Video Self-Supervised Cross-Pathway Training Based on Slow and Fast Pathways.
2023 IEEE International Conference on Systems, Man, and Cybernetics (SMC)(2023)
摘要
In the field of video self-supervised learning, contrastive instance learning methods suffer from a lack of semantic information, resulting in inadequate generalization in downstream tasks. Although optical flow can provide some semantic information, it requires significant computational cost prior to training. To address this, we propose a Video self-supervised Cross-pathway training model based on Slow and Fast pathways (VCSF). This model separately extracts temporal and spatial features from pure RGB video frames, and uses the complementary representations of the two pathways to conduct cross-pathway training. Additionally, we propose a motion perception module in the low-frame-rate space to enhance the network's ability to perceive rapidly changing human motion. We conducted extensive experiments in downstream missions of UCF101 and HMDB51, and obtained state-of-the-art results in models using the UCF101 data set for self-supervised pre-training, including motion recognition and nearest neighbor retrieval.
更多查看译文
关键词
Self-supervised learning,Cross-pathway training,Slow and Fast pathways
AI 理解论文
溯源树
样例
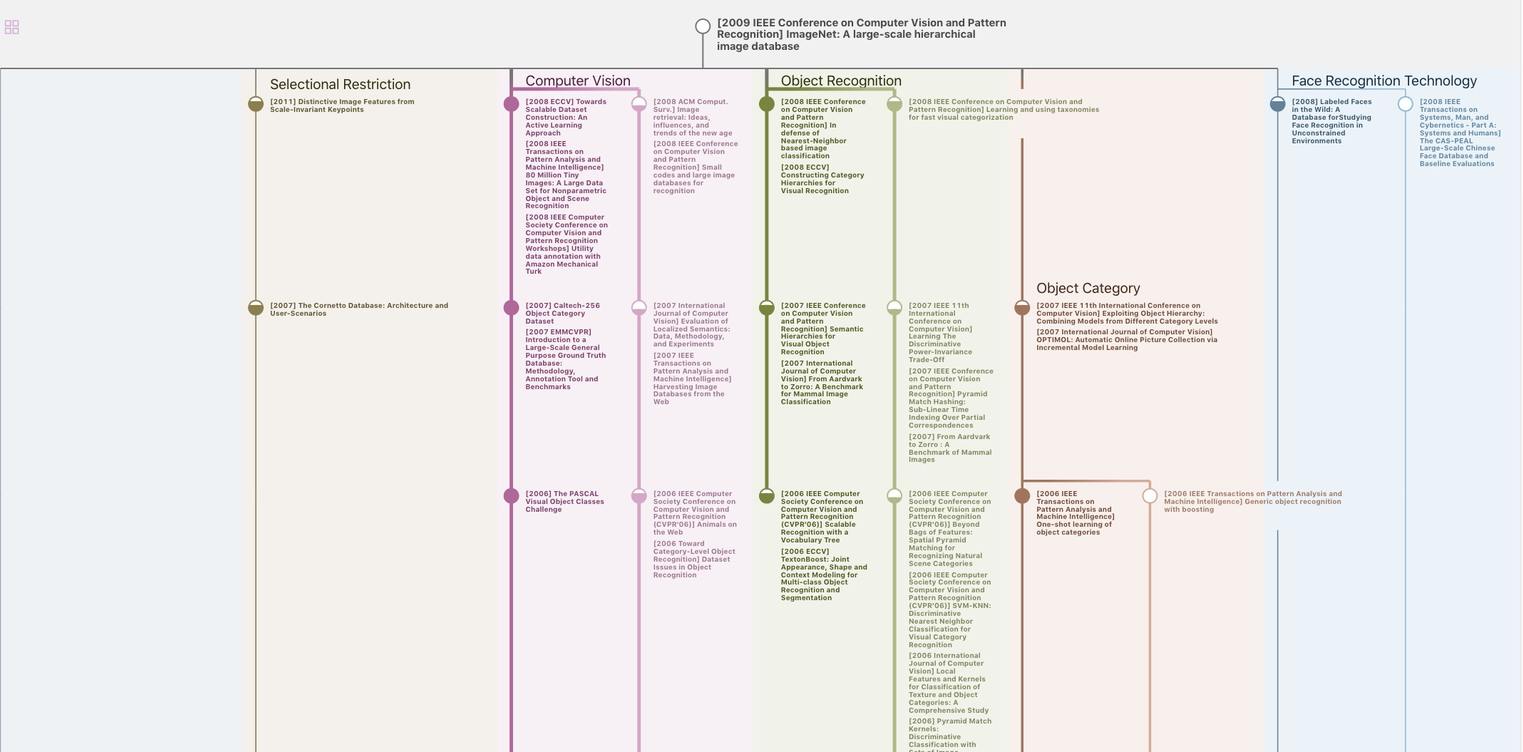
生成溯源树,研究论文发展脉络
Chat Paper
正在生成论文摘要