Clustering and Geodesic Scaling of Dissimilarities on the Spherical Surface
Journal of Agricultural, Biological and Environmental Statistics(2024)
摘要
Spherical embedding is an important tool in several fields of data analysis, including environmental data, spatial statistics, text mining, gene expression analysis, medical research and, in general, areas in which the geodesic distance is a relevant factor. Many data acquisition technologies are related to massive data acquisition, and these high-dimensional vectors are often normalised and transformed into spherical data. In this representation of data on spherical surfaces, multidimensional scaling plays an important role. Traditionally, the methods of clustering and representation have been combined, since the precision of the representation tends to decrease when a large number of objects are involved, which makes interpretation difficult. In this paper, we present a model that partitions objects into classes while simultaneously representing the cluster centres on a spherical surface based on geodesic distances. The model combines a partition algorithm based on the approximation of dissimilarities to geodesic distances with a representation procedure for geodesic distances. In this process, the dissimilarities are transformed in order to optimise the radius of the sphere. The efficiency of the procedure described is analysed by means of an extensive Monte Carlo experiment, and its usefulness is illustrated for real data sets. Supplementary material to this paper is provided online.
更多查看译文
关键词
Multidimensional scaling,Dissimilarity,Geodesic distance,Cluster analysis,Spherical surface
AI 理解论文
溯源树
样例
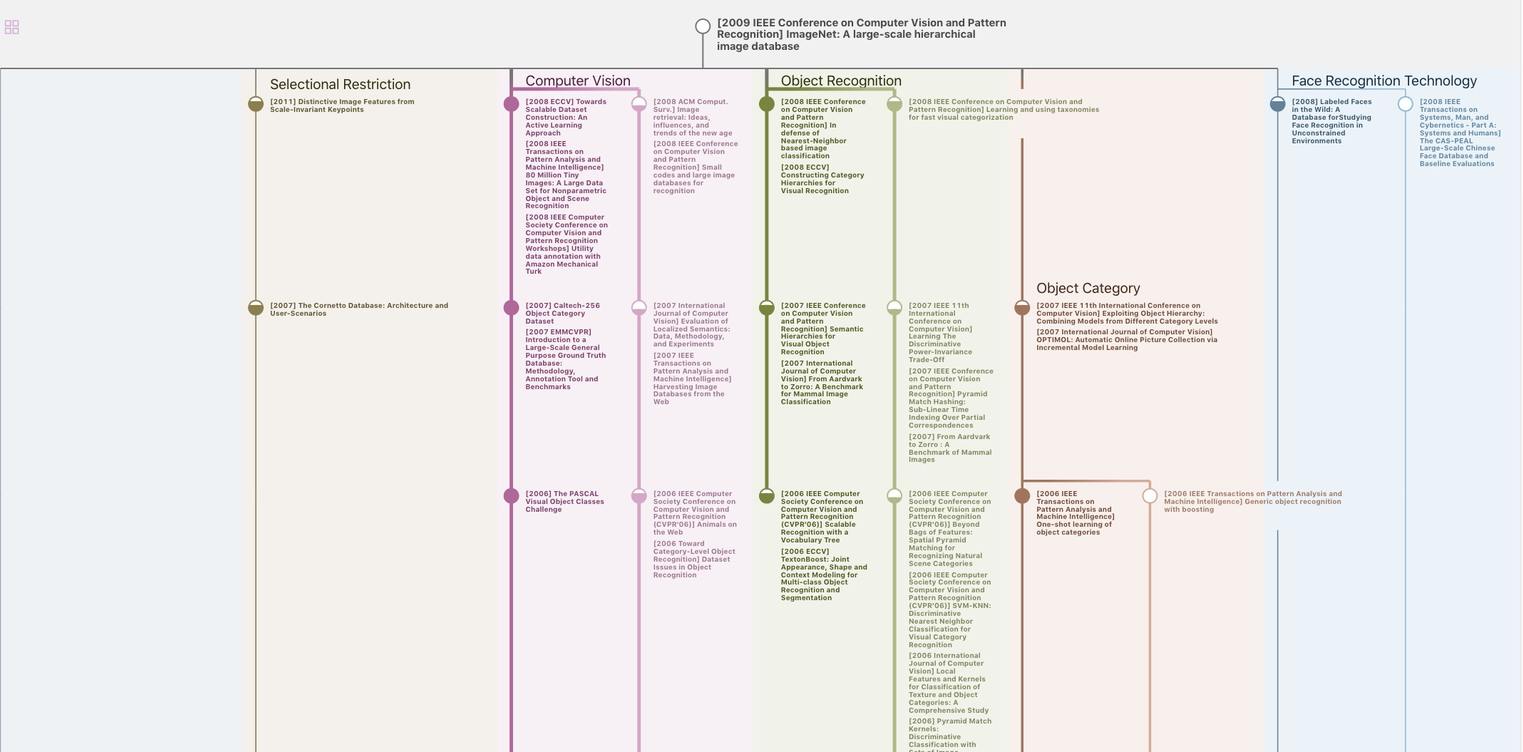
生成溯源树,研究论文发展脉络
Chat Paper
正在生成论文摘要