Adaptive Local Feature Matching for Few-shot Fine-grained Image Recognition.
2023 International Conference on Digital Image Computing: Techniques and Applications (DICTA)(2023)
摘要
Few-shot fine-grained image recognition aims to recognize fine categories with subtle differences, given only a few labeled examples. Existing methods try to mine the discriminative local regions to do fine-grained image recognition but still suffer from large variations of the same semantic object and noisy background disturbance. To this end, we propose an adaptive local feature matching network to do few-shot fine-grained image recognition, which matches local features between the support and query images adaptively according to their belonged semantics. Specifically, an Adaptive Thresholding Module (ATM) is proposed to automatically depress the irrelevant and noisy background regions for enlarging inter-class differences. Then a Local Feature Matching Module (LFM) is used for learning consistent local features of the same class. We conduct extensive experiments on three benchmark datasets, CUB-200-2011, Stanford Dogs, and Stanford Cars. The results illustrate the effectiveness and superiority of our proposed method.
更多查看译文
关键词
Few-shot learning,fine-grained recognition,local feature matching
AI 理解论文
溯源树
样例
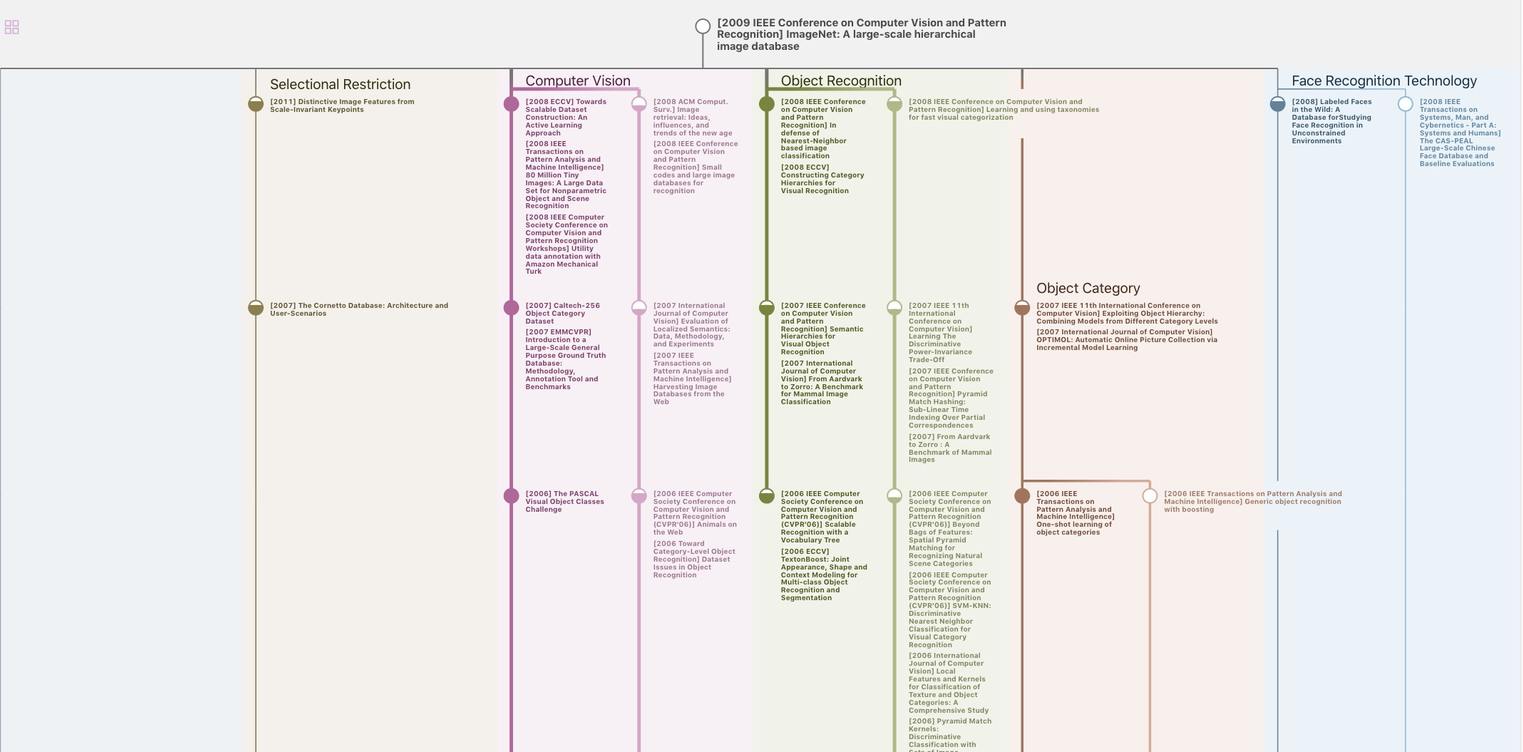
生成溯源树,研究论文发展脉络
Chat Paper
正在生成论文摘要