Hierarchical Incentive Mechanism for Federated Learning: A Single Contract to Dual Contract Approach for Smart Industries
INTERNATIONAL JOURNAL OF INTELLIGENT SYSTEMS(2024)
摘要
Federated learning (FL) has shown promise in smart industries as a means of training machine-learning models while preserving privacy. However, it contradicts FL's low communication latency requirement to rely on the cloud to transmit information with data owners in model training tasks. Furthermore, data owners may not be willing to contribute their resources for free. To address this, we propose a single contract to dual contract approach to incentivize both model owners and workers to participate in FL-based machine learning tasks. The single-contract incentivizes model owners to contribute their model parameters, and the dual contract incentivizes workers to use their latest data to participate in the training task. The latest data draw out the trade-off between data quantity and data update frequency. Performance evaluation shows that our dual contract satisfies different preferences for data quantity and update frequency, and validates that the proposed incentive mechanism is incentive compatible and flexible.
更多查看译文
AI 理解论文
溯源树
样例
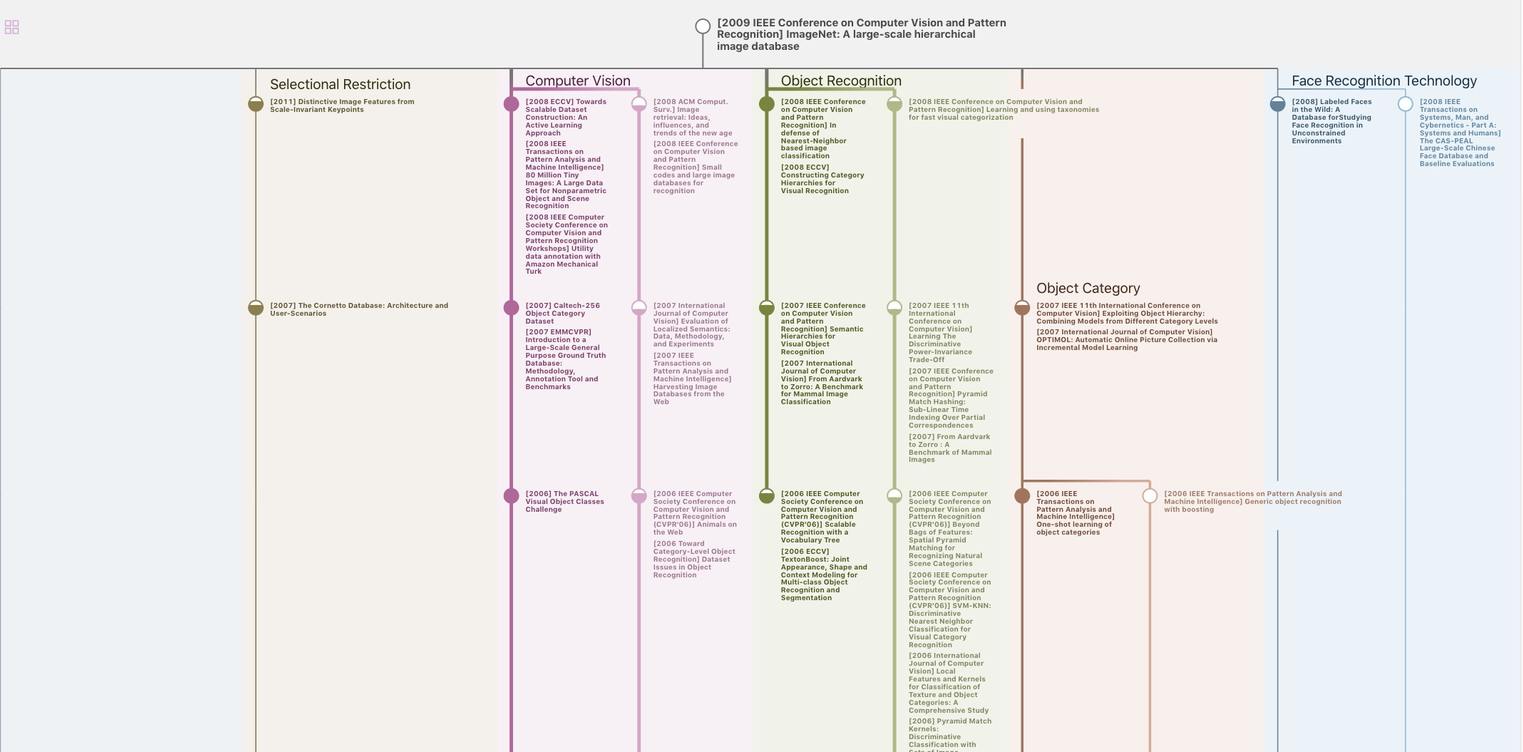
生成溯源树,研究论文发展脉络
Chat Paper
正在生成论文摘要