A Multivariate Logistic Regression Model with Over Sampling for Bronchopulmonary Dysplasia Associated with Pulmonary Hypertension in Very Preterm Infants: A Multi-Center Retrospective Study
2023 IEEE International Conference on Medical Artificial Intelligence (MedAI)(2023)
摘要
Bronchopulmonary dysplasia associated with pulmonary hypertension (BPD-PH) is considered as one of the most serious complications in premature infants, and its early detection and intervention can help improve the patients' prognosis. This study aims to apply machine learning technique to predict very premature infants with BPD-PH. 761 clinical records were collected from the neonatology department of four public hospitals in China. After data preprocessing, feature selection and model selection, 5 of 47 features, including invasive ventilator time, BPD index, ventilator-associated pneumonia, pulmonary hemorrhage, and early diagnosis of PH are used to build a multivariate logistic regression model. To work around the unbalancing issue of the dataset, oversampling algorithms have been applied to improve the model and achieve 94% recall, 88% accuracy, and 0.94 area under the receiver operating characteristic curve, which is sufficient to support clinicians to make early diagnosis and form better treatment plan for patients with BPD-PH.
更多查看译文
关键词
bronchopulmonary dysplasia,pulmonary hypertension,very preterm infants,artificial intelligence,prediction model,oversampling
AI 理解论文
溯源树
样例
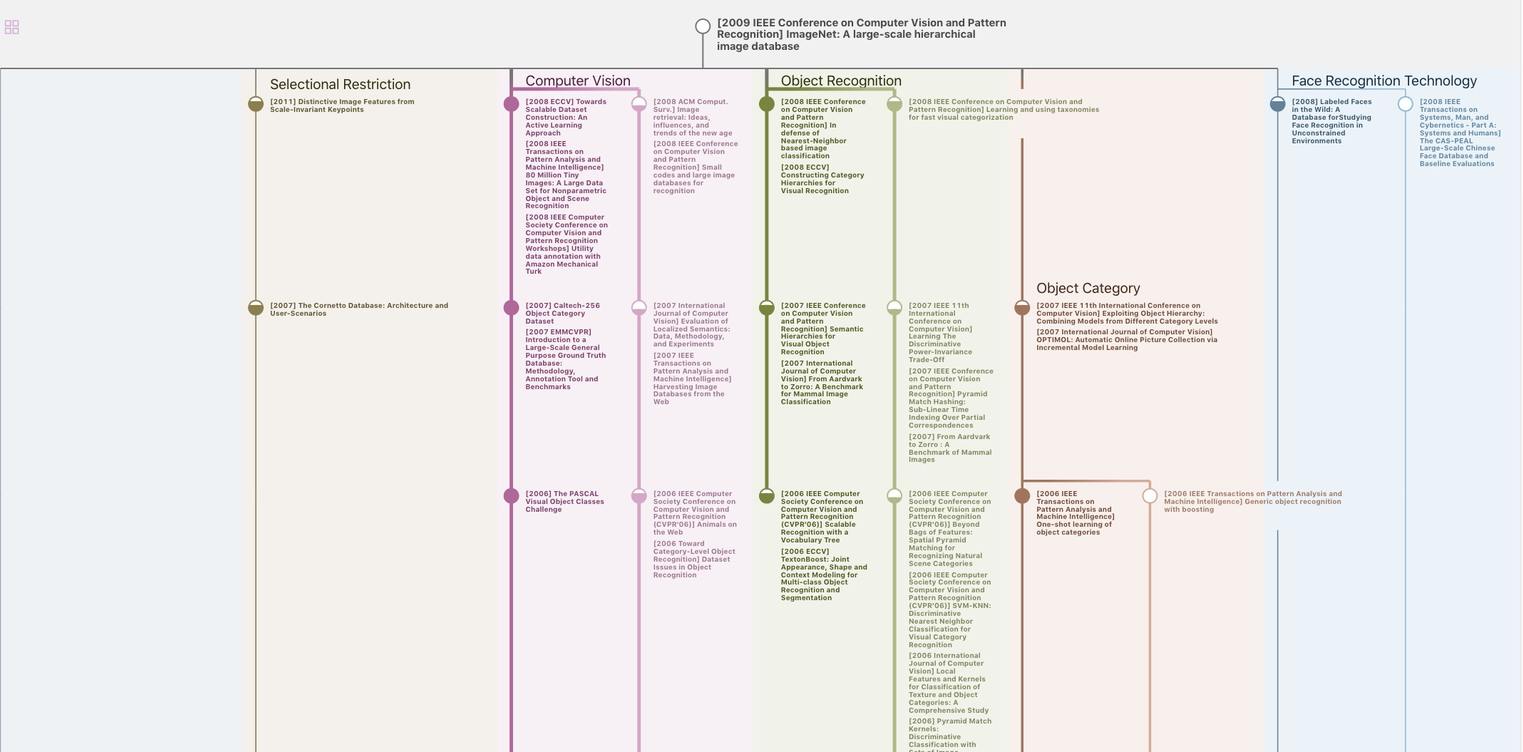
生成溯源树,研究论文发展脉络
Chat Paper
正在生成论文摘要