Ball Detection for a Lightweight Mobile Platform.
2023 International Conference on Digital Image Computing: Techniques and Applications (DICTA)(2023)
摘要
While convolutional neural networks have excelled in recent years in the domain of object detection, many of the benchmark datasets focus on large objects in static frames and neglect the importance of small moving object detection. Furthermore, most state-of-the-art detectors are comprised of many millions of parameters, making them too large to be efficiently deployed onto a computationally constrained mobile platform. To address the challenge of detecting small moving targets from a robotic platform, we introduce a lightweight temporally-aware ball tracker, with a reduced computational footprint and enhanced motion understanding. To better understand how different model variants utilise motion, we analyse the detection performance of different architectures under varying forms of motion information and find that multiple-input-multiple-output models learn to favour motion information in sequences more strongly than multiple-input-single-output models.
更多查看译文
关键词
n/a
AI 理解论文
溯源树
样例
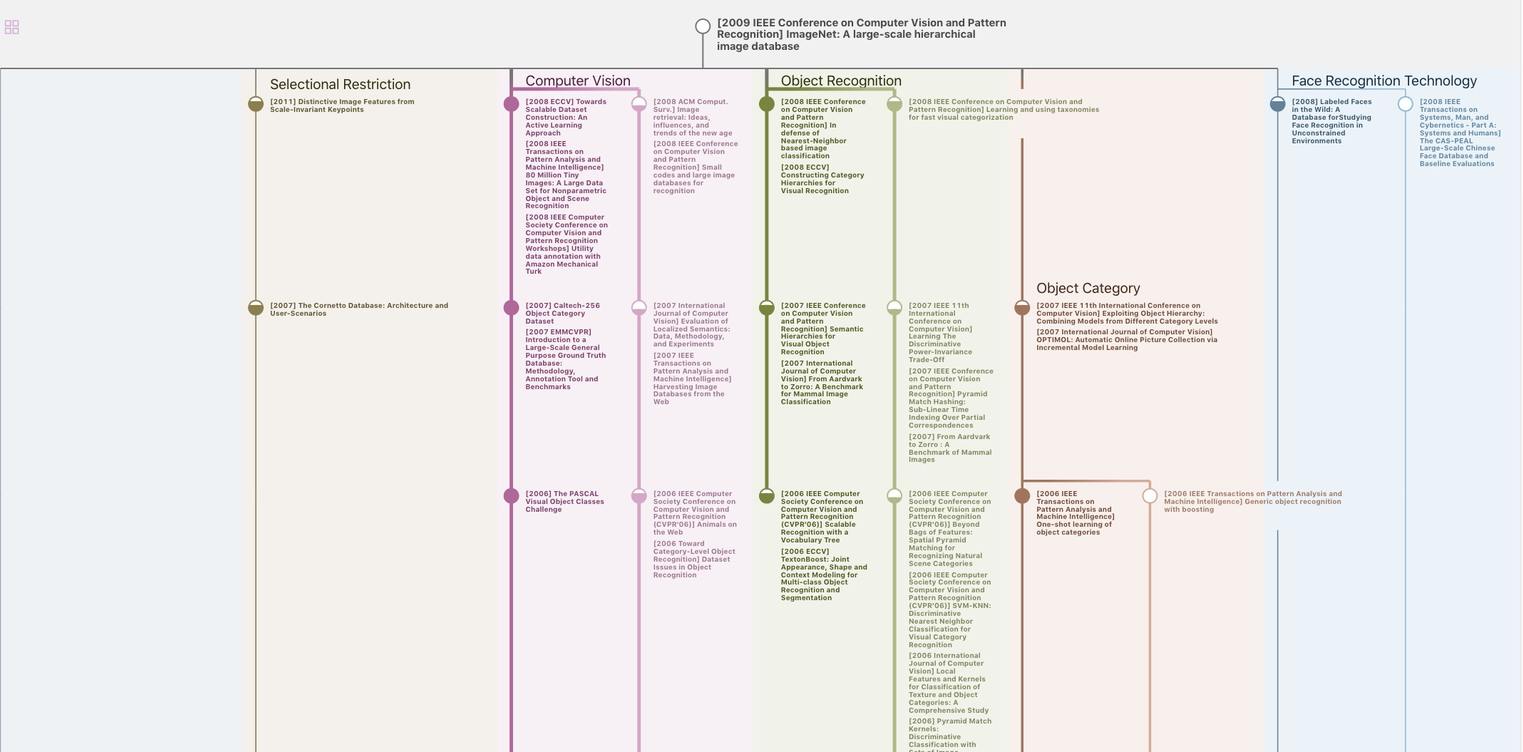
生成溯源树,研究论文发展脉络
Chat Paper
正在生成论文摘要