A Federated Mining Framework for Complete Frequent Itemsets.
2023 IEEE International Conference on Systems, Man, and Cybernetics (SMC)(2023)
摘要
In this paper, we address the common features of horizontal federated learning in data mining and propose a federated mining framework, which adopts a client-server model that cooperates with multiple data-source clients. The proposed algorithm handles client-side mining and server-side aggregation. For client-side mining, the algorithm uses prelarge itemsets to collect additional information for the server to integrate the clients' local mining results. For server-side aggregation, the algorithm considers the characteristics of large and prelarge itemsets sent from the clients and use a boundary strategy for integration. Experiments show that our method acquires the complete mined results while protecting data.
更多查看译文
关键词
federated mining,frequent itemset,prelarge itemset,privacy-preserving
AI 理解论文
溯源树
样例
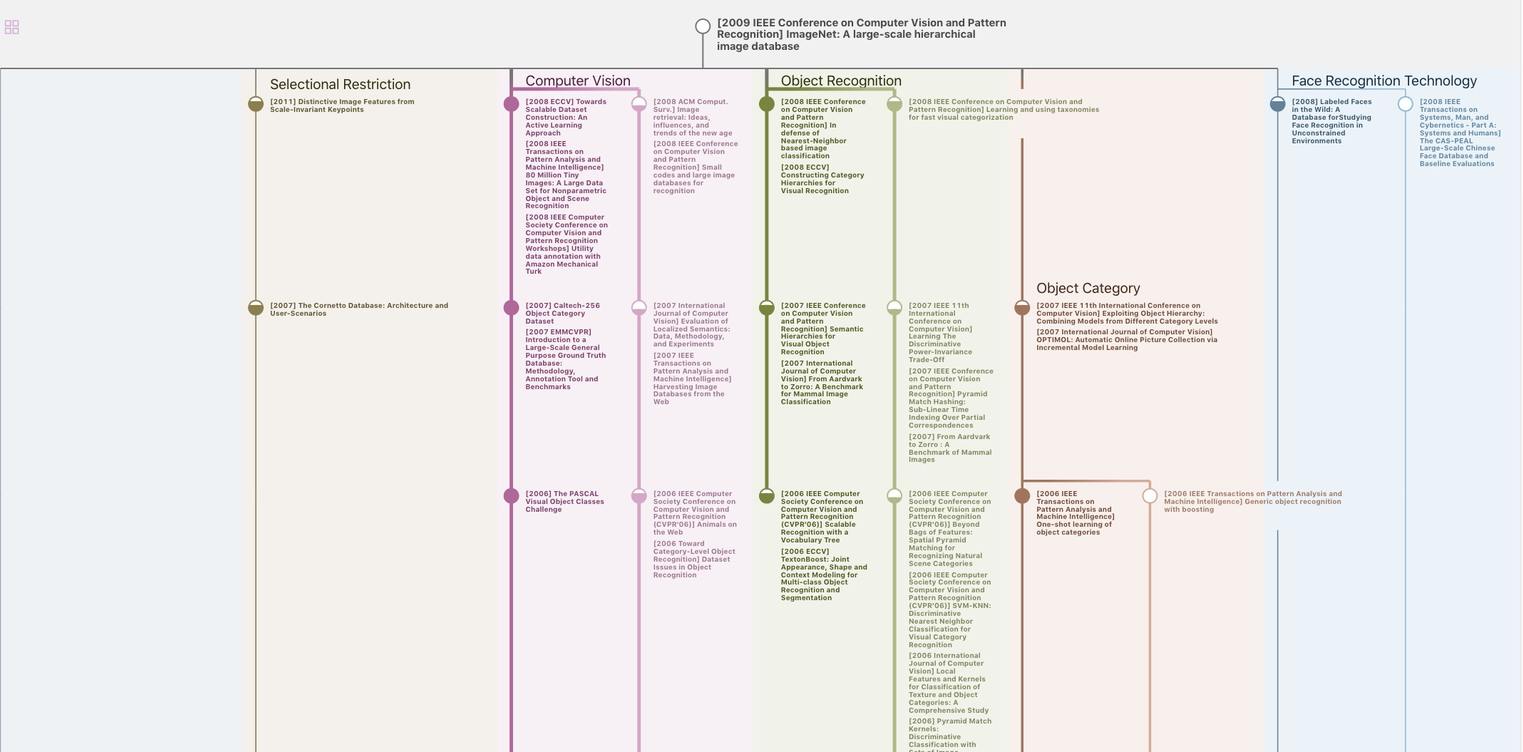
生成溯源树,研究论文发展脉络
Chat Paper
正在生成论文摘要