Liquid Democracy for Low-Cost Ensemble Pruning
CoRR(2024)
摘要
We argue that there is a strong connection between ensemble learning and a
delegative voting paradigm – liquid democracy – that can be leveraged to
reduce ensemble training costs. We present an incremental training procedure
that identifies and removes redundant classifiers from an ensemble via
delegation mechanisms inspired by liquid democracy. Through both analysis and
extensive experiments we show that this process greatly reduces the
computational cost of training compared to training a full ensemble. By
carefully selecting the underlying delegation mechanism, weight centralization
in the classifier population is avoided, leading to higher accuracy than some
boosting methods. Furthermore, this work serves as an exemplar of how
frameworks from computational social choice literature can be applied to
problems in nontraditional domains.
更多查看译文
AI 理解论文
溯源树
样例
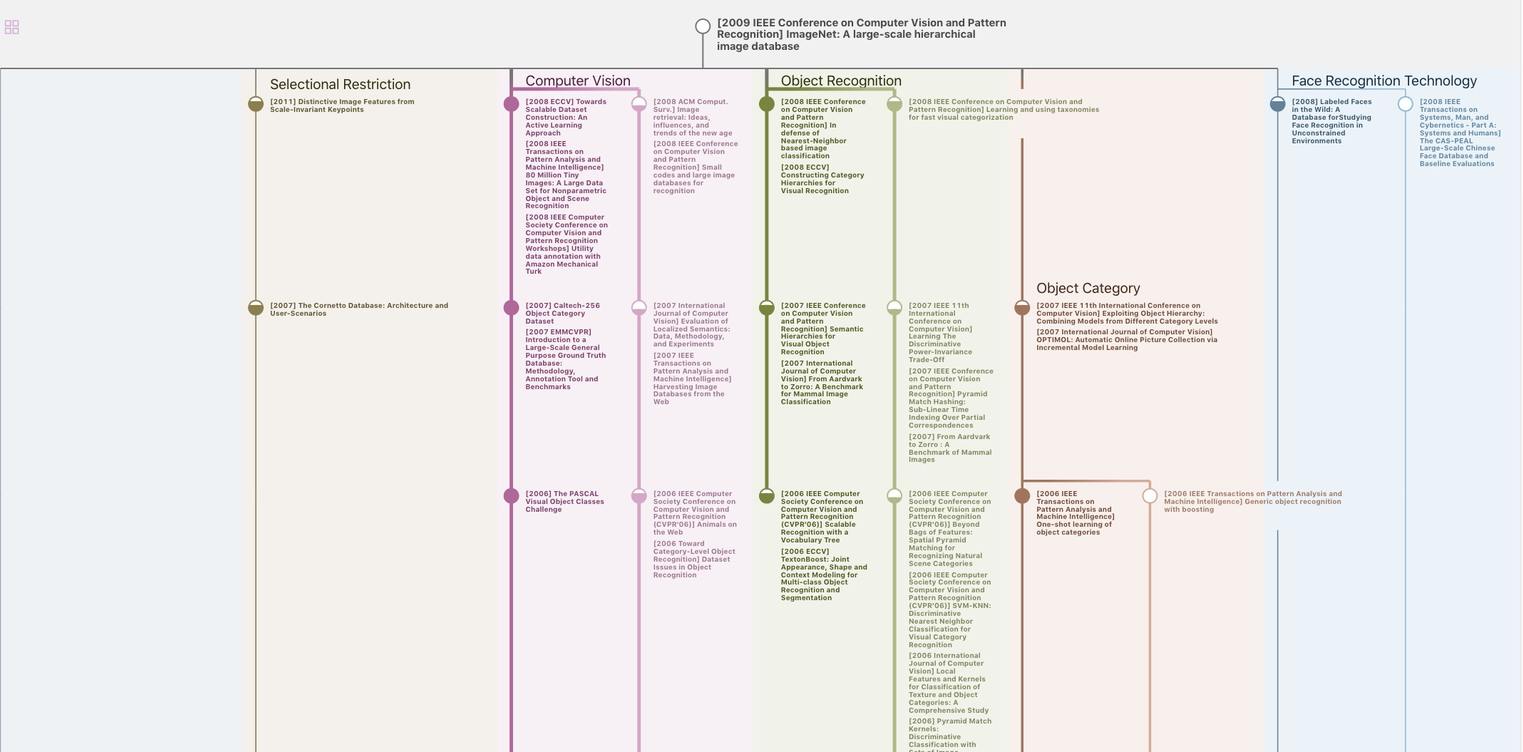
生成溯源树,研究论文发展脉络
Chat Paper
正在生成论文摘要