Prediction of Stroke Risk Within 7-Years Follow Up Using Machine Learning Models*
2023 IEEE EMBS Special Topic Conference on Data Science and Engineering in Healthcare, Medicine and Biology(2023)
摘要
A stroke, also known as brain attack, occurs when blood supply to your brain is interrupted. Primary prevention relies on prompt prediction of a stroke. While currently there are several clinical risk scores, machine learning (ML) models seems to be more suitable tools for accurate prediction of stroke events. Therefore, this work focuses on the prediction of stroke within 7 years follow-up in patients who have not suffered from a stroke or TIA event at baseline. LightGBM (LGBM), Extreme Grading Boosting (XGBoost), Support Vector Machine (SVM) and Decision Tree were employed in the getABI dataset, which includes 5,897 participants. The performance of models was calculated by Accuracy (ACC), Sensitivity (SENS), Specificity (SPE) and area under the receiver operating characteristic curve (AUC) of each model. According to the comparison analysis’s results, LGBM has been shown to be the most trustworthy algorithm, with accuracy 68 %. Moreover, sex, age, status of peripheral artery disease (PAD), history of myocardial infarction, angina pectoris, amputation and diabetes and pulse status of different arteries can be used as a simple and cost-effective way to predict stroke.Clinical Relevance: A fatal medical emergency, stroke may be anticipated using artificial intelligence, and the sooner it is predicted, the more cerebrovascular disease occurrences can be avoided.
更多查看译文
关键词
Machine Learning Models,Risk Of Stroke,Model Performance,Support Vector Machine,Decision Tree,Follow-up Of Patients,Amputation,Transient Ischemic Attack,Peripheral Artery Disease,Stroke Events,XGBoost,History Of Myocardial Infarction,Stroke Brain,Sensitivity,Clinical Risk Score,Predictor Of Stroke,LightGBM,Diastolic Blood Pressure,Artificial Neural Network,Laboratory Data,Classification Of Patients,History Of Comorbidities
AI 理解论文
溯源树
样例
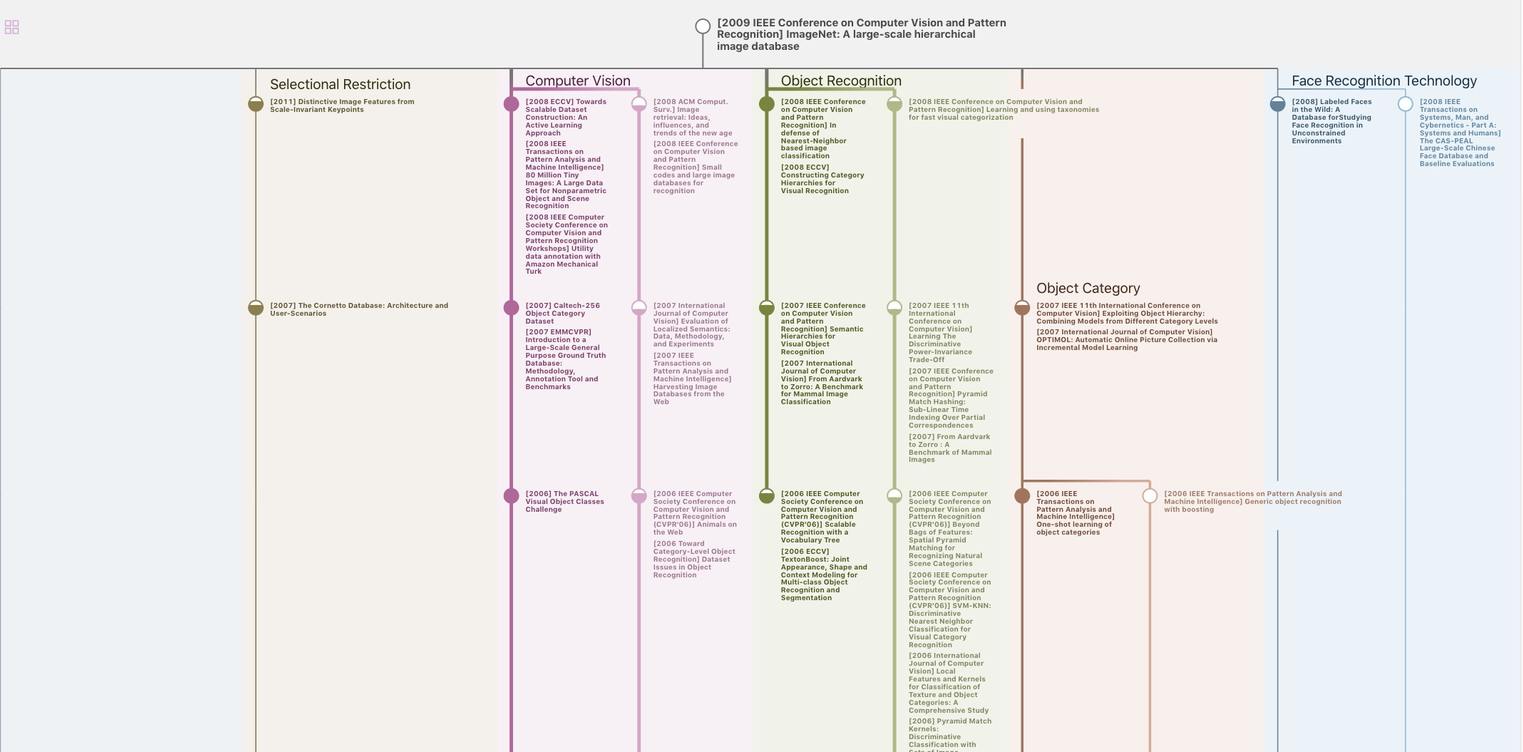
生成溯源树,研究论文发展脉络
Chat Paper
正在生成论文摘要