Combining Self-Organizing Map with Reinforcement Learning for Multivariate Time Series Anomaly Detection.
2023 IEEE International Conference on Systems, Man, and Cybernetics (SMC)(2023)
摘要
Anomaly detection plays a critical role in condition monitors to support the trustworthiness of Cyber- Physical Systems (CPS). Detecting multivariate anomalous data in such systems is challenging due to the lack of a complete comprehension of anomalous behaviors and features. This paper proposes a framework to address time series multivariate anomaly detection problems by combining the Self-Organizing Map (SOM) with Deep Reinforcement Learning (DRL). By clustering the multivariate data, SOM creates an environment to enable the DRL agents interacting with the collected system operational data in terms of a tabular dataset. In this environment, Markov chains reveal the likely anomalous features to support the DRL agent exploring and exploiting the state-action space to maximize anomaly detection performance. We use a time series dataset, Skoltech Anomaly Benchmark (SKAB), to evaluate our framework. Compared with the best results by some currently applied methods, our framework improves the F1 score by 9% from 0.67 to 0.73.
更多查看译文
关键词
Time Series,Anomaly Detection,Self-organizing Map,Multivariate Time Series,Markov Chain,F1 Score,Physical System,Multivariate Data,Deep Reinforcement Learning,Cyber-physical Systems,Anomalous Data,Deep Reinforcement Learning Agent,Long Short-term Memory,Transition Probabilities,Generative Adversarial Networks,Input State,Reward Function,Transition Dynamics,Temporal Dependencies,Markov Decision Process,Deep Q-network,Anomaly Detection Methods,State-action Pair,Labeled Data Set,Multivariate Datasets,Gated Recurrent Unit,Nominal Conditions,Variational Autoencoder,Semi-supervised Learning,Anomalous Conditions
AI 理解论文
溯源树
样例
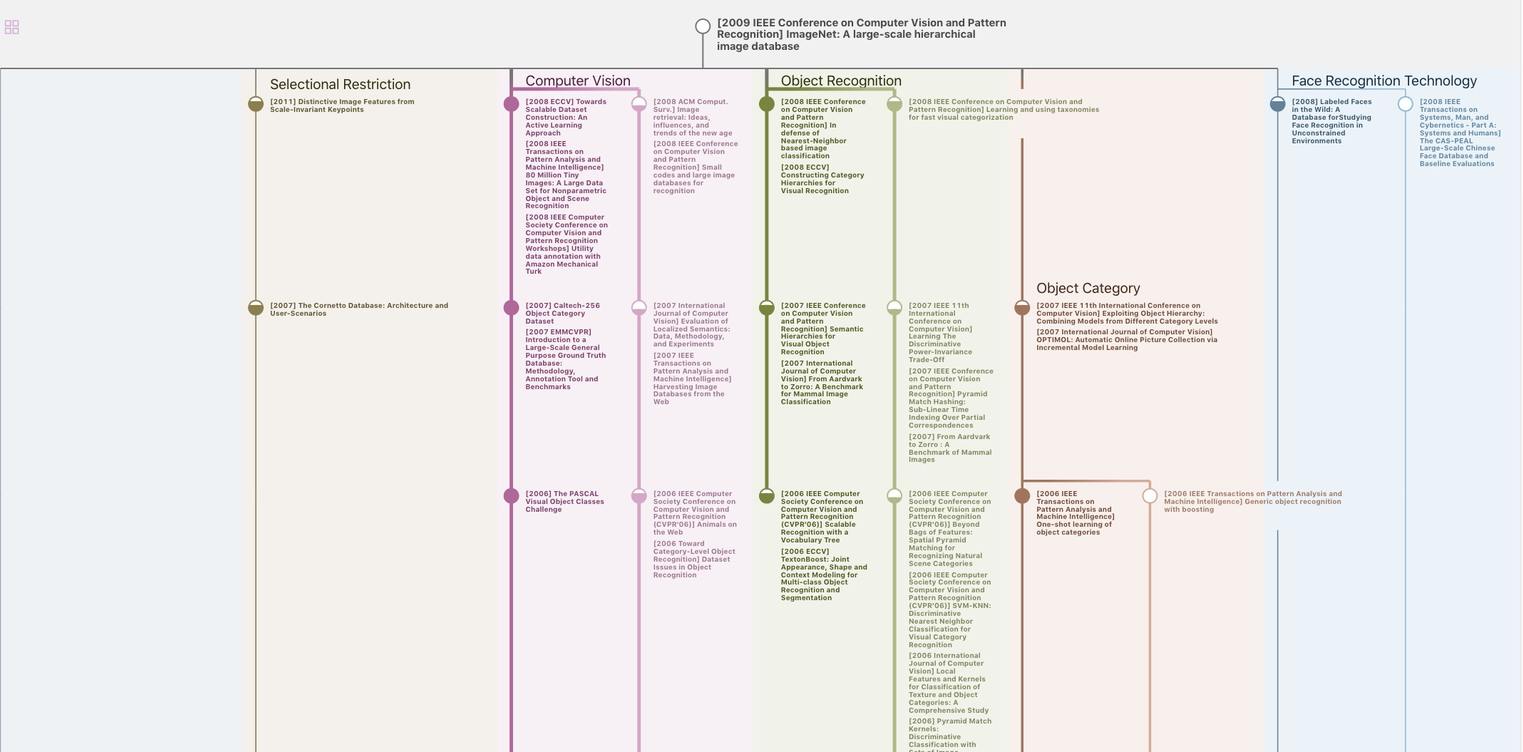
生成溯源树,研究论文发展脉络
Chat Paper
正在生成论文摘要