Latent State Synchronization in Dyadic Partners using EEG.
2023 IEEE International Conference on Systems, Man, and Cybernetics (SMC)(2023)
摘要
Emerging research has shown that interbrain synchronization can occur when individuals interact on a shared task or receive shared stimuli. While the mechanisms producing such effects are not fully understood, interbrain synchronization has been observed in multiple contexts. Analyses of such synchrony, however, are often limited to individual, bottom-up features such as activity at individual cortical sites and frequency bands, or cross-projections of the data into a common space that maximizes correlation. It is an open question whether synchronization can also be observed using more top-down notions of latent state composed of multiple, simultaneous features that are not fit to the individual or task at hand? Here, we investigate whether latent state estimation methods, borrowed from the domain generalization toolboxes of the brain-computer interface community can be used to assess interbrain synchrony. Domain generalization methods learn models of neural activity that transfer from one domain to another without the need for participant- or task-specific training data. We use data from single individuals in a controlled task to train a domain-generalized model to detect latent state changes previously described as relating to alertness or vigilance. We apply the model to a novel, target domain in which teams of two worked together to locate and defuse simulated improvised explosive devices. Our results revealed significantly greater correlation between the latent states of dyadic partners compared to randomly paired individuals, establishing that interbrain synchronization can be observed using top-down methods that are not fit to the individuals, or task, in question.
更多查看译文
关键词
Latent State,Dyad Partner,Changes In Conditions,Training Data,Frequency Band,Neural Activity,Domain Generalization,Explosive Devices,Synchronization Analysis,Improvised Explosive Devices,Training Set,Individual Characteristics,Convolutional Neural Network,EEG Data,Convolutional Neural Network Model,Gameplay,Color Vision,EEG Signals,Changes In Power,Inter-subject Correlation,Time Block,Key Codes,Neural Synchrony,Hz Power,Ensemble Approach,Temporal Filtering,Spectral Changes,Multiple Individuals
AI 理解论文
溯源树
样例
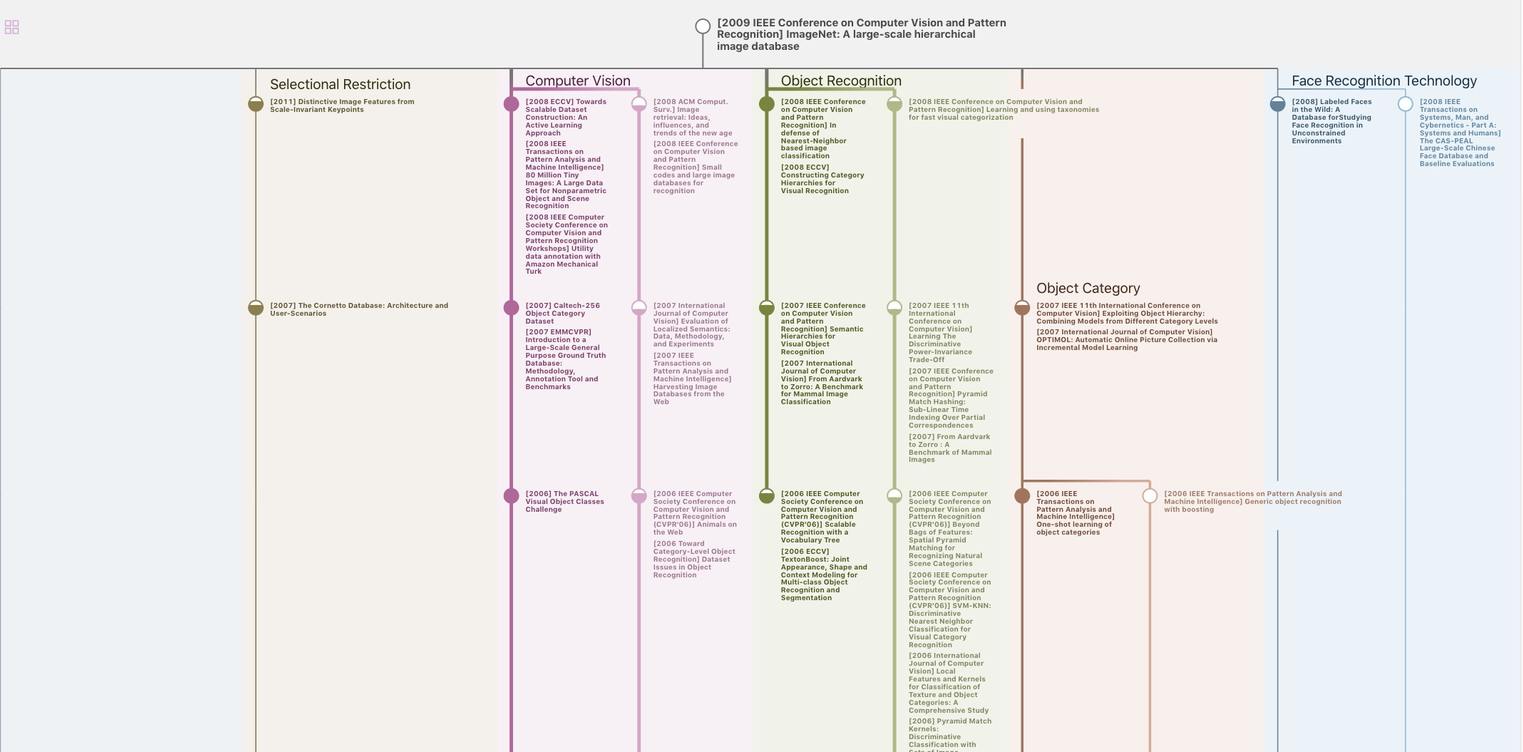
生成溯源树,研究论文发展脉络
Chat Paper
正在生成论文摘要