Efficient Input Uncertainty Quantification for Regenerative Simulation.
Winter Simulation Conference(2023)
摘要
The initial bias in steady-state simulation can be characterized as the bias of a ratio estimator if the simulation model has a regenerative structure. This work tackles input uncertainty quantification for a regenerative simulation model when its input distributions are estimated from finite data. Our aim is to construct a bootstrap-based confidence interval (CI) for the true simulation output mean performance that provides a correct coverage with significantly less computational cost than the traditional methods. Exploiting the regenerative structure, we propose a k-nearest neighbor (kNN) ratio estimator for the steady-state performance measure at each set of bootstrapped input models and construct a bootstrap CI from the computed estimators. Asymptotically optimal choices for k and bootstrap sample size are discussed. We further improve the CI by combining the kNN and likelihood ratio methods. We empirically compare the efficiency of the proposed estimators with the standard estimator using queueing examples.
更多查看译文
关键词
Input Uncertainty,Simulation Model,Bootstrap Samples,K-nearest Neighbor,Model Input,Standard Estimates,Ratio Estimates,Bootstrap Confidence Intervals,Input Distribution,Steady-state Measurements,Steady-state Simulations,Likelihood Ratio Method,kNN Method,Mean Square Error,Maximum Likelihood Estimation,Remainder Of This Paper,Empirical Analysis,Sample Mean,Ellipsoid,Choice Of Parameters,Regeneration Cycles,Inter-arrival Time,Confidence Interval Width,Service Time,Exponential Family,Simulation Error,Canonical Form,Quantile Estimation,Central Limit Theorem,Taylor Expansion
AI 理解论文
溯源树
样例
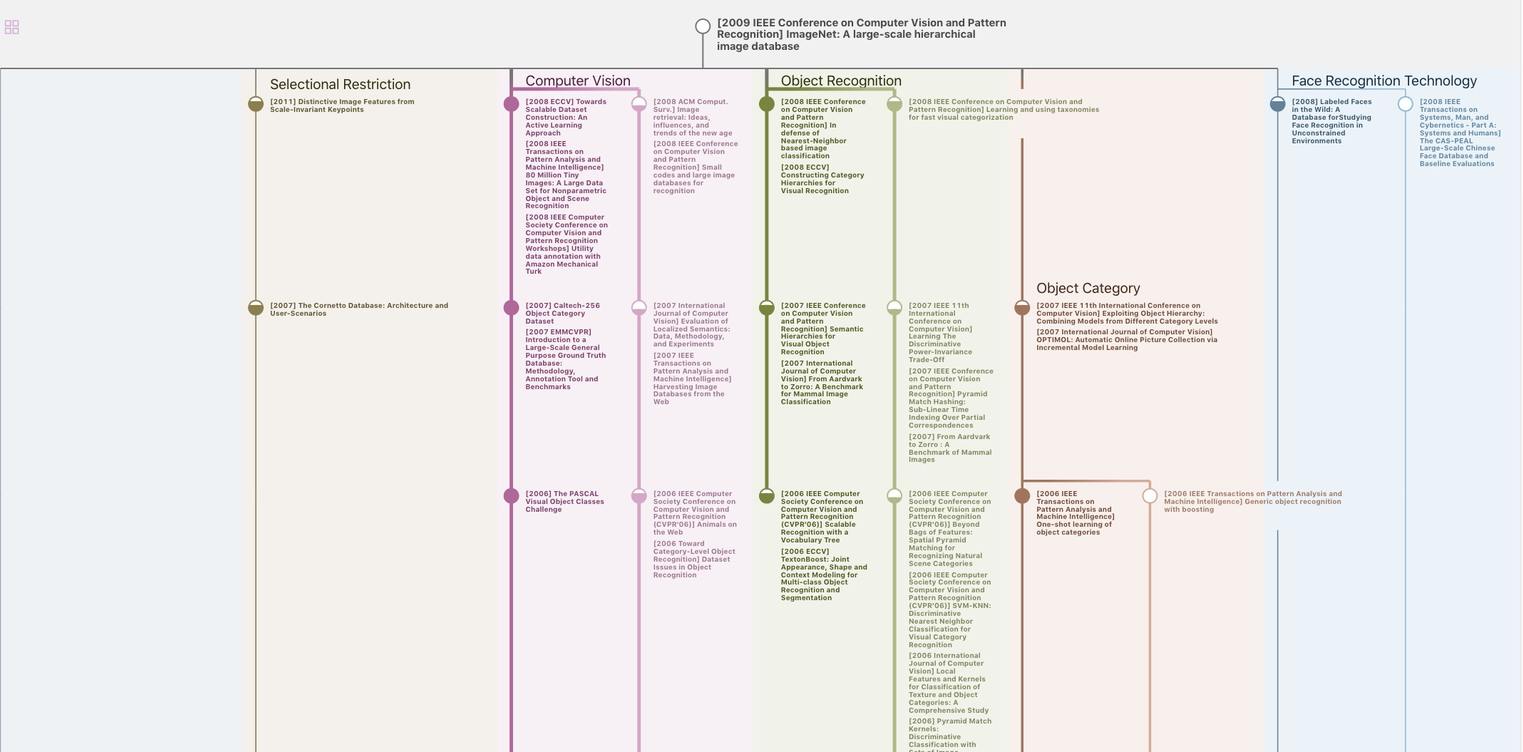
生成溯源树,研究论文发展脉络
Chat Paper
正在生成论文摘要