Improving lesion detection in mammograms by leveraging a Cycle-GAN-based lesion remover
Breast Cancer Research(2024)
摘要
Background The wide heterogeneity in the appearance of breast lesions and normal breast structures can confuse computerized detection algorithms. Our purpose was therefore to develop a Lesion Highlighter (LH) that can improve the performance of computer-aided detection algorithms for detecting breast cancer on screening mammograms. Methods We hypothesized that a Cycle-GAN based Lesion Remover (LR) could act as an LH , which can improve the performance of lesion detection algorithms. We used 10,310 screening mammograms from 4,832 women that included 4,942 recalled lesions (BI-RADS 0) and 5,368 normal results (BI-RADS 1). We divided the dataset into Train:Validate:Test folds with the ratios of 0.64:0.16:0.2. We segmented image patches (400 × 400 pixels) from either lesions marked by MQSA radiologists or normal tissue in mammograms. We trained a Cycle-GAN to develop two GANs, where each GAN transferred the style of one image to another. We refer to the GAN transferring the style of a lesion to normal breast tissue as the LR. We then highlighted the lesion by color-fusing the mammogram after applying the LR to its original. Using ResNet18, DenseNet201, EfficientNetV2, and Vision Transformer as backbone architectures, we trained three deep networks for each architecture, one trained on lesion highlighted mammograms (Highlighted), another trained on the original mammograms (Baseline), and Highlighted and Baseline combined (Combined). We conducted ROC analysis for the three versions of each deep network on the test set. Results The Combined version of all networks achieved AUCs ranging from 0.963 to 0.974 for identifying the image with a recalled lesion from a normal breast tissue image, which was statistically improved (p-value < 0.001) over their Baseline versions with AUCs that ranged from 0.914 to 0.967. Conclusions Our results showed that a Cycle-GAN based LR is effective for enhancing lesion conspicuity and this can improve the performance of a detection algorithm.
更多查看译文
关键词
Lesion highlight,Convolutional neural network,Cycle generative adversarial network,Computer-aided detection
AI 理解论文
溯源树
样例
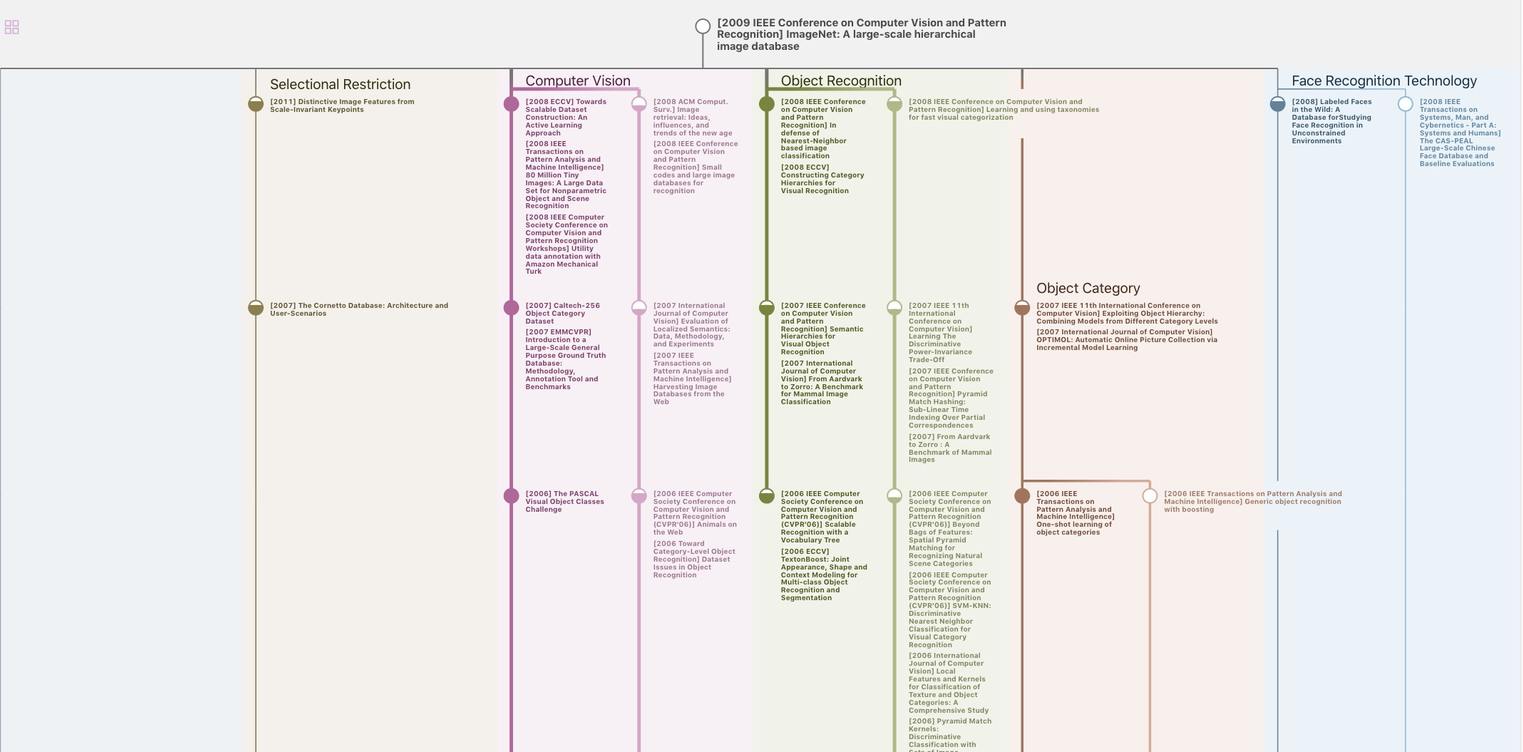
生成溯源树,研究论文发展脉络
Chat Paper
正在生成论文摘要