Improving single-stage activity recognition of excavators using knowledge distillation of temporal gradient data
COMPUTER-AIDED CIVIL AND INFRASTRUCTURE ENGINEERING(2024)
摘要
Single-stage activity recognition methods have been gaining popularity within the construction domain. However, their low per-frame accuracy necessitates additional post-processing to link the per-frame detections. Therefore, limiting their real-time monitoring capabilities is an indispensable component of the emerging construction of digital twins. This study proposes knowledge DIstillation of temporal Gradient data for construction Entity activity Recognition (DIGER), built upon the you only watch once (YOWO) method and improving its activity recognition and localization performance. Activity recognition is improved by designing an auxiliary backbone to exploit the complementary information in the temporal gradient data (transferred into YOWO using knowledge distillation), while localization is improved primarily through integration of complete intersection over union loss. DIGER achieved a per-frame activity recognition accuracy of 93.6% and localization mean average precision at 50% of 79.8% on a large custom dataset, outperforming state-of-the-art methods without requiring additional computation during inference, making it highly effective for real-time monitoring of construction site activities.
更多查看译文
AI 理解论文
溯源树
样例
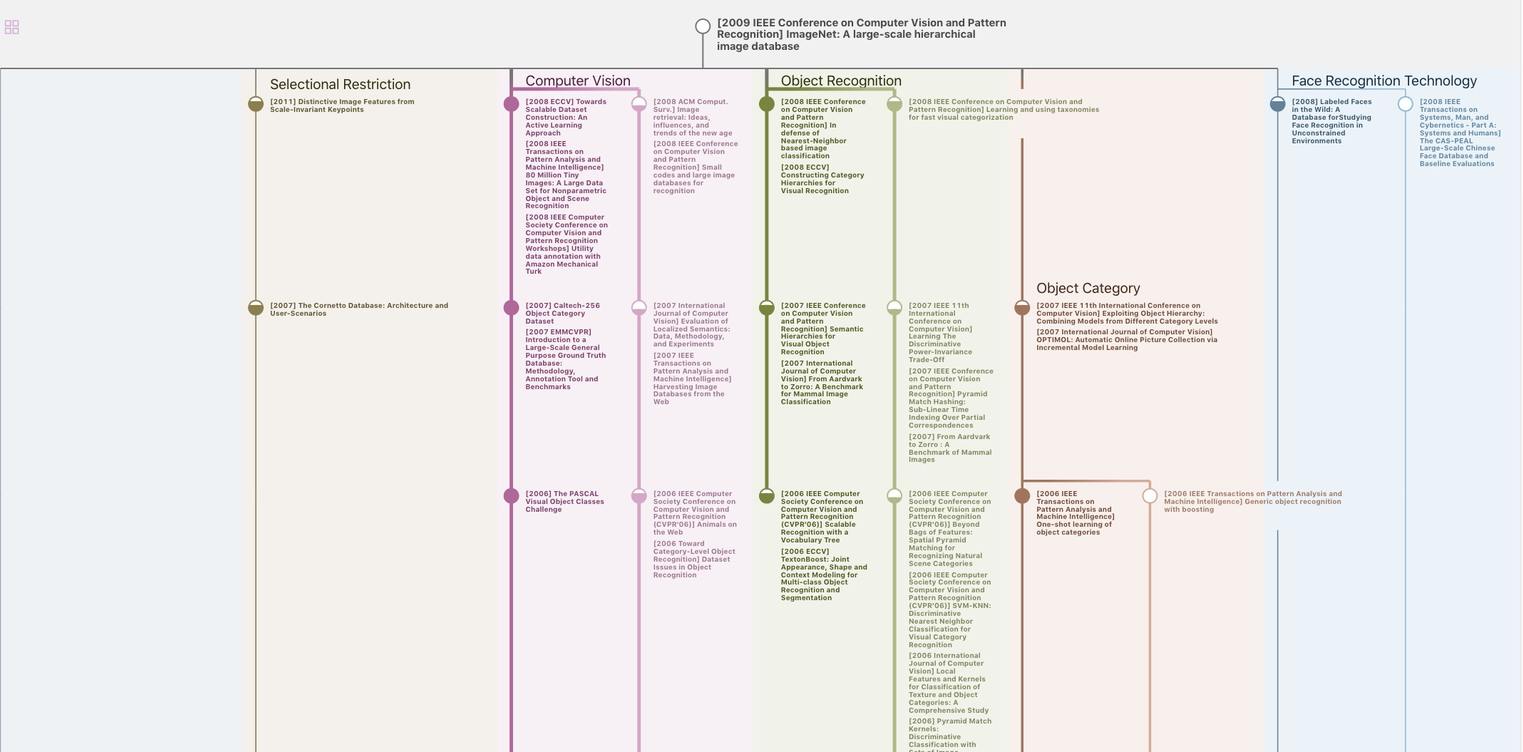
生成溯源树,研究论文发展脉络
Chat Paper
正在生成论文摘要