Weaver: Foundation Models for Creative Writing
CoRR(2024)
摘要
This work introduces Weaver, our first family of large language models (LLMs)
dedicated to content creation. Weaver is pre-trained on a carefully selected
corpus that focuses on improving the writing capabilities of large language
models. We then fine-tune Weaver for creative and professional writing purposes
and align it to the preference of professional writers using a suit of novel
methods for instruction data synthesis and LLM alignment, making it able to
produce more human-like texts and follow more diverse instructions for content
creation. The Weaver family consists of models of Weaver Mini (1.8B), Weaver
Base (6B), Weaver Pro (14B), and Weaver Ultra (34B) sizes, suitable for
different applications and can be dynamically dispatched by a routing agent
according to query complexity to balance response quality and computation cost.
Evaluation on a carefully curated benchmark for assessing the writing
capabilities of LLMs shows Weaver models of all sizes outperform generalist
LLMs several times larger than them. Notably, our most-capable Weaver Ultra
model surpasses GPT-4, a state-of-the-art generalist LLM, on various writing
scenarios, demonstrating the advantage of training specialized LLMs for writing
purposes. Moreover, Weaver natively supports retrieval-augmented generation
(RAG) and function calling (tool usage). We present various use cases of these
abilities for improving AI-assisted writing systems, including integration of
external knowledge bases, tools, or APIs, and providing personalized writing
assistance. Furthermore, we discuss and summarize a guideline and best
practices for pre-training and fine-tuning domain-specific LLMs.
更多查看译文
AI 理解论文
溯源树
样例
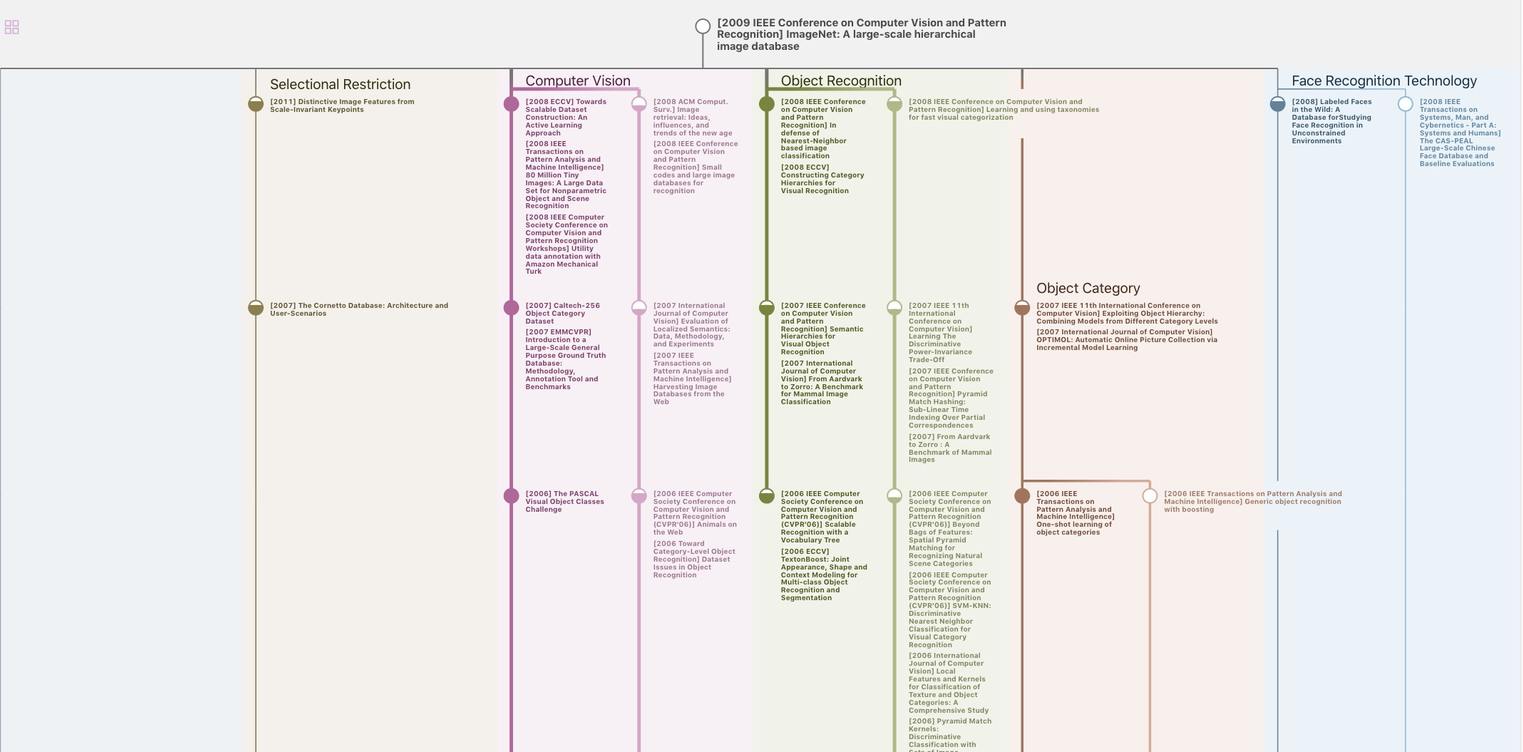
生成溯源树,研究论文发展脉络
Chat Paper
正在生成论文摘要