ReacLLaMA: Merging chemical and textual information in chemical reactivity AI models
CoRR(2024)
摘要
Chemical reactivity models are developed to predict chemical reaction
outcomes in the form of classification (success/failure) or regression (product
yield) tasks. The vast majority of the reported models are trained solely on
chemical information such as reactants, products, reagents, and solvents, but
not on the details of a synthetic protocol. Herein incorporation of procedural
text with the aim to augment the Graphormer reactivity model and improve its
accuracy is presented. Two major approaches are used: training an adapter
Graphormer model that is provided with a GPT-2-derived latent representation of
the text procedure (ReacLLaMA-Adapter) and labeling an unlabeled part of a
dataset with the LLaMA 2 model followed by training the Graphormer on an
extended dataset (Zero-Shot Labeling ReacLLaMA). Both methodologies enhance the
discernment of unpromising reactions, thereby providing more accurate models
with improved specificity.
更多查看译文
AI 理解论文
溯源树
样例
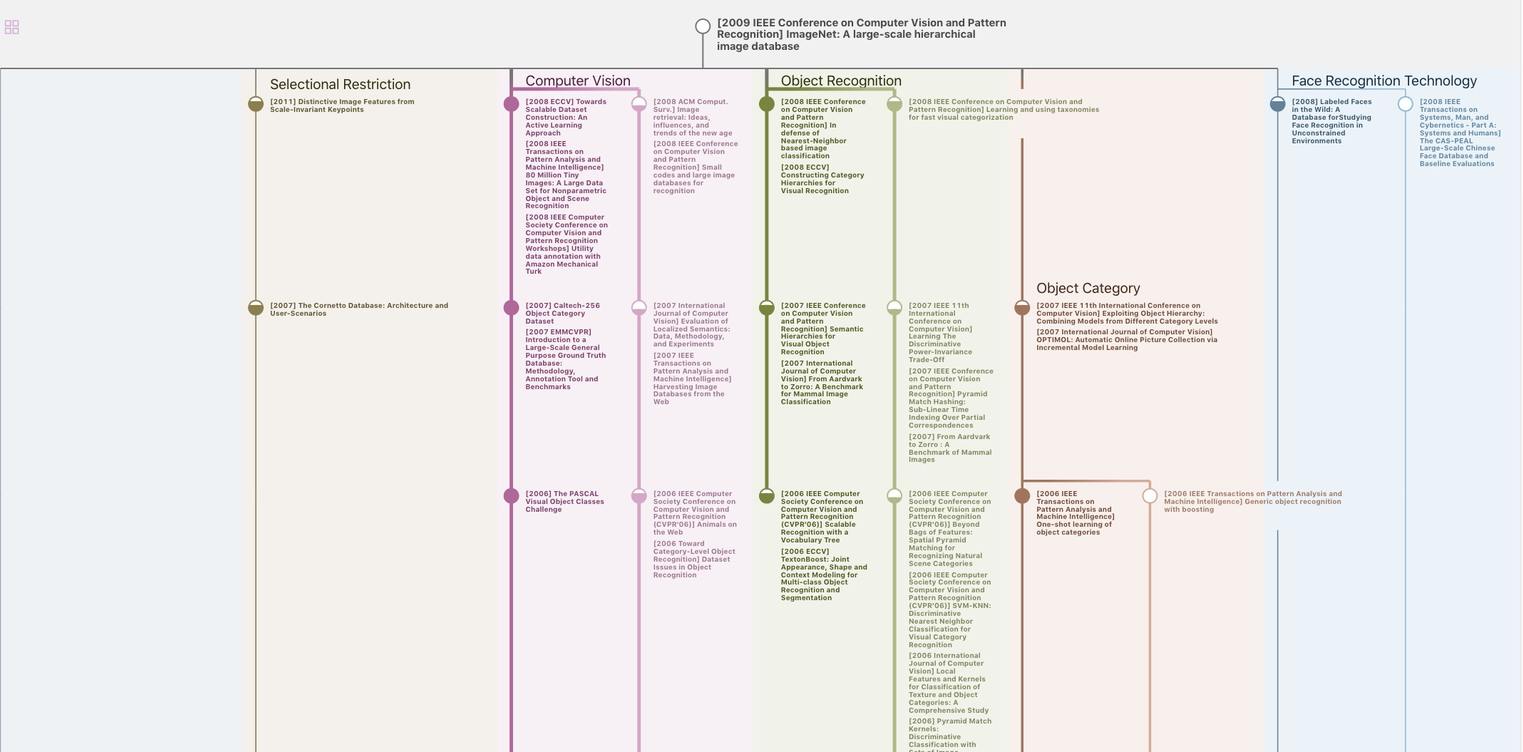
生成溯源树,研究论文发展脉络
Chat Paper
正在生成论文摘要