Spiking Neural Networks for sEMG-Based Hand Gesture Recognition.
2023 IEEE International Conference on Systems, Man, and Cybernetics (SMC)(2023)
摘要
Given the recent surge of significant interest in implementing intelligent hand gesture recognition methods in human-machine interface systems, a wide variety of Deep Neural Networks (DNNs) have been proposed in the literature. In this paper, we introduce a novel and compact Spiking Neural Network (SNN) model for hand gesture recognition using High-Density surface Electromyogram (HD-sEMG) signals. Capitalizing on their ability to extract spatiotemporal features of HD-sEMG signals along with their proven strength in imitating human brain's neural activity using event-driven data processing, we used SNNs as the main building block of our proposed hand gesture recognition model. We show that our proposed model can efficiently differentiate 14 hand movements by considering each sample of the HD-sEMG data as a single time step for the SNN architecture. Moreover, we show that the proposed SNN model does not require huge pre-processing, spike encoding and feature extraction tasks and works effectively on Min-Max normalized continuous-value sEMG signals. We evaluate our SNN model using a 5-fold cross-validation scheme and categorize different participants based on the range of classification accuracy we obtained for them. The following results are acquired by segmenting HD-sEMG signals into windows of size 62.5ms with no overlap. The proposed method led to 6 out of 19 subjects achieving average classification accuracy of ≥ 80% with maximum accuracy of 98% associated with 3
rd
session of the sEMG dataset as the test set.
更多查看译文
关键词
Neural Network,Natural Sciences,Spiking Neural Networks,Hand Gestures,Gesture Recognition,Hand Gesture Recognition,Time Step,Classification Accuracy,Deep Neural Network,Average Accuracy,Hand Movements,5-fold Cross-validation,Recognition Model,Single Time Step,sEMG Signals,Loss Function,Electrode,Signed-rank Test,Support Vector Machine,Image Classification,Dynamic Vision Sensor,Leaky Integrate-and-fire,Output Spike,Final Loss,Training Stage,sEMG Data,Spike Count,Human-machine Interaction,Hybrid Architecture,Linear Discriminant Analysis
AI 理解论文
溯源树
样例
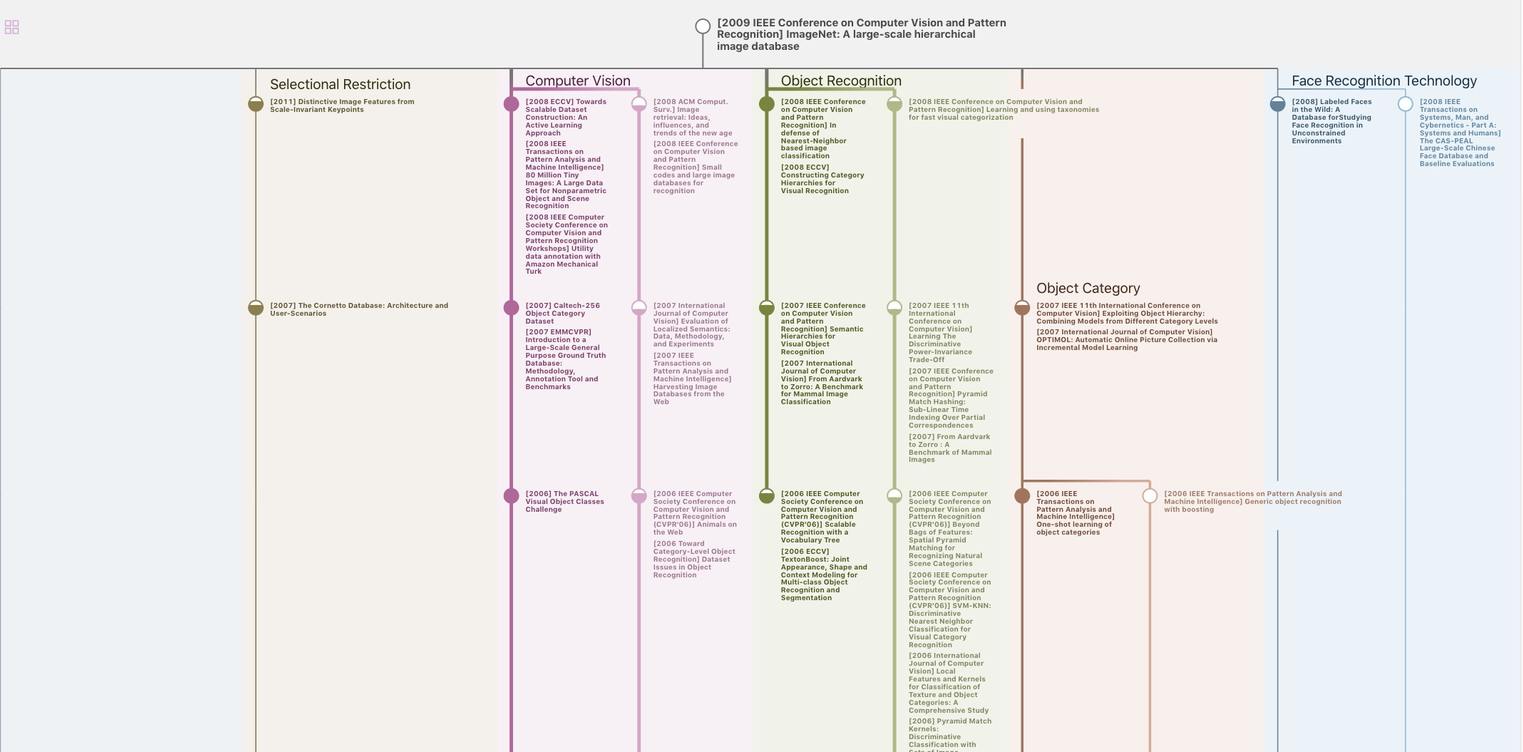
生成溯源树,研究论文发展脉络
Chat Paper
正在生成论文摘要