X-PEFT: eXtremely Parameter-Efficient Fine-Tuning for Extreme Multi-Profile Scenarios
CoRR(2024)
摘要
Parameter-efficient fine-tuning (PEFT) techniques, such as adapter tuning,
aim to fine-tune a pre-trained language model (PLM) using a minimal number of
parameters for a specific task or profile. Although adapter tuning provides
increased parameter efficiency compared to full-model fine-tuning, it
introduces a small set of additional parameters attached to a PLM for each
profile. This can become problematic in practical applications with multiple
profiles, particularly when a significant increase in the number of profiles
linearly boosts the total number of additional parameters. To mitigate this
issue, we introduce X-PEFT, a novel PEFT method that leverages a multitude of
given adapters by fine-tuning an extremely small set of compact tensors for a
new profile, which serve as binary masks to adaptively select the given
adapters. To efficiently validate our proposed method, we implement it using a
large number of trained or untrained (random) adapters. We evaluate the
performance of X-PEFT through LaMP and GLUE tasks and demonstrate that it
either matches or surpasses the effectiveness of conventional adapter tuning,
despite reducing the memory requirements per profile by a factor of 10,000
compared to it.
更多查看译文
AI 理解论文
溯源树
样例
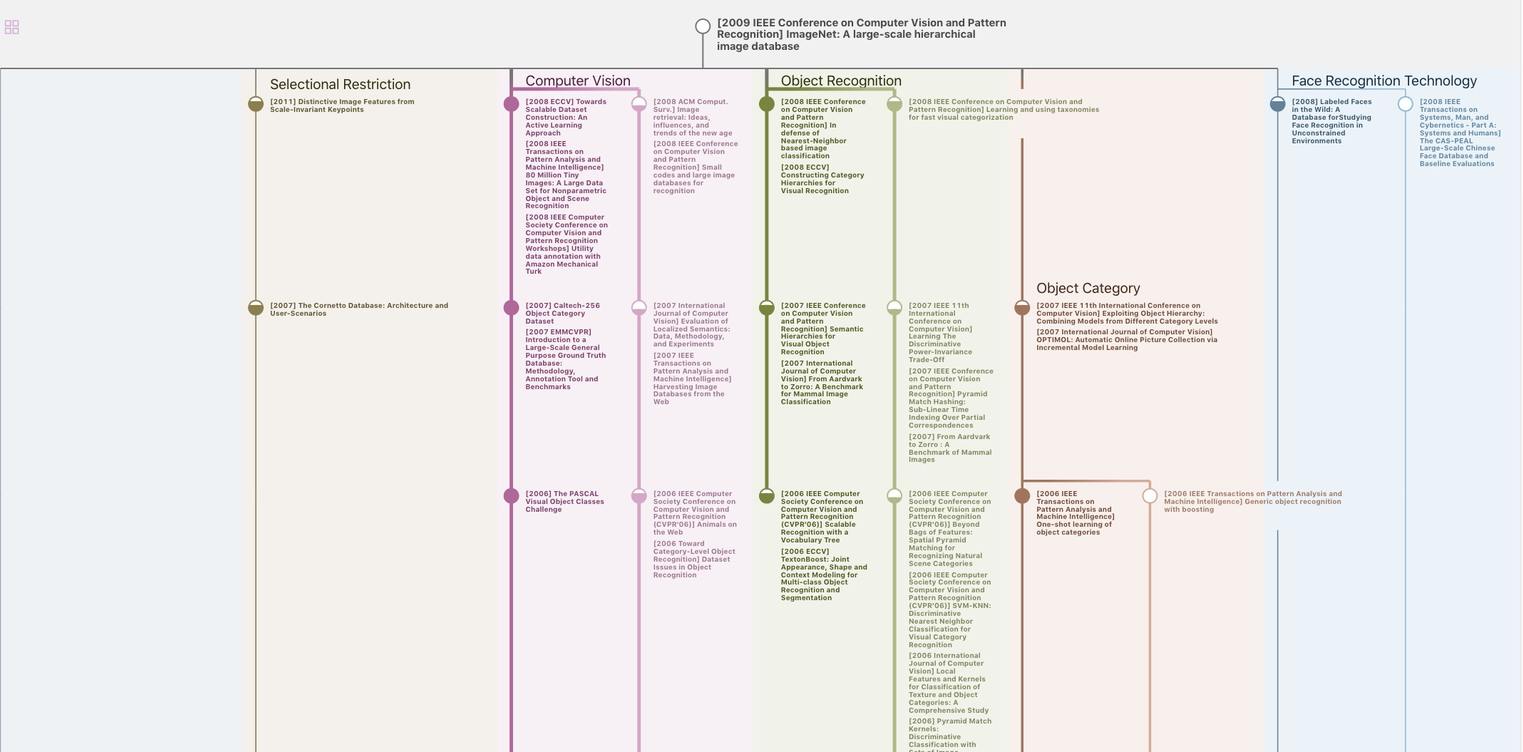
生成溯源树,研究论文发展脉络
Chat Paper
正在生成论文摘要