Mining technology trends in scientific publications: a graph propagated neural topic modeling approach
Knowledge and Information Systems(2024)
摘要
The past decades have witnessed significant progress in scientific research, where new technologies emerge and traditional technologies constantly evolve. As a critical task in the Science of Science (SciSci), automatically mining technology trends from massive scientific publications have attracted broad research interests in various communities. While existing approaches can achieve remarkable performance, there are still many critical challenges to address, such as data sparsity, cross-document influence, and temporal dependency. To this end, in this paper, we propose a technical terms-based graph propagated neural topic model for mining technology trends in scientific publications. Specifically, we first utilize the documents’ citation relations and technical terms to construct a heterogeneous graph. Then, we design a term propagation network to spread the technical terms on the heterogeneous graph to overcome the sparseness of technical terms. In addition, we develop a dynamic embedded topic modeling method to capture the temporal dependencies for technical terms in cross-document, which can discover the distribution of technical terms over time. Finally, extensive experiments on real-world scientific datasets validate the effectiveness and interpretability of our approach compared with state-of-the-art baselines.
更多查看译文
关键词
Science of science,Technology trend mining,Neural topic modeling
AI 理解论文
溯源树
样例
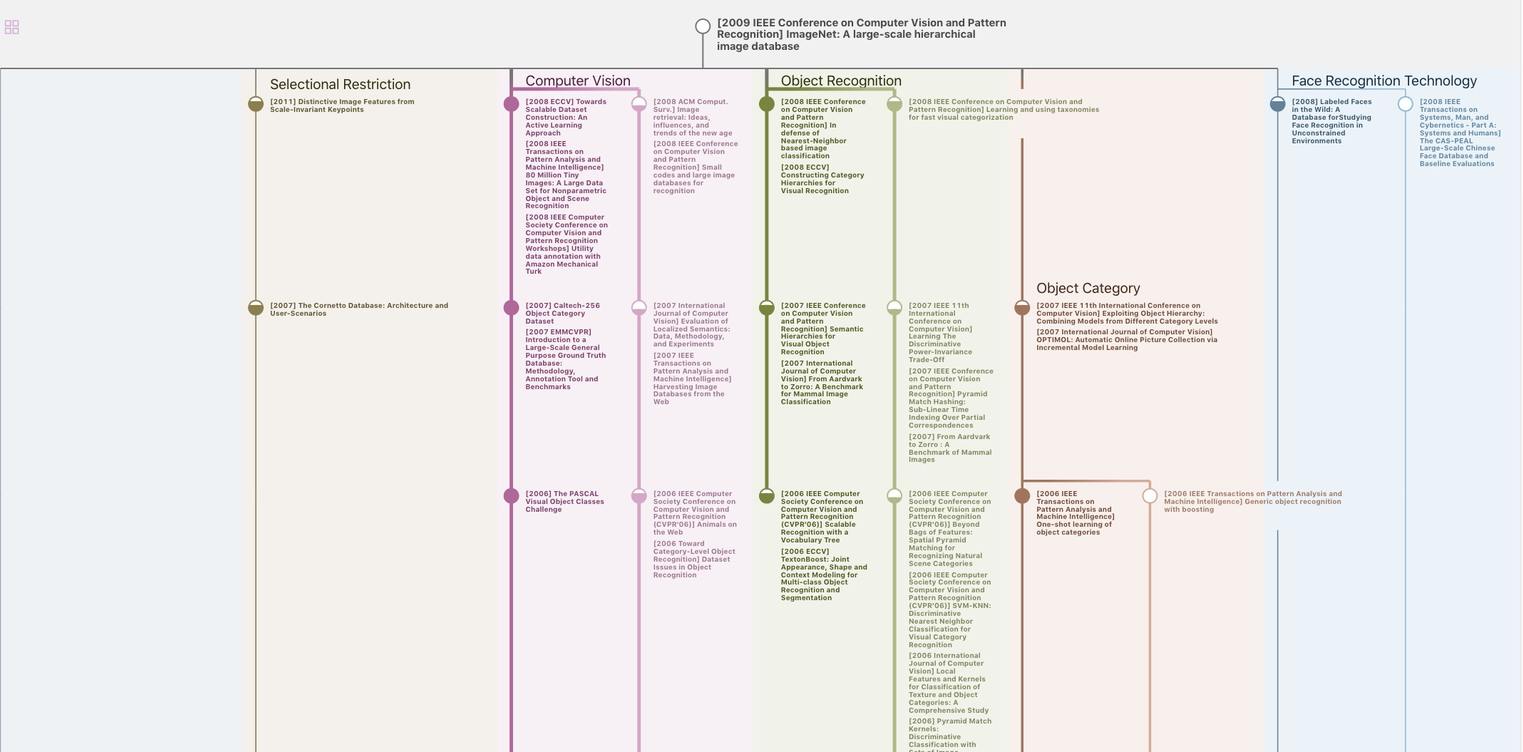
生成溯源树,研究论文发展脉络
Chat Paper
正在生成论文摘要