Exposing Computer-Generated Images Via Amplified Texture Differences Learning.
2023 IEEE International Conference on Systems, Man, and Cybernetics (SMC)(2023)
摘要
Many Computer-Generated (CG) images are spreading widely on the Internet, which may deliberately misinform or deceive the public. Therefore, distinguishing CG images from natural photographic (PG) has become a frontier research topic in the field of image forensics. Although many algorithms have been proposed, it is still very challenging to detect CG images generated by the recent cutting-edge generative methods. Besides, most existing algorithms tend to generalize poorly when facing different unseen multimodal generative models. To address this issue, a novel method based on amplified texture differences learning is proposed to tackle this problem. We first design a deep texture enhancement module for discriminative texture amplification. Specifically, a semantic segmentation module is utilized to generate semantic segmentation map for the affine transformation operation guidance, which can be further used to recover the texture in different regions of the input image. Then, the combination of the original image and the high-frequency components of the original and enhanced images are fed into a hybrid neural network equipped with attention mechanisms, which refines intermediate features and facilitates trace exploration in spatial and channel dimensions respectively. By verifying on several commonly used benchmark datasets and a newly constructed dataset
1
1
The benchmark is available at https://github.com/191578010/DSGCG. with more realistic and diverse images, the experimental results demonstrate that the proposed approach outperforms some existing methods.
更多查看译文
关键词
Differences In Texture,Computer-generated Images,Neural Network,Input Image,Image Regions,Attention Mechanism,Benchmark Datasets,Image Generation,Semantic Segmentation,Affine Transformation,Segmentation Map,Semantic Map,Enhancement Module,Hybrid Neural Network,Convolutional Neural Network,Positive Samples,Convolutional Layers,Feature Maps,Visual Features,Batch Normalization,Generative Adversarial Networks,Photographic Images,Output Feature Map,Realistic Dataset,Attention Module,Residual Block,Spatial Attention,Detection In Images
AI 理解论文
溯源树
样例
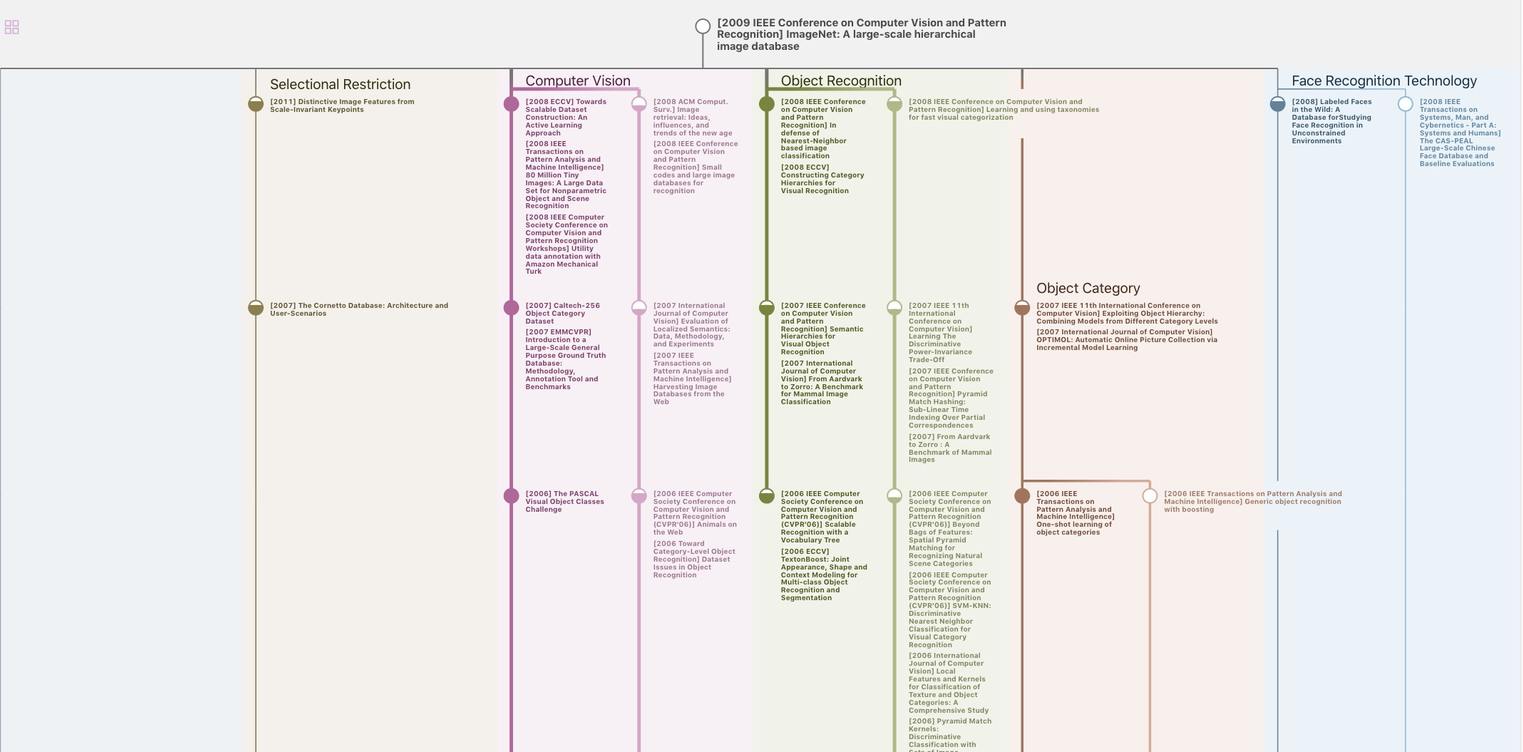
生成溯源树,研究论文发展脉络
Chat Paper
正在生成论文摘要