Atrial Fibrillation Diagnosis Using Machine Learning: Leveraging Minimal Health Data from UK Biobank
2023 IEEE EMBS Special Topic Conference on Data Science and Engineering in Healthcare, Medicine and Biology(2023)
摘要
This study aims to develop a machine learning model to diagnose atrial fibrillation(AF) using only medical information that can be obtained during routine care or medical examinations. We obtained the age, sex, body mass index, and presence of hypertension, stroke, or coronary artery disease of a total of 404,898 subjects, including 6,661 AF patients, from UK Biobank data and developed and validated an XGBoost-based model to diagnose AF. The developed model showed an average AUROC of 0.785 for diagnosing AF, with the following factors having the greatest impact on the results, in this order: age, sex, hypertension, body mass index, coronary artery disease, and stroke.Clinical Relevance: A model that diagnoses AF based on simple demographic and disease status can screen suspected AF early from routine healthcare without dedicated tests.
更多查看译文
关键词
Atrial Fibrillation,Diagnosis Of Atrial Fibrillation,Body Mass Index,Coronary Artery Disease,Atrial Fibrillation Patients,Demographic Data,Genetic Information,XGBoost Model,SHapley Additive exPlanations,SHapley Additive exPlanations Values,Atrial Fibrillation Group
AI 理解论文
溯源树
样例
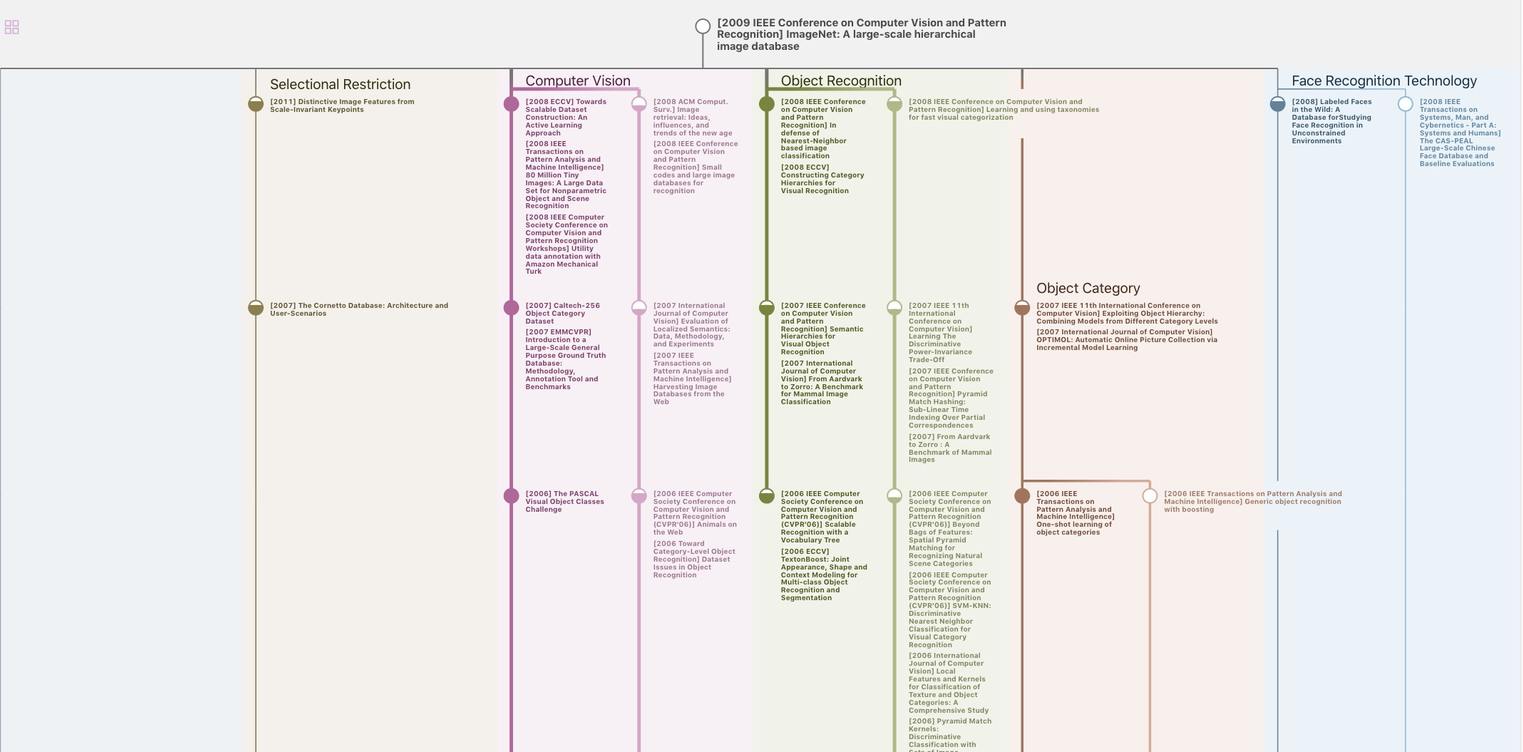
生成溯源树,研究论文发展脉络
Chat Paper
正在生成论文摘要