An Advanced Multi-Scale Feature Compression using Selective Learning Strategy for Video Coding for Machines.
2023 IEEE International Conference on Visual Communications and Image Processing (VCIP)(2023)
摘要
Machine vision-based applications have witnessed widespread adoption in diverse fields. Efficiently processing and compressing the vast amounts of video data collected by machines is crucial for these applications. To address this need, the Moving Picture Experts Group (MPEG) is developing a new' video coding standard known as Video Coding for Machines (VCM), specifically optimized for video consumed by machines in vision applications. This paper proposes an advanced multi-scale feature compression (advMSFC) method with a selective learning strategy (SLS) for the Feature Compression for VCM (FCVCM). By applying the SLS, the proposed method converts multi-scale features into a single-scale feature arranged based on channel-wise importance, enabling adaptive feature channel truncation based on the QP. The truncated feature is efficiently compressed using the latest video codec, Versatile Video Coding (VVC). The proposed method outperforms the VCM feature anchor in instance segmentation, object detection, and object tracking tasks, achieving significant Bjontegaard delta-rate (BD-rate) gains. The adaptability of our method using a single trained model for various QPs shows promise for efficient feature compression in machine vision applications.
更多查看译文
关键词
Machine vision,multi-scale feature compression,selective learning,video coding for machines
AI 理解论文
溯源树
样例
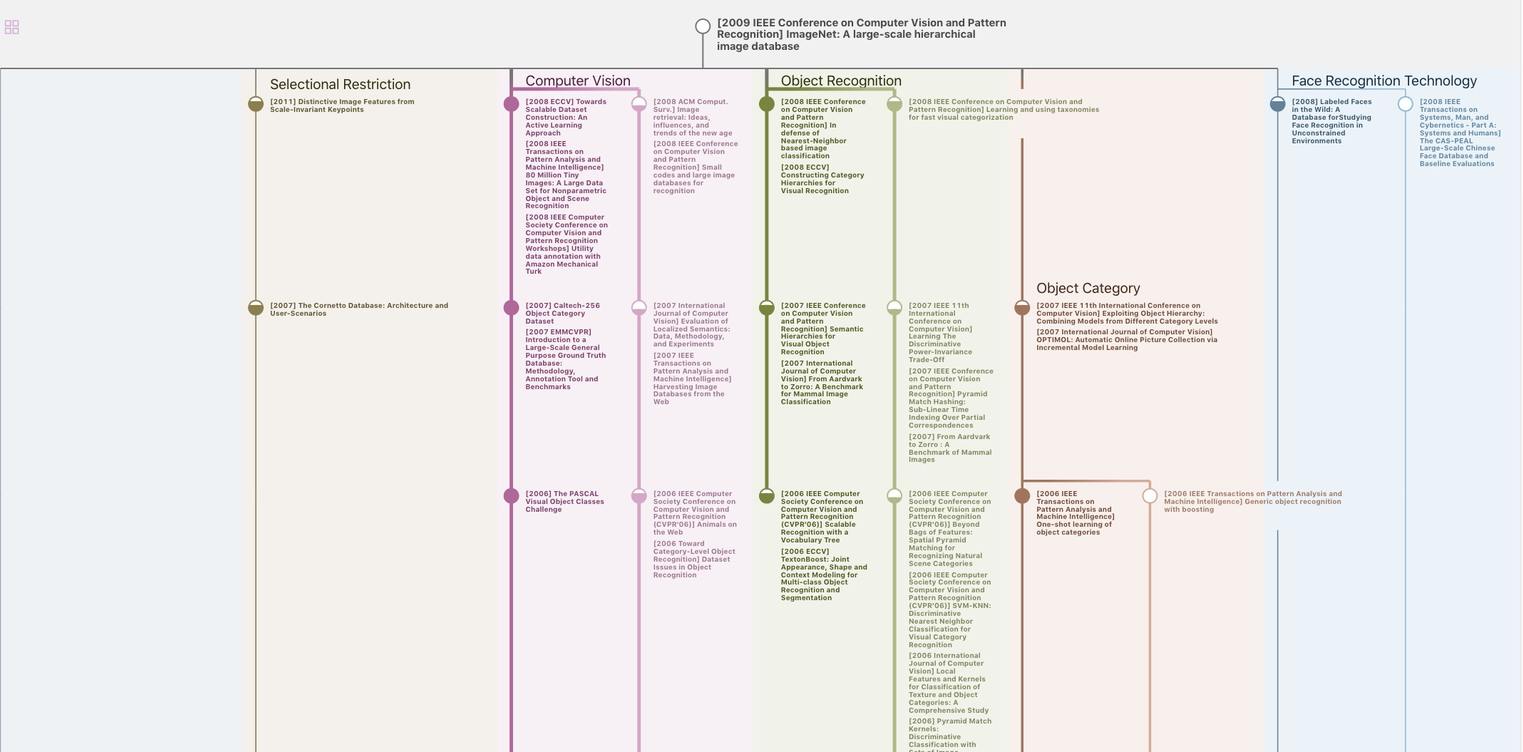
生成溯源树,研究论文发展脉络
Chat Paper
正在生成论文摘要