Deep Learning-Based Sample Misidentification Error Detection in Clinical Chemistry Test
2023 IEEE EMBS Special Topic Conference on Data Science and Engineering in Healthcare, Medicine and Biology(2023)
摘要
We developed a deep neural network (DNN) model to detect sample misidentification error using previous and current clinical chemistry test results as input and compared the detection performance with conventional methods such as DPC and absDPC. DNN models were developed to detect sample misidentification errors for each of the five tumor markers; AFP, CA19-9, CA125, CEA, and PSA. As a result of 1,000 times of repeated simulations of 1% random sample misidentification test, the DNN model achieved an AUC of ≥0.802. Moreover, the accuracy of DNN model was ≥0.732, which is higher than the DPC (≥0.646) and absDPC (≥0.509).Clinical Relevance: The DNN model more effectively detects sample mix errors in tumor markers than the conventional delta check methods.
更多查看译文
关键词
Clinical Testing,Clinical Chemistry Tests,Deep Neural Network,Detection Performance,Prostate-specific Antigen,Deep Neural Network Model,Cancer Antigen 125,Deep Learning,South Korea,Adaptive Moment Estimation
AI 理解论文
溯源树
样例
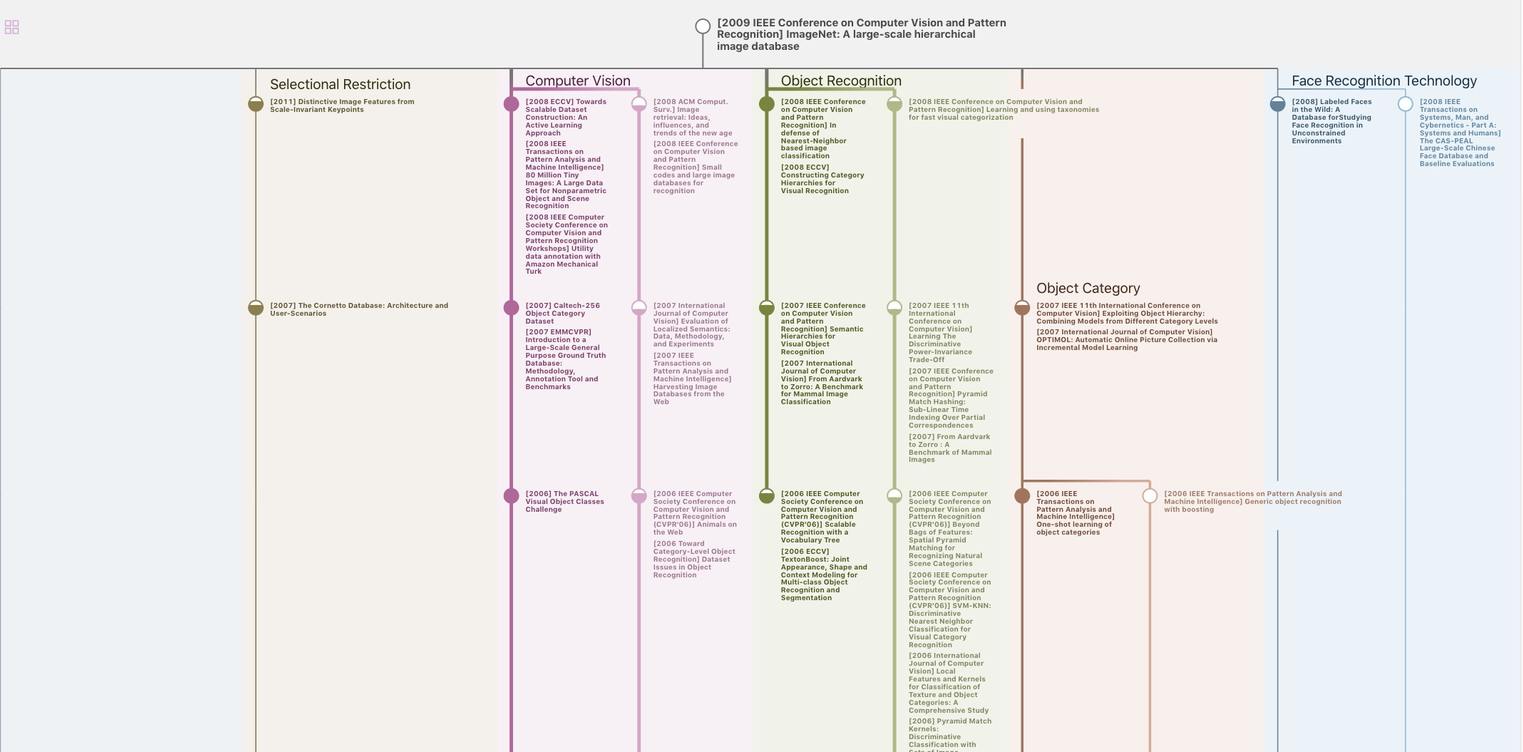
生成溯源树,研究论文发展脉络
Chat Paper
正在生成论文摘要