CNN-based Boreal Peatland Fertility Classification from Sentinel-1 and Sentinel-2 Imagery.
International Symposium on Robotic and Sensors Environments(2023)
摘要
Peatland semantic segmentation is a sub-problem of land cover classification conducted to provide valuable information for environmental monitoring and land management. In this paper, we propose a Convolutional Neural Network (CNN) to fuse two open-source satellite input image sources, Sentinel-1 SAR and optical Sentinel-2, for pixel-level classification of peatland fertility classes. To overcome the overfitting problem and reduce the time and computation complexity, we proposed a window-based approach which consider the defined spatial resolutions around each pixel to perform classification. Two early and late fusion architectures combining inputs at different stages in the network are evaluated. The multi-modal architectures are compared with uni-modal architecture which only uses one input data for training the CNN. Moreover, we investigate how the performance of CNN changes when it is trained with a high number of bands or only the best bands of each data source. The experimental results are acquired on a study area in Finland which cover a variety minerotrophic aapa mire peatlands. They show that our late fusion architecture able to classify peatland fertility levels around 56.73% total accuracy in the study area when the CNN is trained based on the best bands of both optical data and radar signals.
更多查看译文
关键词
convolutional neural network,land cover classification,semantic segmentation,remote sensing
AI 理解论文
溯源树
样例
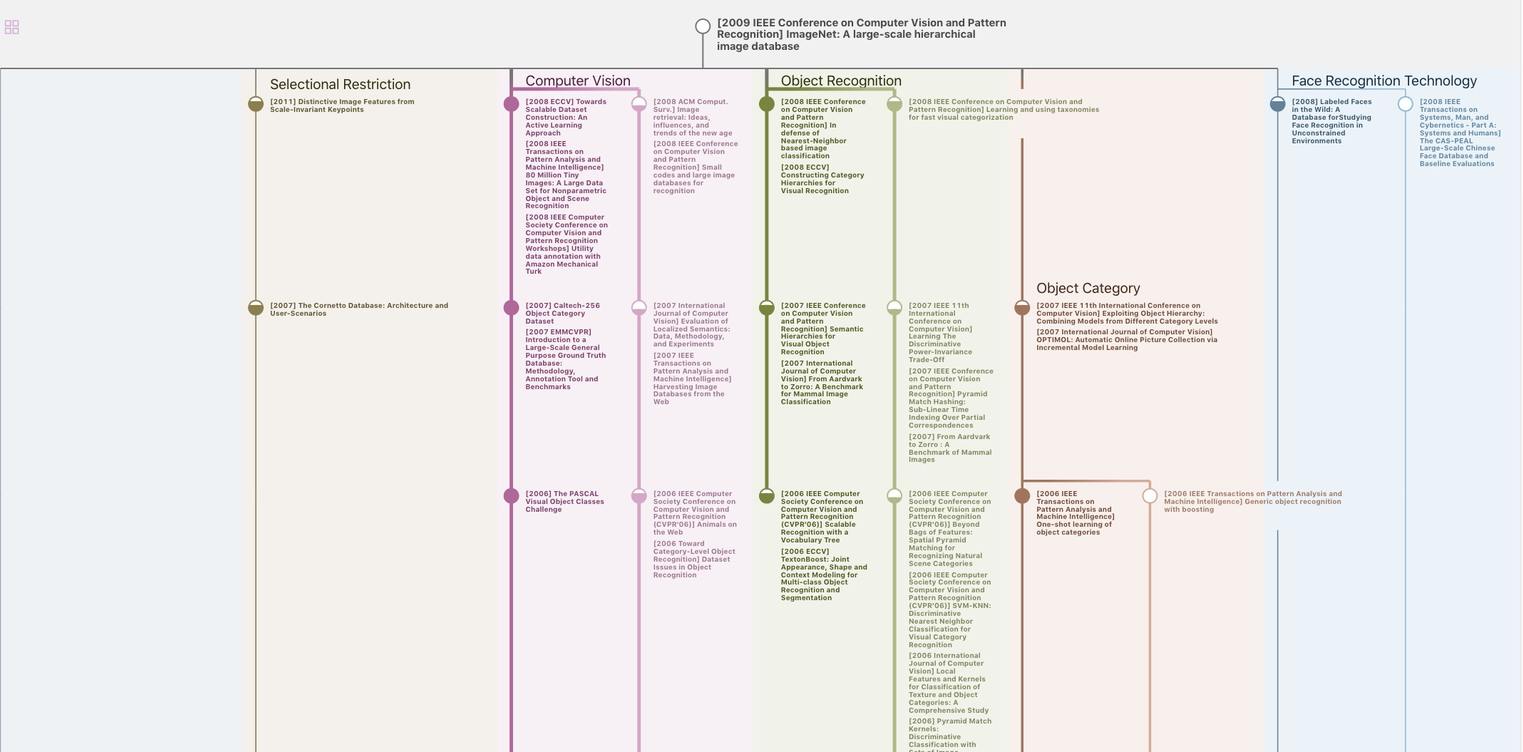
生成溯源树,研究论文发展脉络
Chat Paper
正在生成论文摘要