CAFCT: Contextual and Attentional Feature Fusions of Convolutional Neural Networks and Transformer for Liver Tumor Segmentation
CoRR(2024)
摘要
Medical image semantic segmentation techniques can help identify tumors
automatically from computed tomography (CT) scans. In this paper, we propose a
Contextual and Attentional feature Fusions enhanced Convolutional Neural
Network (CNN) and Transformer hybrid network (CAFCT) model for liver tumor
segmentation. In the proposed model, three other modules are introduced in the
network architecture: Attentional Feature Fusion (AFF), Atrous Spatial Pyramid
Pooling (ASPP) of DeepLabv3, and Attention Gates (AGs) to improve contextual
information related to tumor boundaries for accurate segmentation. Experimental
results show that the proposed CAFCT achieves a mean Intersection over Union
(IoU) of 90.38
Segmentation Benchmark (LiTS) dataset, outperforming pure CNN or Transformer
methods, e.g., Attention U-Net, and PVTFormer.
更多查看译文
AI 理解论文
溯源树
样例
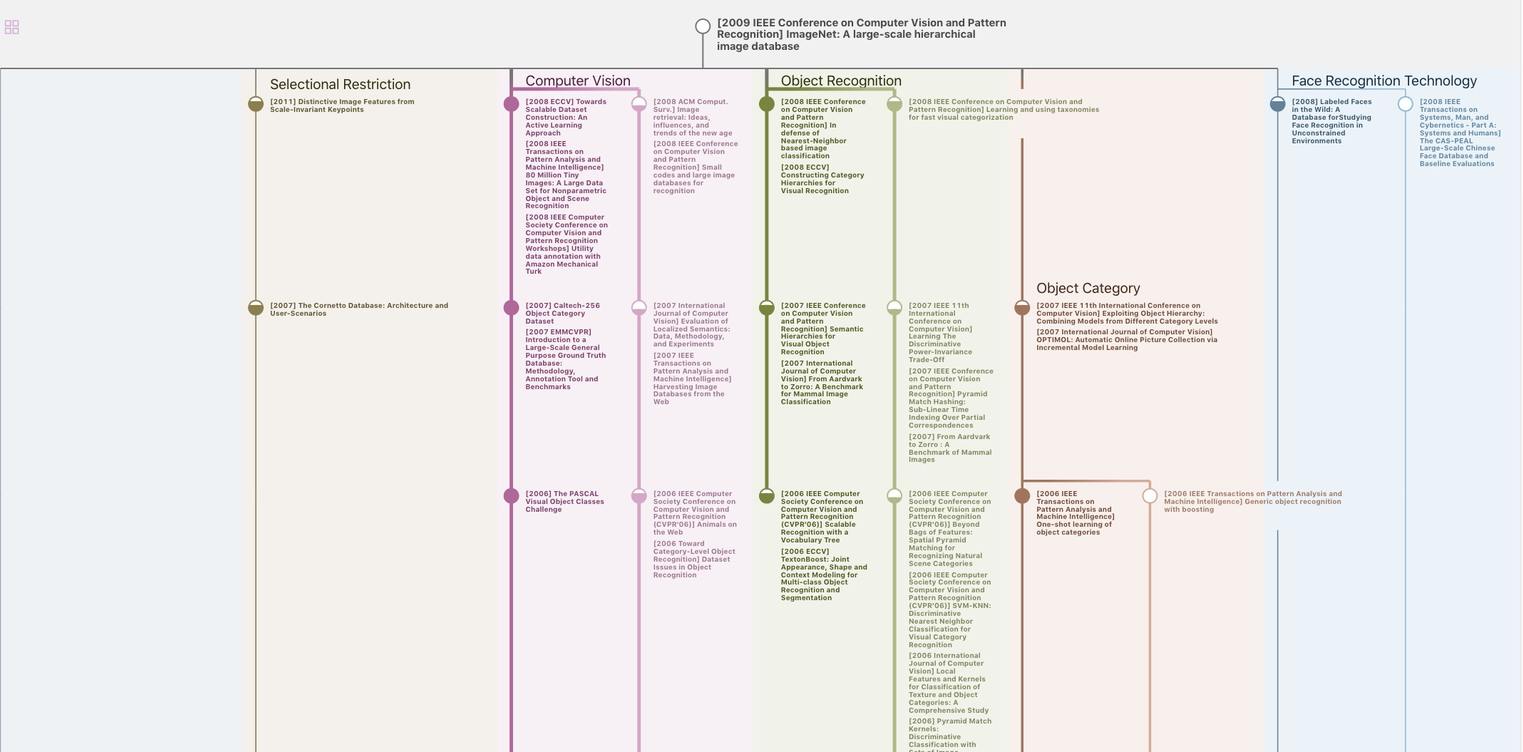
生成溯源树,研究论文发展脉络
Chat Paper
正在生成论文摘要