Activity Detection for Massive Connectivity in Cell-Free Networks With Unknown Large-Scale Fading, Channel Statistics, Noise Variance, and Activity Probability: A Bayesian Approach
IEEE TRANSACTIONS ON SIGNAL PROCESSING(2024)
摘要
Activity detection is an important task in the next generation grant-free multiple access. While there are a number of existing algorithms designed for this purpose, they mostly require precise information about the network, such as large-scale fading coefficients, small-scale fading channel statistics, noise variance at the access points, and user activity probability. Acquiring these information would take a significant overhead and their estimated values might not be accurate. This problem is even more severe in cell-free networks as there are many of these parameters to be acquired. Therefore, this paper sets out to investigate the activity detection problem without the above-mentioned information. In order to handle so many unknown parameters, this paper employs the Bayesian approach, where the unknown variables are endowed with prior distributions which effectively act as regularizations. Together with the likelihood function, a maximum a posteriori (MAP) estimator and a variational inference algorithm are derived. Extensive simulations demonstrate that the proposed methods, even without the knowledge of these system parameters, perform better than existing state-of-the-art methods, such as covariance-based and approximate message passing methods.
更多查看译文
关键词
Bayesian,activity detection,cell-free,massive machine-type communications,grant-free random access
AI 理解论文
溯源树
样例
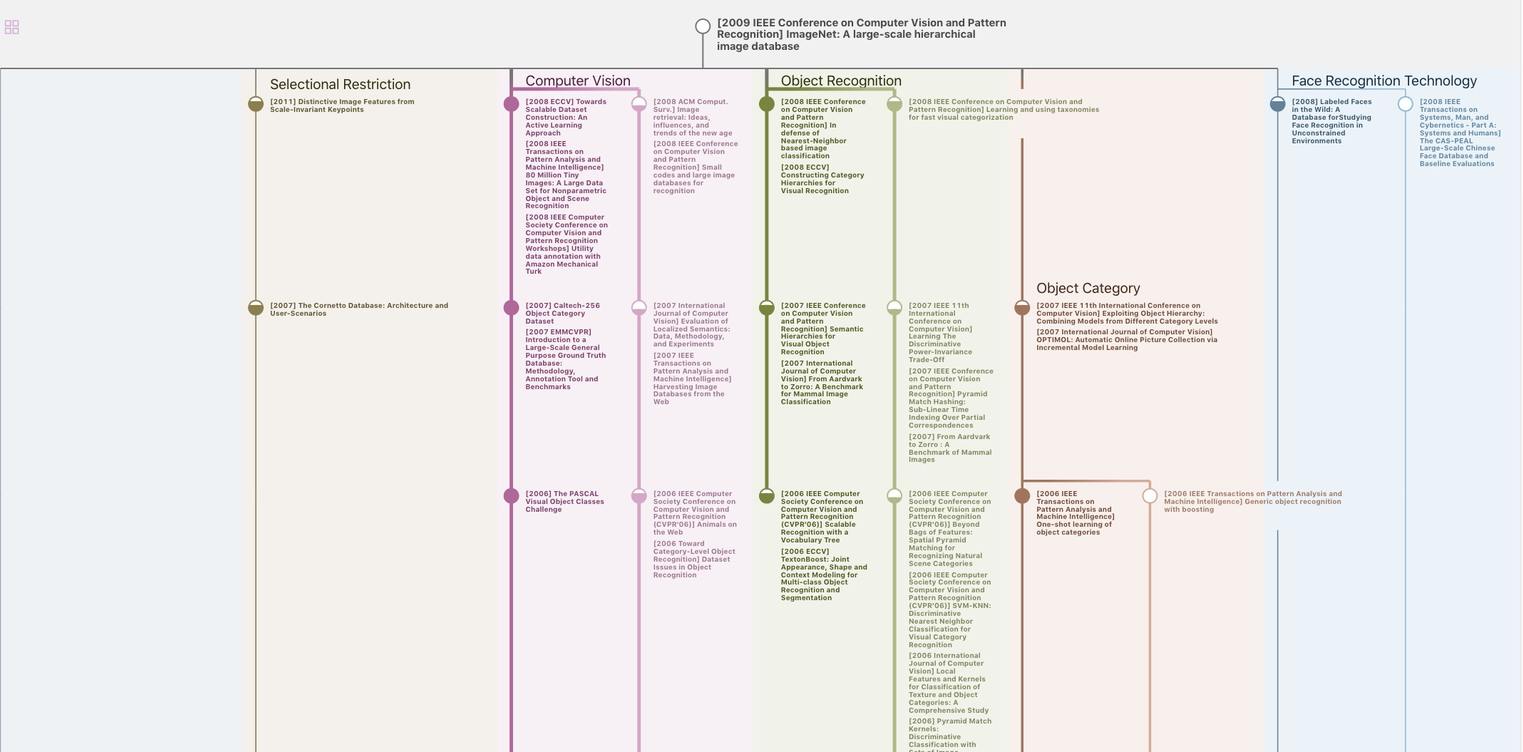
生成溯源树,研究论文发展脉络
Chat Paper
正在生成论文摘要