Local-Adaptive Transformer for Multivariate Time Series Anomaly Detection and Diagnosis.
2023 IEEE International Conference on Systems, Man, and Cybernetics (SMC)(2023)
摘要
Time series data are pervasive in varied real-world applications, and accurately identifying anomalies in time series is of great importance. Many current methods are insufficient to model long-term dependence, whereas some anomalies can be only identified through long temporal contextual information. This may finally lead to disastrous outcomes due to false negatives of these anomalies. Prior arts employ Transformers (i.e., a neural network architecture that has powerful capability in modeling long-term dependence and global association) to alleviate this problem; however, Transformers are insensitive in sensing local context, which may neglect subtle anomalies. Therefore, in this paper, we propose a local-adaptive Transformer based on cross-correlation for time series anomaly detection, which unifies both global and local information to capture comprehensive time series patterns. Specifically, we devise a cross-correlation mechanism by employing causal convolution to adaptively capture local pattern variation, offering diverse local information into the long-term temporal learning process. Furthermore, a novel optimization objective is utilized to jointly optimize reconstruction of the entire time series and matrix derived from cross-correlation mechanism, which prevents the cross-correlation from becoming trivial in the training phase. The generated cross-correlation matrix reveals underlying interactions between dimensions of multivariate time series, which provides valuable insights into anomaly diagnosis. Extensive experiments on six real-world datasets demonstrate that our model outperforms state-of-the-art competing methods and achieves 6.8%-27.5%
$F_{1}$
score improvement. Our method also has good anomaly interpretability and is effective for anomaly diagnosis.
更多查看译文
关键词
Time Series,Anomaly Detection,Multivariate Time Series,Development Program Of China,Time Series Anomaly,Neural Network,Local Information,Local Context,Time Series Data,Local Patterns,Training Phase,Real-world Datasets,Joint Optimization,Cross-correlation Matrix,Window Size,Fast Fourier Transform,Long Short-term Memory,Recurrent Neural Network,Autoencoder,Kullback-Leibler,Graph Neural Networks,Real-world Systems,Traditional Transformation,Multivariate Time Series Data,Time Series Properties,Local Anomalies,Self-attention Mechanism,Embedding Vectors,Anomaly Score,Convolution Kernel Size
AI 理解论文
溯源树
样例
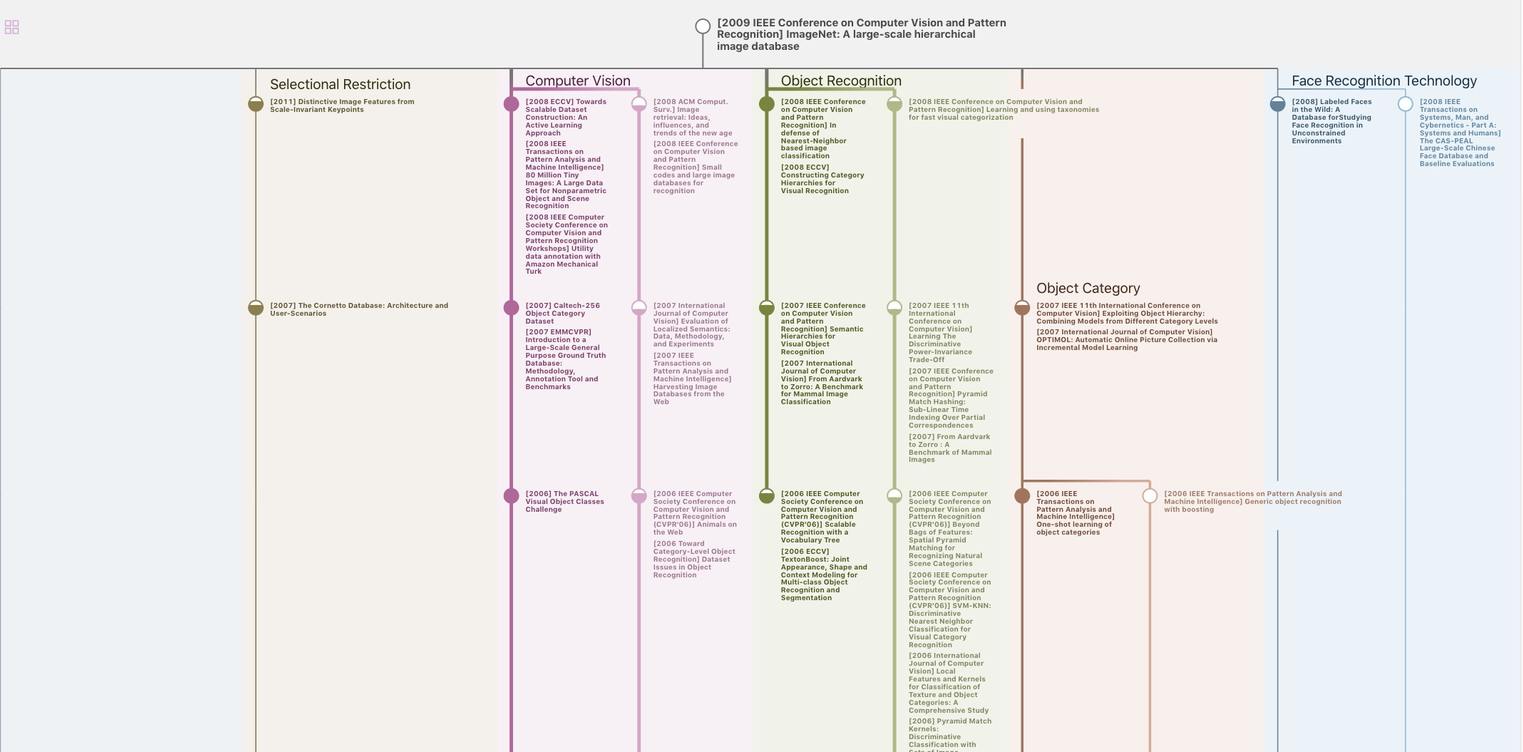
生成溯源树,研究论文发展脉络
Chat Paper
正在生成论文摘要