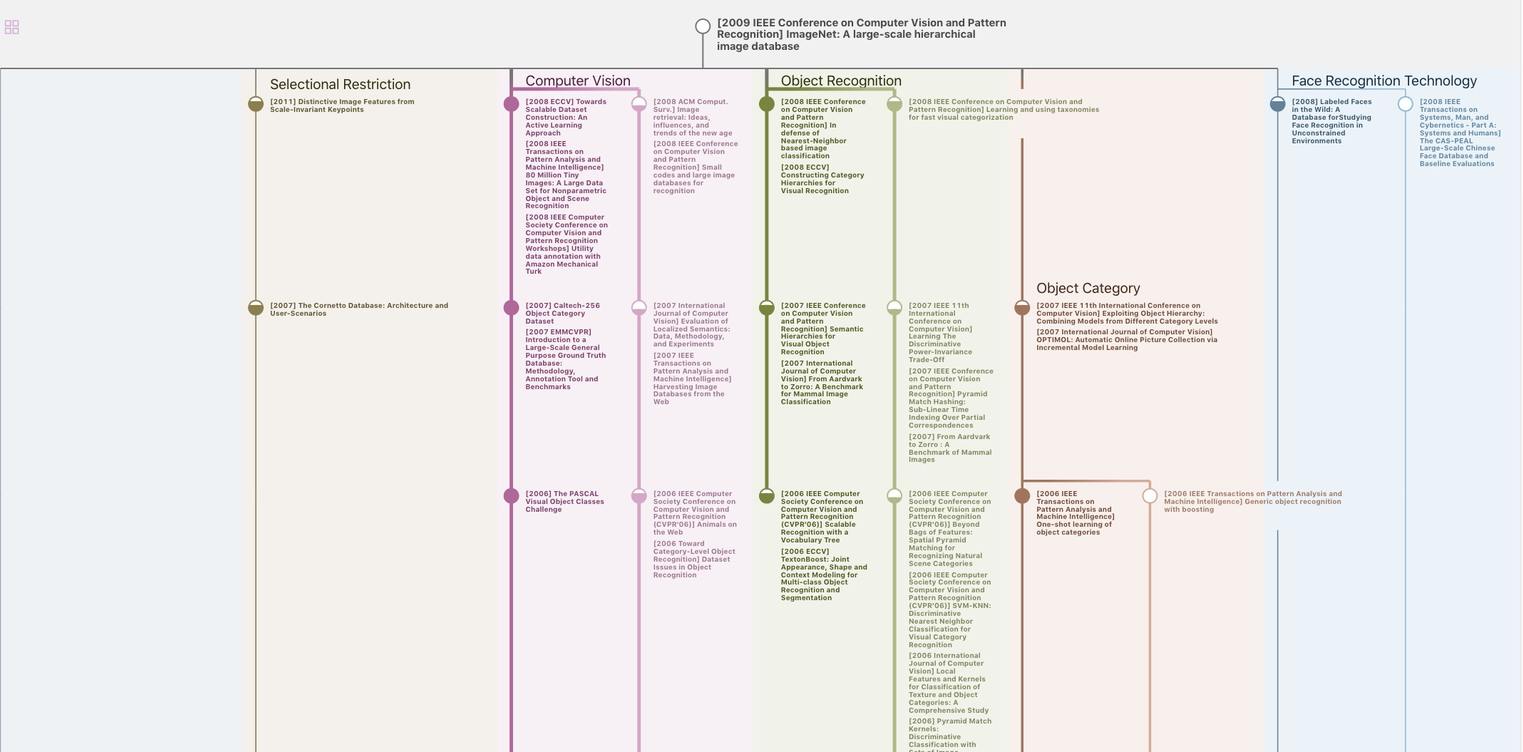
生成溯源树,研究论文发展脉络
Chat Paper
正在生成论文摘要