Unsupervised Manufacturing Fault Detection Based on Self-labeled Training of Fingerprint Image Constructed from Time-Series Data
International Journal of Precision Engineering and Manufacturing(2024)
摘要
The acquisition of properly labeled datasets is challenging, which hampers the implementation of industrial deep learning technology in actual manufacturing sites. This paper proposes an unsupervised manufacturing fault detection method based on self-labeled training to remedy the lack of properly labeled datasets. The proposed method comprises a two-step process of time-series imaging, termed fingerprinting, and normality calculation using self-labeled classification in a deep learning architecture. We compared our model with state-of-art one-class classification algorithms using an unlabeled dataset, which was obtained by varying the ratio of production failure samples in datasets. The proposed model exhibited better performance than baseline algorithms in terms of area under receiver operating curve (AUROC), even for the one-class classification using datasets comprising production success cases only. Moreover, more robust performance was observed compared to the baseline algorithms in several contamination conditions of datasets where the datasets comprised different proportions of production failure cases to simulate unlabeled manufacturing data. Our results suggest that the self-labeling-based fault detection model we propose is adaptable to both unsupervised and semi-supervised conditions. It can effectively enhance the detection of defects in scenarios characterized by exceptionally rare failure cases that closely resemble real-world situations.
更多查看译文
关键词
Fault detection,Unsupervised learning,Anomaly detection,One-class classification,Deep learning
AI 理解论文
溯源树
样例
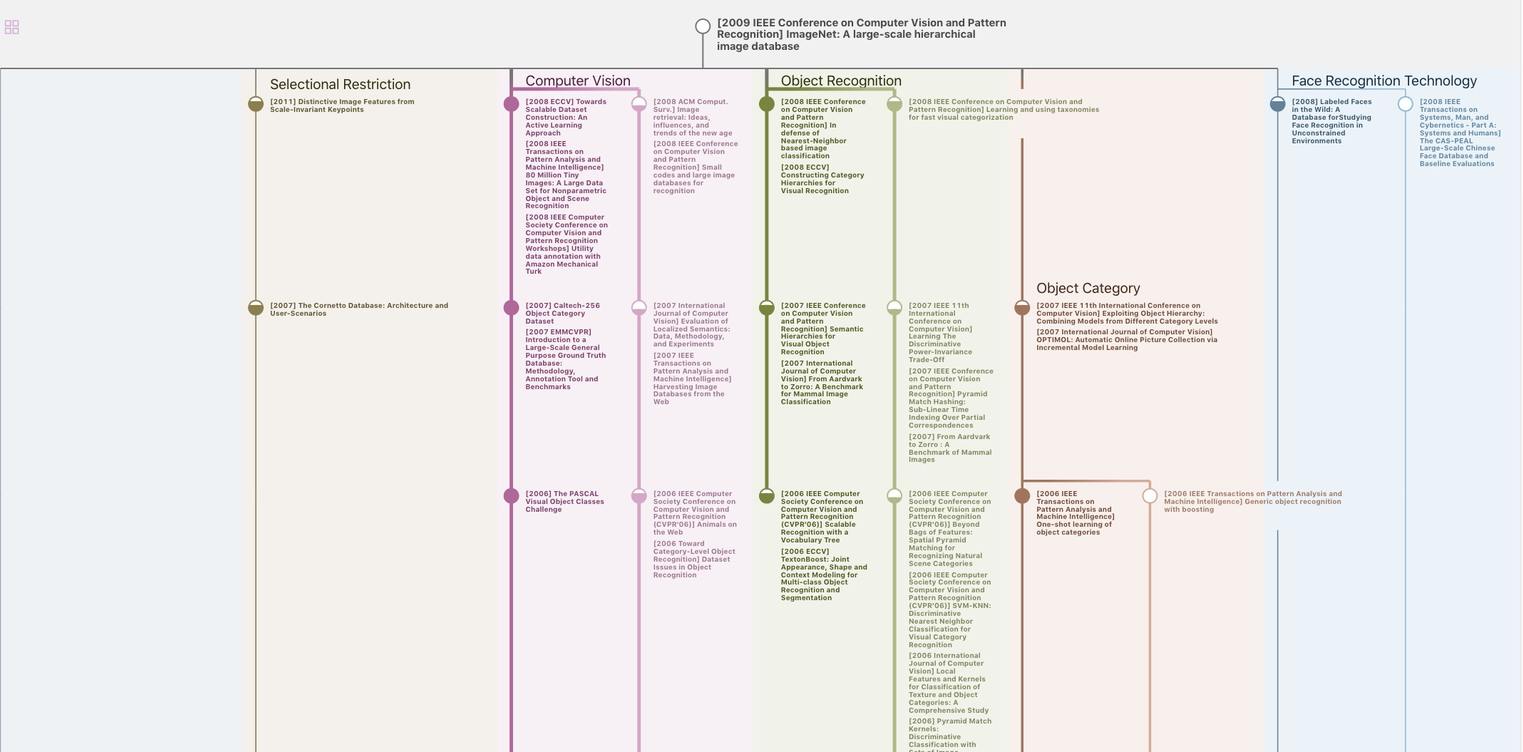
生成溯源树,研究论文发展脉络
Chat Paper
正在生成论文摘要