A discussion of statistical criteria for assessing awareness with SMR BCI after brain injury.
2023 IEEE International Conference on Systems, Man, and Cybernetics (SMC)(2023)
摘要
This work discusses the implications of selecting particular statistical metrics and thresholds as criteria to diagnose awareness through Brain-Computer Interface (BCI) technology in patients with Disorders of Consciousness (DOC). We report a first analysis of a novel dataset collected to investigate whether a motor attempt electroencephalography (EEG) paradigm coupled with Functional Electrical Stimulation (FES) can detect command following and, therefore, signs of conscious awareness in DOC. We assessed 22 DOC patients admitted to the acute rehabilitation unit after a brain lesion over one or more sessions. We extracted EEG sensorimotor rhythms and performed a standard open-loop BCI pipeline evaluation, classifying motor attempt against resting-state trials. We validate this approach by correlating classification accuracy with the established clinical scale Coma Recovery Scale Revised. We employ a machine learning (ML)-inspired diagnostic criterion based on confidence intervals over chance-level classification accuracy and show that it yields more conservative and, arguably, reliable inference of Cognitive Motor Dissociation (CMD) by means of command-following, neuroimaging-based tools, compared to diagnoses based on clinical assessments or criteria examining the statistical significance of brain features across different mental states.
更多查看译文
关键词
disorders of consciousness,awareness,brain-computer interface,motor attempt,chance-level
AI 理解论文
溯源树
样例
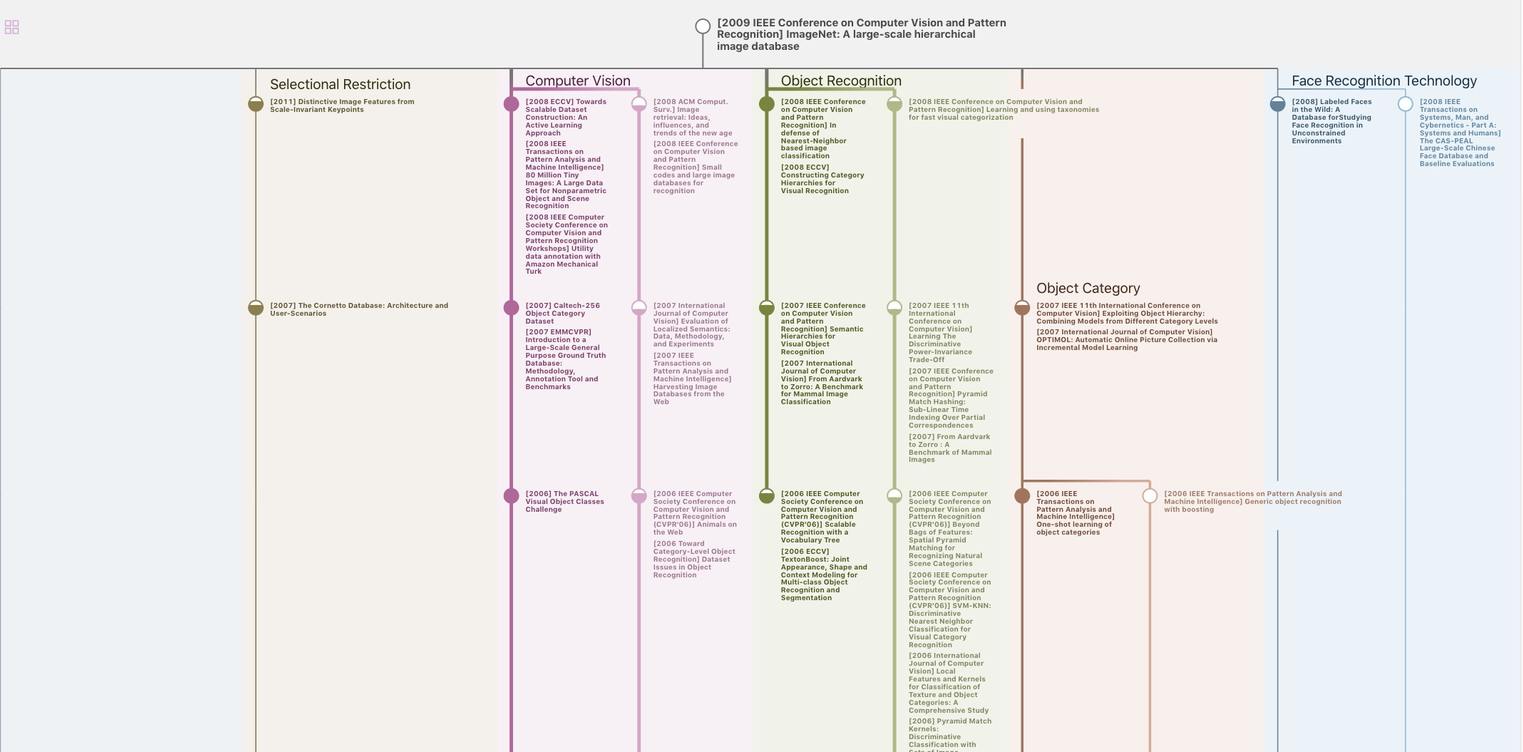
生成溯源树,研究论文发展脉络
Chat Paper
正在生成论文摘要