Reconstruction of global ionospheric TEC maps from IRI-2020 model based on deep learning method
Journal of Geodesy(2024)
摘要
The Total Electron Content (TEC) computed from ionospheric models is a widely used parameter for characterizing the morphological structure of the ionosphere. The global TEC maps from empirical models, like the International Reference Ionosphere (IRI) model, have limited accuracy compared to those calculated by dual-frequency measurements from the global navigation satellite systems (GNSS). We have developed a reconstructed IRI TEC model for generating high-precision global TEC maps based on a deep learning method. For this, we have collected 48,204 pairs of global TEC maps from the IRI-2020 model and Global Ionosphere Maps (GIM) model with 2-h time resolution from 2009 to 2019 covering the whole solar cycle 24. The daily solar radio flux ( F 10.7 ), sunspot number (SSN), Dst, and K p indices are also introduced as input features to train the model. We have investigated the optimum combination of the input parameters for the reconstructed TEC model and compared the performance of the model during the years with high and low solar activity levels. Results show that the reconstructed TEC model with F 10.7 and K p features has a better performance compared to that considering all solar and geomagnetic indices. The global TEC maps predicted from our model are much more consistent with the corresponding TEC maps from the GIM model than those from the IRI-2020 model. Especially, the large-scale equatorial ionospheric anomaly (EIA) crests and the pronounced enhancement of TEC are well predicted by the reconstructed TEC model. From statistical metrics, the accuracy of the reconstructed TEC model increased by 40.8% during the high solar activity year 2015 and 43.0% during the low solar activity year 2018 compared with the IRI-2020 model. The prediction performance of the reconstructed TEC model also shows better accuracy during the storm periods.
更多查看译文
关键词
Ionosphere,Total electron content (TEC),International reference ionosphere (IRI),Geomagnetic and solar indices,Deep learning
AI 理解论文
溯源树
样例
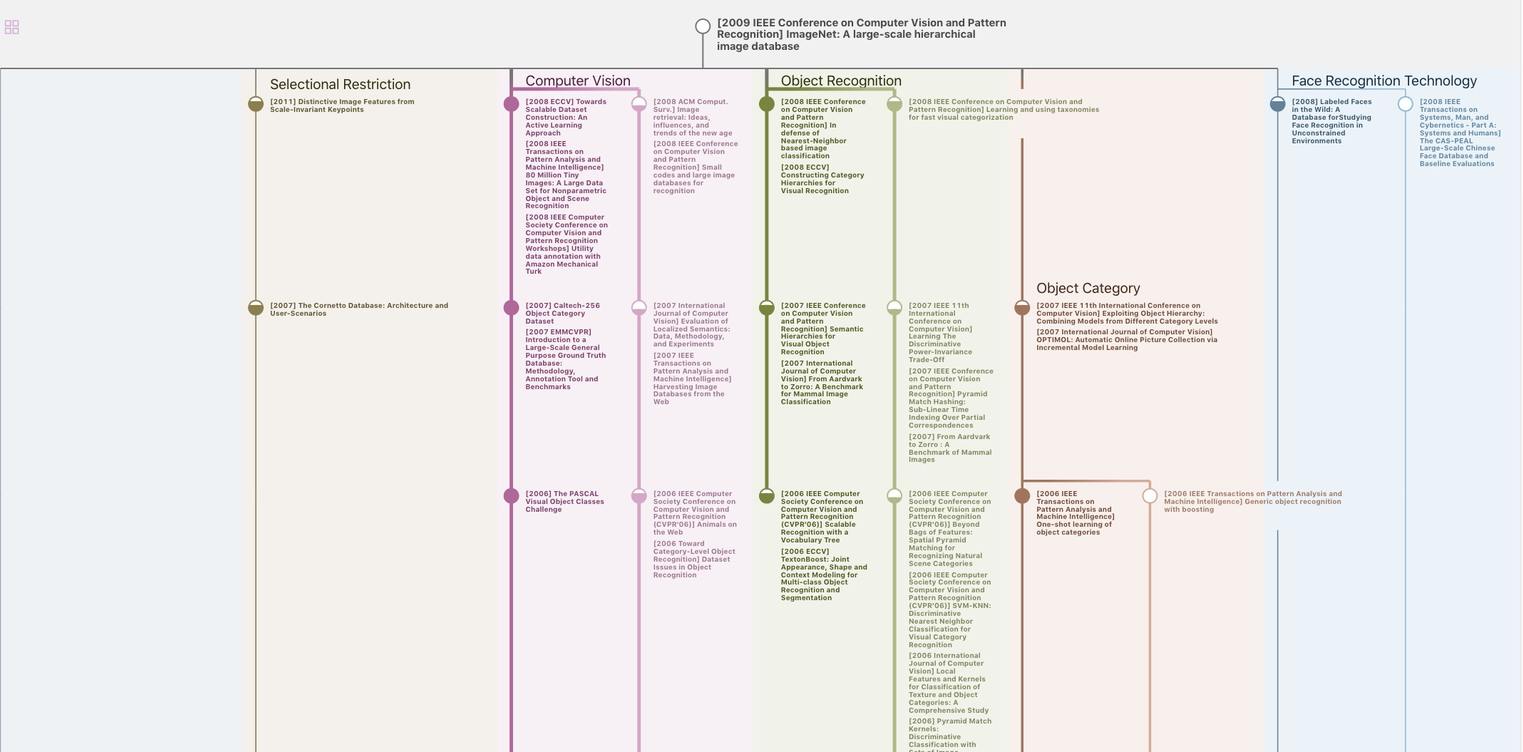
生成溯源树,研究论文发展脉络
Chat Paper
正在生成论文摘要