Improving Reliability of State Estimation by Particle Filter with Cauchy Likelihood Function
2023 7th International Conference on Information Technology (InCIT)(2023)
摘要
Particle filtering, a sequential Monte Carlo method, is a powerful framework that the posterior probability density function (PDF) of the state can be approximated by a set of random particles and their associated weights. Particle filter (PF) is composed of these steps: particle initialization, prediction, updating, and resampling. For updating step, the framework needs to calculate the likelihood function which is based on the measurement model of system. When the measurement noise is considered additive and Gaussian, the conventional Gaussian error model is utilized. However, when the measurement noise level is low, Gaussian noise model provides large prediction error. We therefore aim to remedy the problem when the measurement noise level is low, instead of using standard likelihood calculation, we propose to implement Cauchy likelihood function (CLF) for particle weight calculation to resolve the problem. Via the root mean squared error (RMSE) and mean absolute error (MAE), simulation results show that it can provide comparatively better estimation performance than Gaussian likelihood function.
更多查看译文
关键词
Cauchy likelihood function,Gaussian likelihood function,particle filter,state estimation,signal processing
AI 理解论文
溯源树
样例
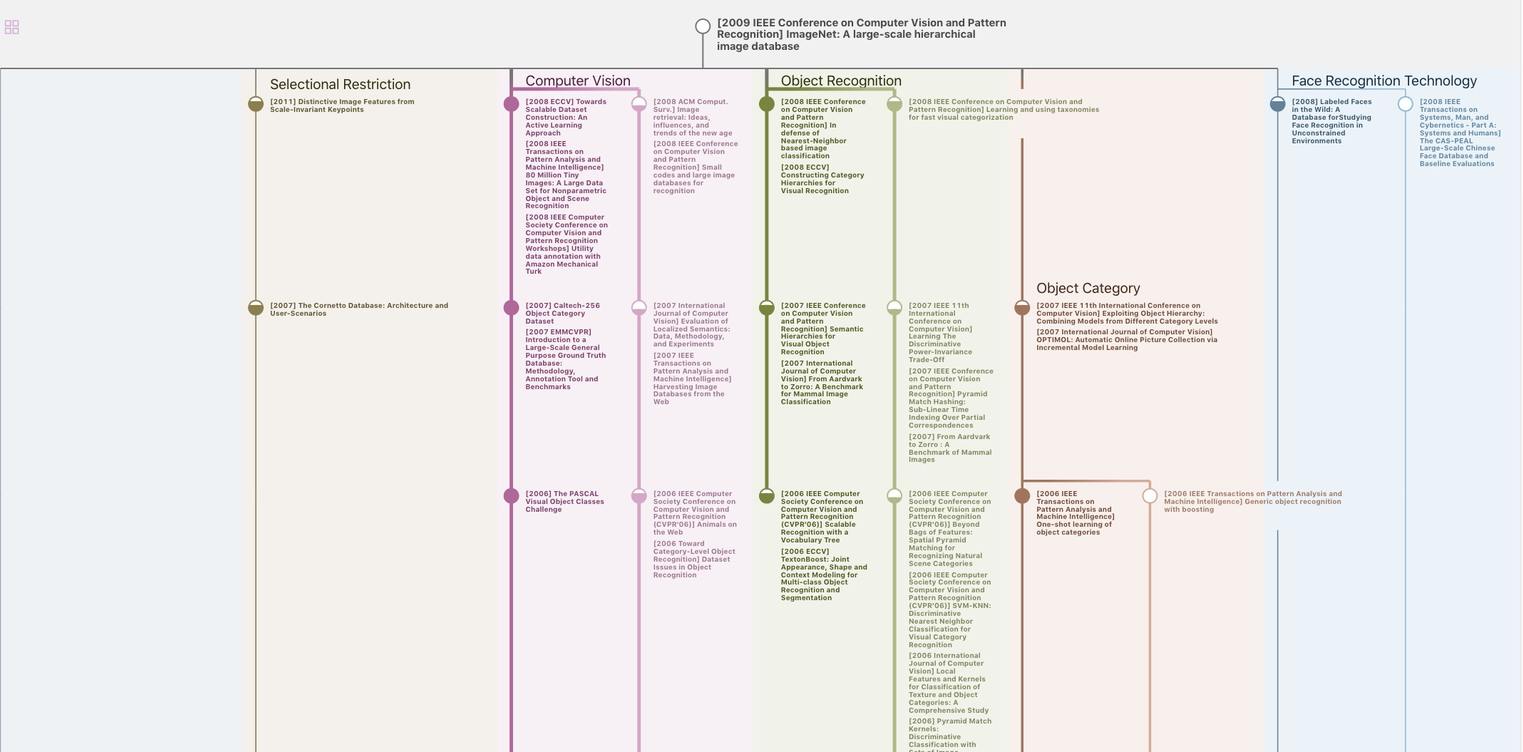
生成溯源树,研究论文发展脉络
Chat Paper
正在生成论文摘要