Generalized Zero-Shot Learning via Implicit Attribute Composition.
2023 IEEE International Conference on Systems, Man, and Cybernetics (SMC)(2023)
摘要
Zero-shot learning (ZSL) is an important but challenging task in computer vision that aims to identify unseen classes without matching training samples. Current cutting-edge ZSL methods based on locality focus on acquiring the explicit locality of distinguishing characteristics, which could face a lack of adequate supervision at the class attribute level. This paper introduces a novel approach called IAC, which aims to learn Implicit Attribute Composition for ZSL. This method is more comprehensive compared to attribute localization that solely focuses on class-level attribute supervision. IAC utilizes subspace representations that efficiently capture the inherent structure of high-dimensional image features. Then, we learn implicit attribute composition through subspace representation learning. The superiority of the proposed IAC compared to the state-of-the-art is demonstrated through sufficient experiments conducted on three commonly used ZSL datasets, CUB, SUN, and AwA2.
更多查看译文
关键词
Zero-shot,Generalized Zero-shot Learning,Image Features,High-dimensional Feature,Lack Of Supervision,Unseen Classes,Subspace Representation,Visual Features,Global Features,Training Methods,Singular Value Decomposition,Generative Adversarial Networks,Latent Space,Harmonic Mean,Learning Spaces,Common Space,Nearest Neighbor Search,Variational Autoencoder,Visual Space,Vector Of Attributes,Orthogonal Basis,Image Encoder,Semantic Space,Unseen Images,Balance Parameters,Global Image Features,Objective Function,Semantic Vectors,Human Gaze
AI 理解论文
溯源树
样例
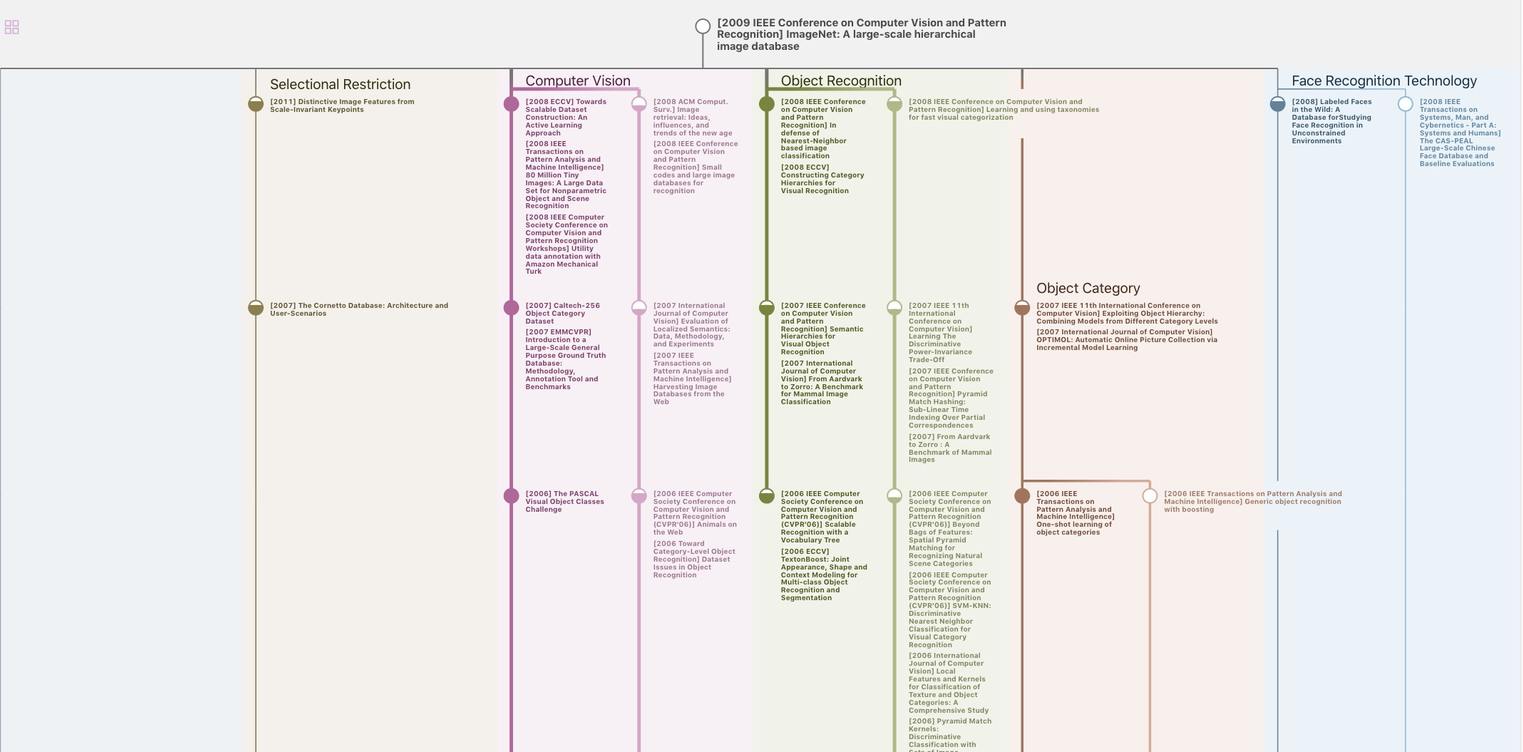
生成溯源树,研究论文发展脉络
Chat Paper
正在生成论文摘要