Learning from heterogeneous data with deep gaussian processes
2023 IEEE 9TH INTERNATIONAL WORKSHOP ON COMPUTATIONAL ADVANCES IN MULTI-SENSOR ADAPTIVE PROCESSING, CAMSAP(2023)
摘要
Deep Gaussian processes (DGPs) are deep models represented by layers of Gaussian processes (GPs). They are flexible Bayesian models capable of capturing highly nonlinear functions while providing well-calibrated uncertainties of the made predictions. Despite these strengths, DGPs encounter difficulties with real-world applications involving heterogeneous datasets and missing values. In this paper, we propose a two-stage framework for DGPs that leverages all available data in such settings. Our inference is performed within a scalable stochastic variational framework, where the variational posterior distributions are reparameterized through sparse GPs. We derive a formulation of the variational lower bound, effectively handling heterogeneous data. Further, through our inference method, we demonstrate the effective use of the GP framework on heterogeneous data. We evaluate our method on a dataset generated by a novel psychosocial screening tool called PROMOTE, which was designed to acquire data for predicting adverse perinatal outcomes and maternal mental health morbidities. Our experimental evaluation of the dataset indicates the effectiveness of our inference framework on various important learning tasks. We demonstrate the model's performance by benchmarking against existing models for high-dimensional heterogeneous data examples.
更多查看译文
关键词
Heterogeneous Data,Gaussian Process,Deep Gaussian Processes,Distribution Of Variables,Adverse Mental Health,Inference Framework,Adverse Maternal Health,Covariance Matrix,Latent Variables,Kernel Function,Financial Statements,Latent Space,Data Space,Latent Variable Model,Variational Inference
AI 理解论文
溯源树
样例
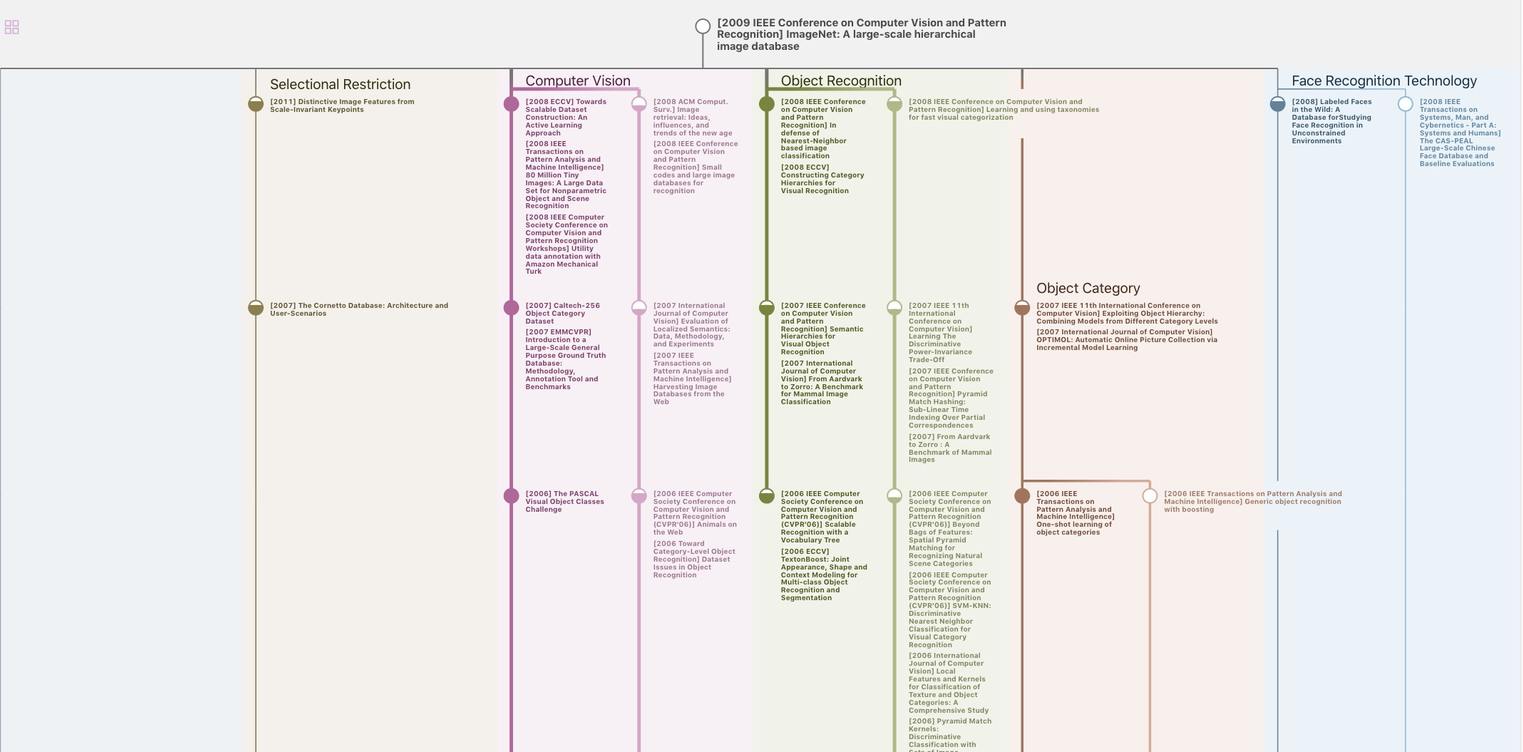
生成溯源树,研究论文发展脉络
Chat Paper
正在生成论文摘要