PVLR: Prompt-driven Visual-Linguistic Representation Learning for Multi-Label Image Recognition
CoRR(2024)
摘要
Multi-label image recognition is a fundamental task in computer vision.
Recently, vision-language models have made notable advancements in this area.
However, previous methods often failed to effectively leverage the rich
knowledge within language models and instead incorporated label semantics into
visual features in a unidirectional manner. In this paper, we propose a
Prompt-driven Visual-Linguistic Representation Learning (PVLR) framework to
better leverage the capabilities of the linguistic modality. In PVLR, we first
introduce a dual-prompting strategy comprising Knowledge-Aware Prompting (KAP)
and Context-Aware Prompting (CAP). KAP utilizes fixed prompts to capture the
intrinsic semantic knowledge and relationships across all labels, while CAP
employs learnable prompts to capture context-aware label semantics and
relationships. Later, we propose an Interaction and Fusion Module (IFM) to
interact and fuse the representations obtained from KAP and CAP. In contrast to
the unidirectional fusion in previous works, we introduce a Dual-Modal
Attention (DMA) that enables bidirectional interaction between textual and
visual features, yielding context-aware label representations and
semantic-related visual representations, which are subsequently used to
calculate similarities and generate final predictions for all labels. Extensive
experiments on three popular datasets including MS-COCO, Pascal VOC 2007, and
NUS-WIDE demonstrate the superiority of PVLR.
更多查看译文
AI 理解论文
溯源树
样例
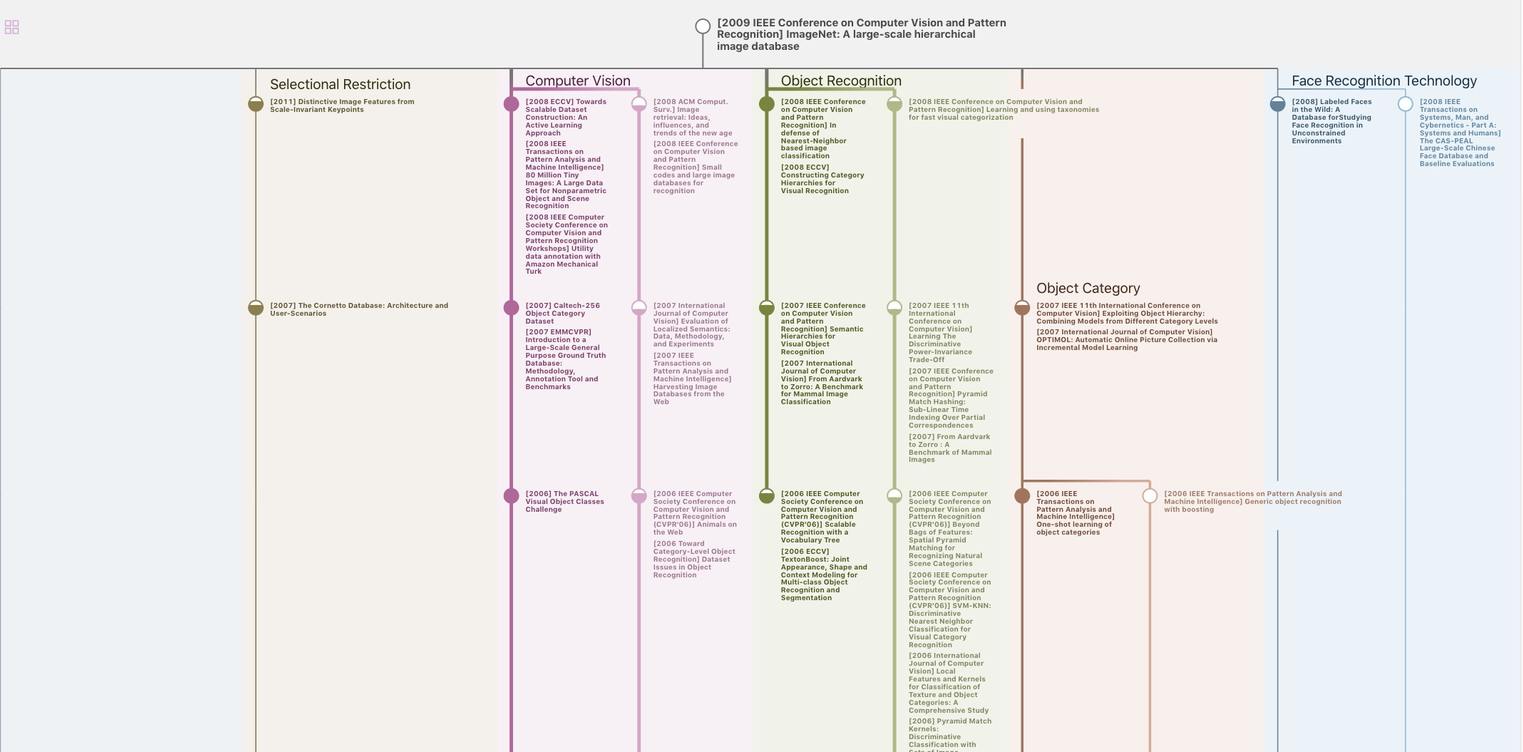
生成溯源树,研究论文发展脉络
Chat Paper
正在生成论文摘要